SPENSER: Towards a NeuroEvolutionary Approach for Convolutional Spiking Neural Networks
PROCEEDINGS OF THE 2023 GENETIC AND EVOLUTIONARY COMPUTATION CONFERENCE COMPANION, GECCO 2023 COMPANION(2023)
摘要
Spiking Neural Networks (SNNs) have attracted recent interest due to their energy efficiency and biological plausibility. However, the performance of SNNs still lags behind traditional Artificial Neural Networks (ANNs), as there is no consensus on the best learning algorithm for SNNs. Best-performing SNNs are based on ANN to SNN conversion or learning with spike-based backpropagation through surrogate gradients. The focus of recent research has been on developing and testing different learning strategies, with hand-tailored architectures and parameter tuning. Neuroevolution (NE), has proven successful as a way to automatically design ANNs and tune parameters, but its applications to SNNs are still at an early stage. DENSER is a NE framework for the automatic design and parametrization of ANNs, based on the principles of Genetic Algorithms (GA) and Structured Grammatical Evolution (SGE). In this paper, we propose SPENSER, a NE framework for SNN generation based on DENSER, for image classification on the MNIST and Fashion-MNIST datasets. SPENSER generates competitive performing networks with a test accuracy of 99.42% and 91.65% respectively.
更多查看译文
关键词
spiking neural networks,neuroevolution,DENSER,computer vision
AI 理解论文
溯源树
样例
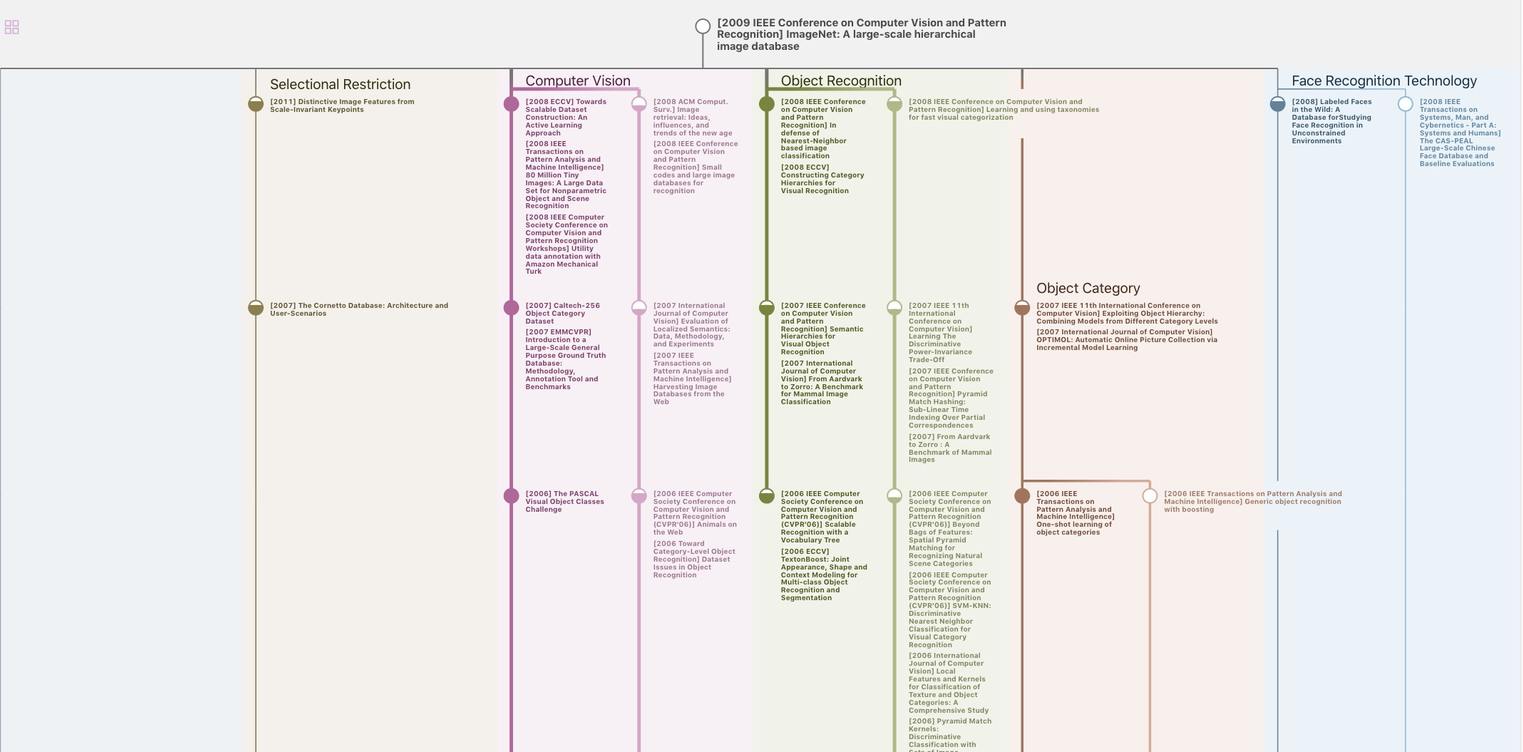
生成溯源树,研究论文发展脉络
Chat Paper
正在生成论文摘要