An Efficient Hourglass Transformer with Modality-guided Dynamic Token Merge for Document Understanding
IJCAI 2023(2023)
摘要
Transformers achieve promising performance in the structure document understanding because of its complex calculation, but remain inefficient in time complexity. Existing lightweight transformers fail to represent different granularity in documents. Therefore, it is difficult for them to achieve a good trade-off between efficiency and performance. In this paper, we present an hourglass architecture for high-performance low-computation document understanding. Specifically, we design a modality-guided dynamic token merging block, which not only makes the model learn multi-granularity representation, but also reduces the number of tokens in the middle layer. Considering that multi-modal interaction is critical for guiding merge, we develop a symmetry cross attention (SCA) to efficiently interact with multi-modal information. SCA allows one modality input as query to calculate cross attention with another modality. Extensive experiments on FUNSD, SROIE, and CORD datasets demonstrate that our model achieves state-of-the-art performance and 1.9x faster inference time than the state-of-the-art methods.
更多查看译文
AI 理解论文
溯源树
样例
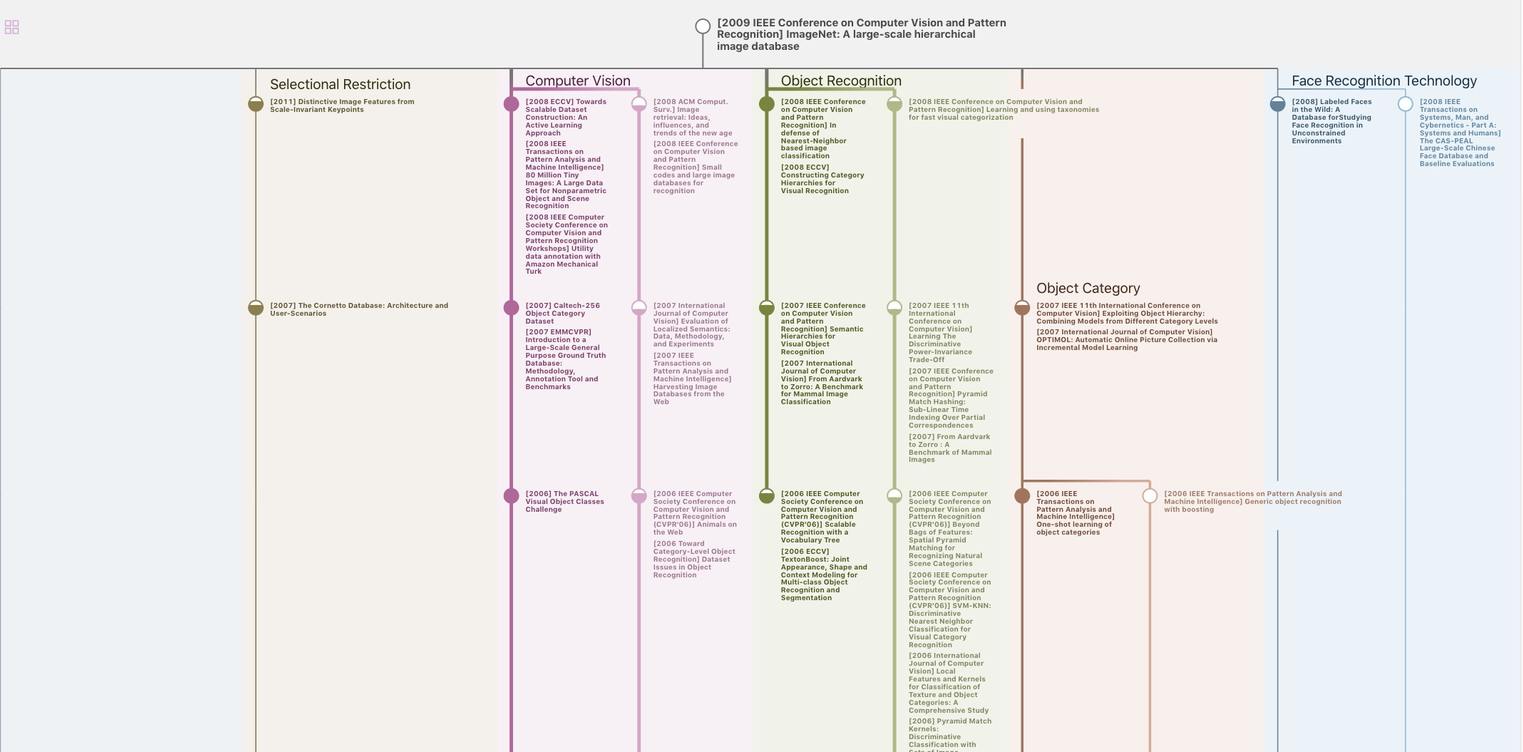
生成溯源树,研究论文发展脉络
Chat Paper
正在生成论文摘要