Controlling neural style transfer with deep reinforcement learning
IJCAI 2023(2023)
摘要
Controlling the degree of stylization in the Neural Style Transfer (NST) is a little tricky since it usually needs hand-engineering on hyper-parameters. In this paper, we propose the first deep Reinforcement Learning (RL) based architecture that splits one-step style transfer into a step-wise process for the NST task. Our RL-based method tends to preserve more details and structures of the content image in early steps, and synthesize more style patterns in later steps. It is a user-easily-controlled style-transfer method. Additionally, as our RL-based model performs the stylization progressively, it is lightweight and has lower computational complexity than existing one-step Deep Learning (DL) based models. Experimental results demonstrate the effectiveness and robustness of our method.
更多查看译文
关键词
neural style transfer,deep reinforcement learning,controlling
AI 理解论文
溯源树
样例
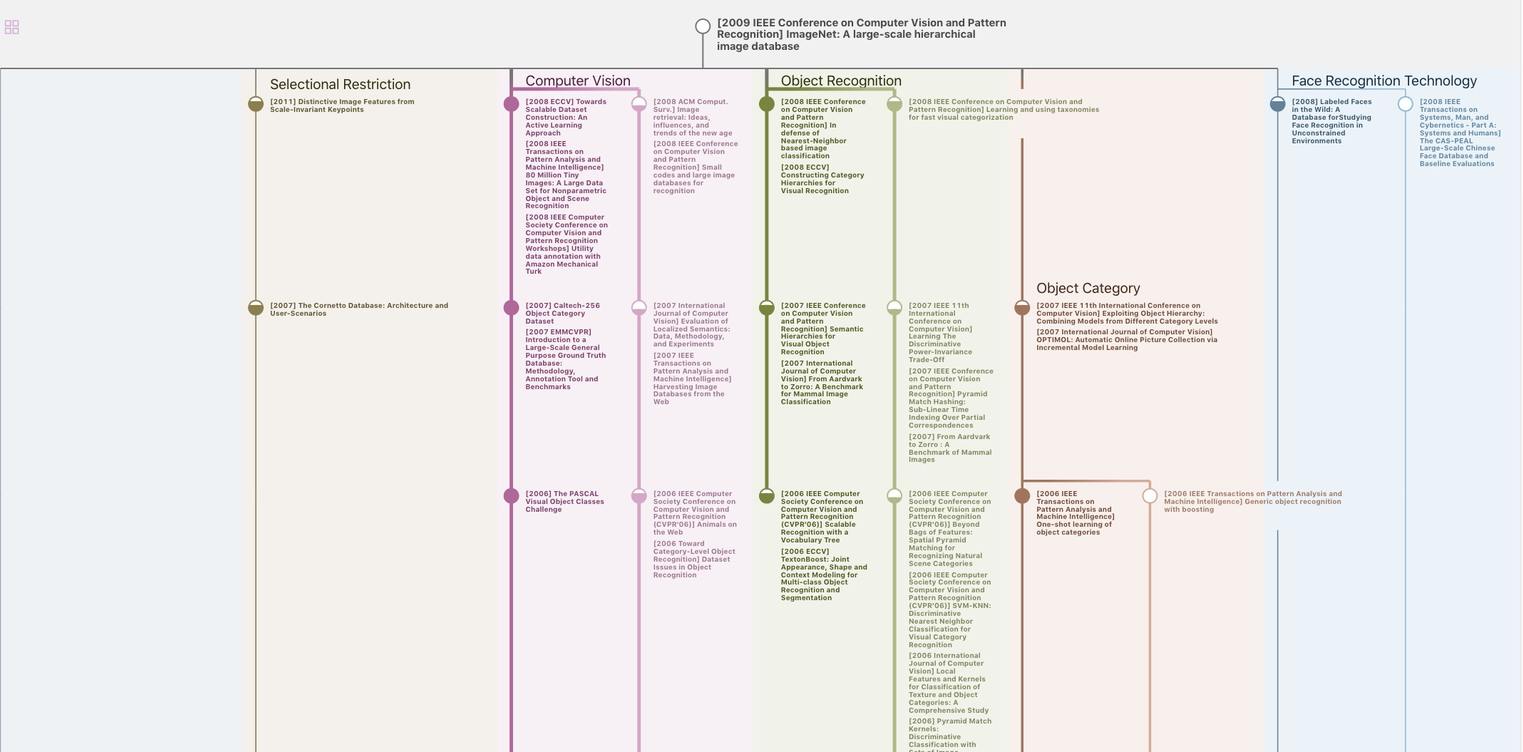
生成溯源树,研究论文发展脉络
Chat Paper
正在生成论文摘要