Adversarial Debiasing techniques towards ‘fair’ skin lesion classification
2023 11th International IEEE/EMBS Conference on Neural Engineering (NER)(2023)
摘要
Early detection of skin cancer lesions impacts overall patient survival. However, as shown in the literature, people of color have worse prognoses and lower survival rates than people with lighter skin tones. Often, this is the result of delayed or incorrect diagnoses for people of color. Deep learning could provide an effective screening technology with readily available image-capturing techniques, even from a mobile phone. However, often skin complexion biases limit the accuracy of the deep learning models as the applications are mainly trained on data that is predominantly light skin. We propose an adversarial debiasing method with partial learning that produces fairer outcomes for both lighter and darker skin colors. The model unlearns the skin color bias by using an additional classifier to penalize the learning of features specific to skin color. In the partial learning, we added Testing with Concept Activation Vector(TCAV) to select the particular layer where the skin color features are most discernable. We evaluated the performance internally on Fizpatrick17k and externally on ISIC datasets. The debiased model performed equally well for identifying malignant cases on both light and darker skin color. In conclusion, our work finds the adversarial debiasing techniques able to increase the skin lesion model's performance for all the skin color variations without the need for a balanced training dataset and provide a generalization to the external datasets.
更多查看译文
关键词
Deep Learning, Fairness, Melanoma, Adversarial Training
AI 理解论文
溯源树
样例
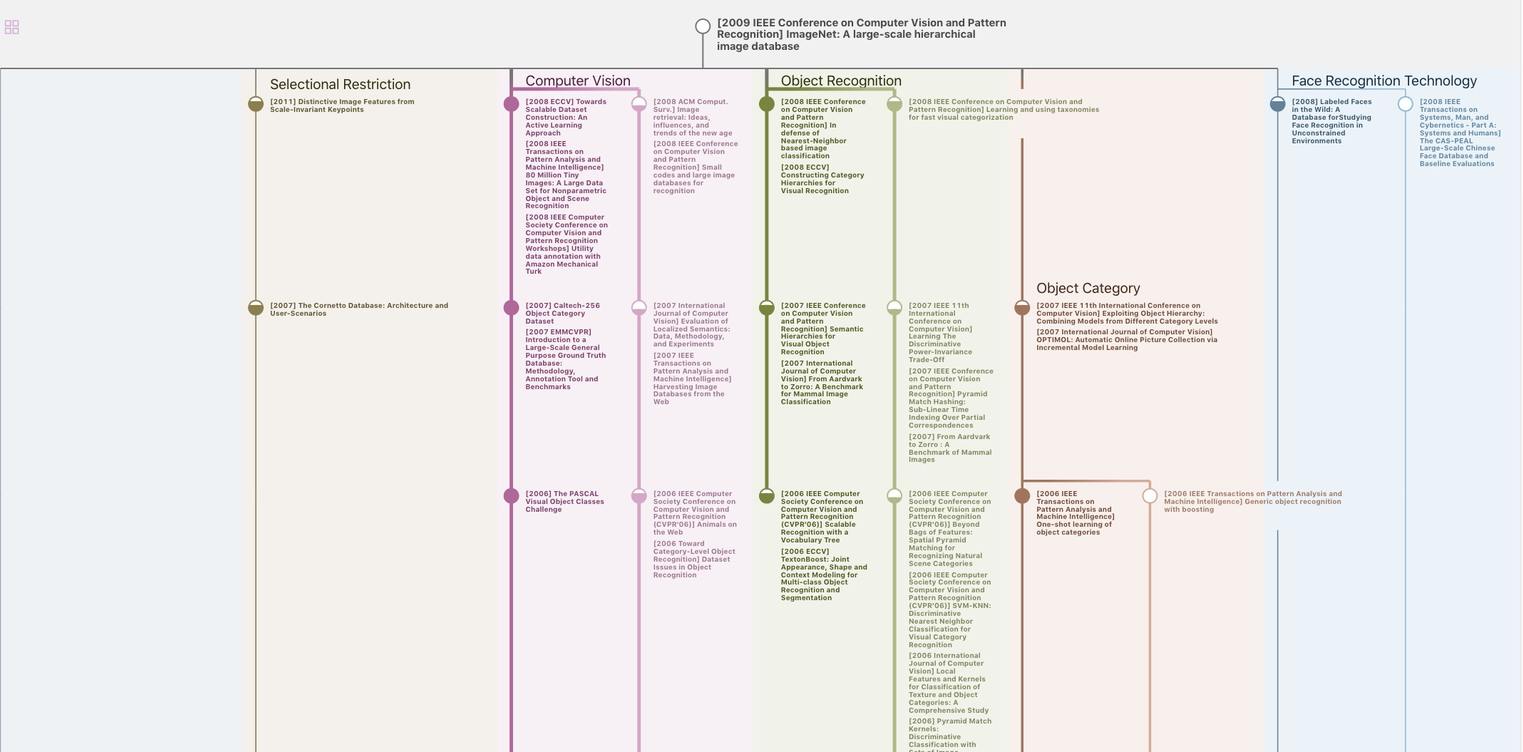
生成溯源树,研究论文发展脉络
Chat Paper
正在生成论文摘要