DenseDINO: boosting dense self-supervised learning with token-based point-level consistency
IJCAI 2023(2023)
摘要
In this paper, we propose a simple yet effective transformer framework for self-supervised learning called DenseDINO to learn dense visual representations. To exploit the spatial information that the dense prediction tasks require but neglected by the existing self-supervised transformers, we introduce point-level supervision across views in a novel token-based way. Specifically, DenseDINO introduces some extra input tokens called reference tokens to match the point-level features with the position prior. With the reference token, the model could maintain spatial consistency and deal with multi-object complex scene images, thus generalizing better on dense prediction tasks. Compared with the vanilla DINO, our approach obtains competitive performance when evaluated on classification in ImageNet and achieves a large margin (+7.2% mIoU) improvement in semantic segmentation on PascalVOC under the linear probing protocol for segmentation.
更多查看译文
关键词
learning,consistency,self-supervised,token-based,point-level
AI 理解论文
溯源树
样例
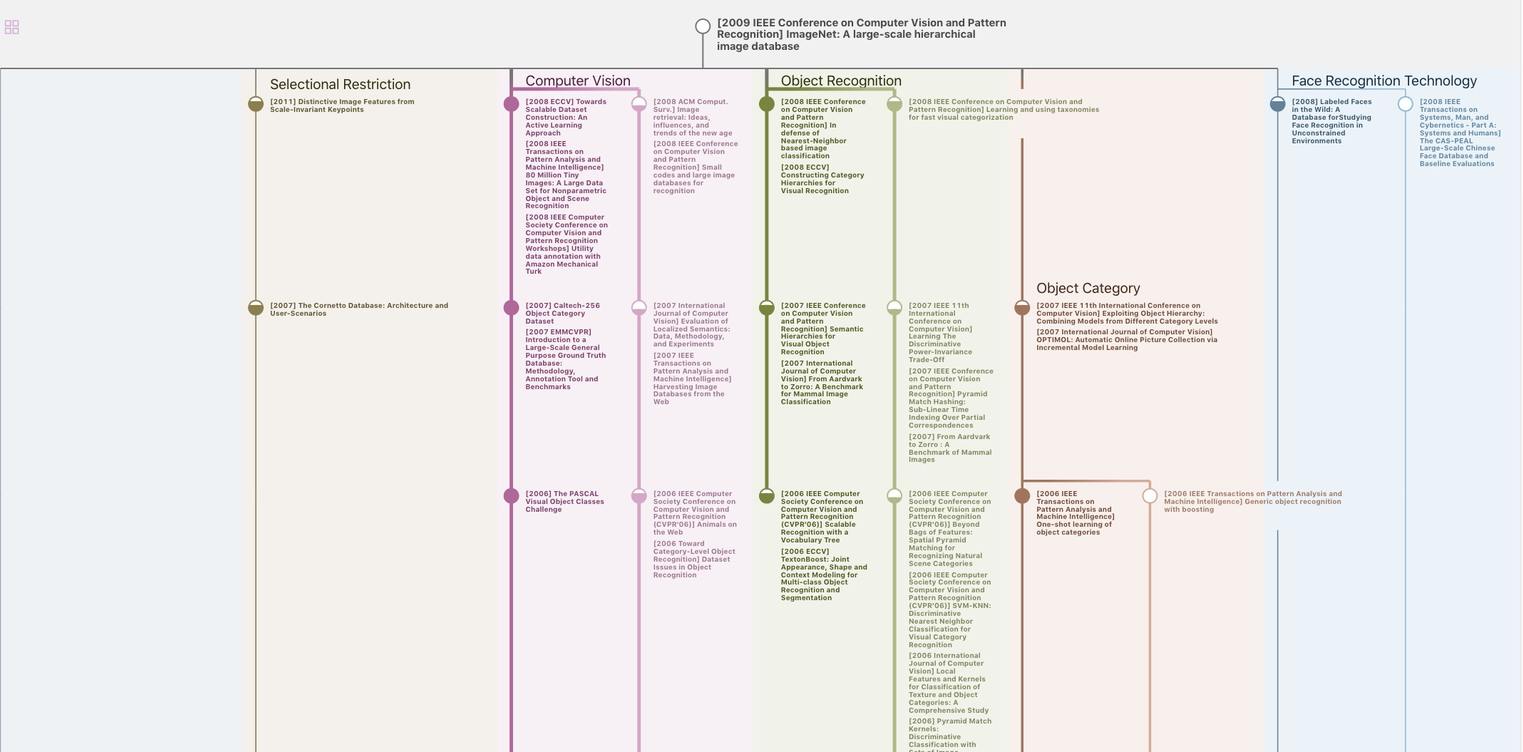
生成溯源树,研究论文发展脉络
Chat Paper
正在生成论文摘要