Unsupervised Multi-channel Separation and Adaptation
ICASSP 2024 - 2024 IEEE International Conference on Acoustics, Speech and Signal Processing (ICASSP)(2023)
摘要
A key challenge in machine learning is to generalize from training data to an application domain of interest. This work generalizes the recently-proposed mixture invariant training (MixIT) algorithm to perform unsupervised learning in the multi-channel setting. We use MixIT to train a model on far-field microphone array recordings of overlapping reverberant and noisy speech from the AMI Corpus. The models are trained on both supervised and unsupervised training data, and are tested on real AMI recordings containing overlapping speech. To objectively evaluate our models, we also use a synthetic multi-channel AMI test set. Holding network architectures constant, we find that a fine-tuned semi-supervised model yields the largest improvement to SI-SNR and to human listening ratings across synthetic and real datasets, outperforming supervised models trained on well-matched synthetic data. Our results demonstrate that unsupervised learning through MixIT enables model adaptation on both single- and multi-channel real-world speech recordings.
更多查看译文
关键词
multi-channel,speech separation
AI 理解论文
溯源树
样例
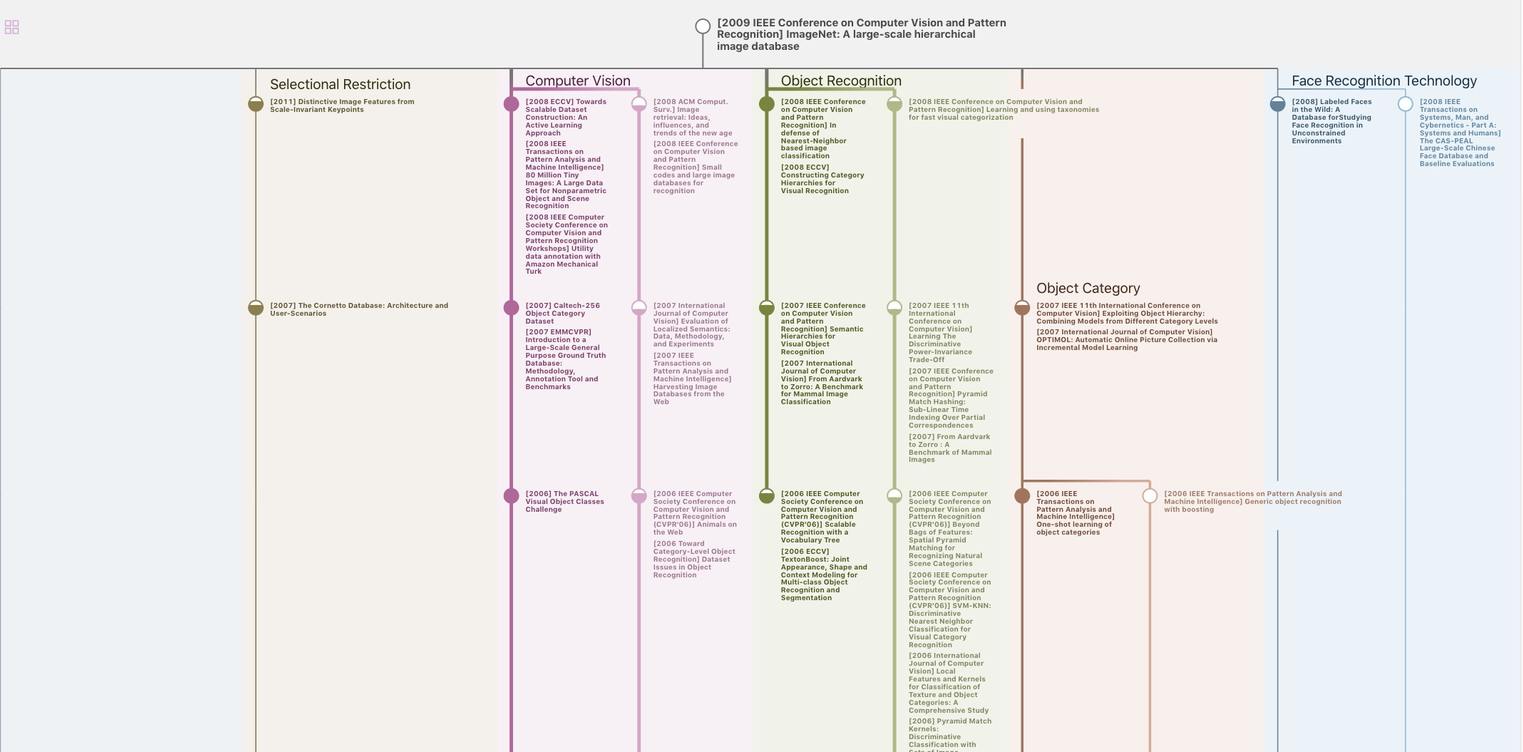
生成溯源树,研究论文发展脉络
Chat Paper
正在生成论文摘要