Sampling, Diffusions, and Stochastic Localization
CoRR(2023)
摘要
Diffusions are a successful technique to sample from high-dimensional distributions can be either explicitly given or learnt from a collection of samples. They implement a diffusion process whose endpoint is a sample from the target distribution and whose drift is typically represented as a neural network. Stochastic localization is a successful technique to prove mixing of Markov Chains and other functional inequalities in high dimension. An algorithmic version of stochastic localization was introduced in [EAMS2022], to obtain an algorithm that samples from certain statistical mechanics models. This notes have three objectives: (i) Generalize the construction [EAMS2022] to other stochastic localization processes; (ii) Clarify the connection between diffusions and stochastic localization. In particular we show that standard denoising diffusions are stochastic localizations but other examples that are naturally suggested by the proposed viewpoint; (iii) Describe some insights that follow from this viewpoint.
更多查看译文
关键词
stochastic localization,diffusions,sampling
AI 理解论文
溯源树
样例
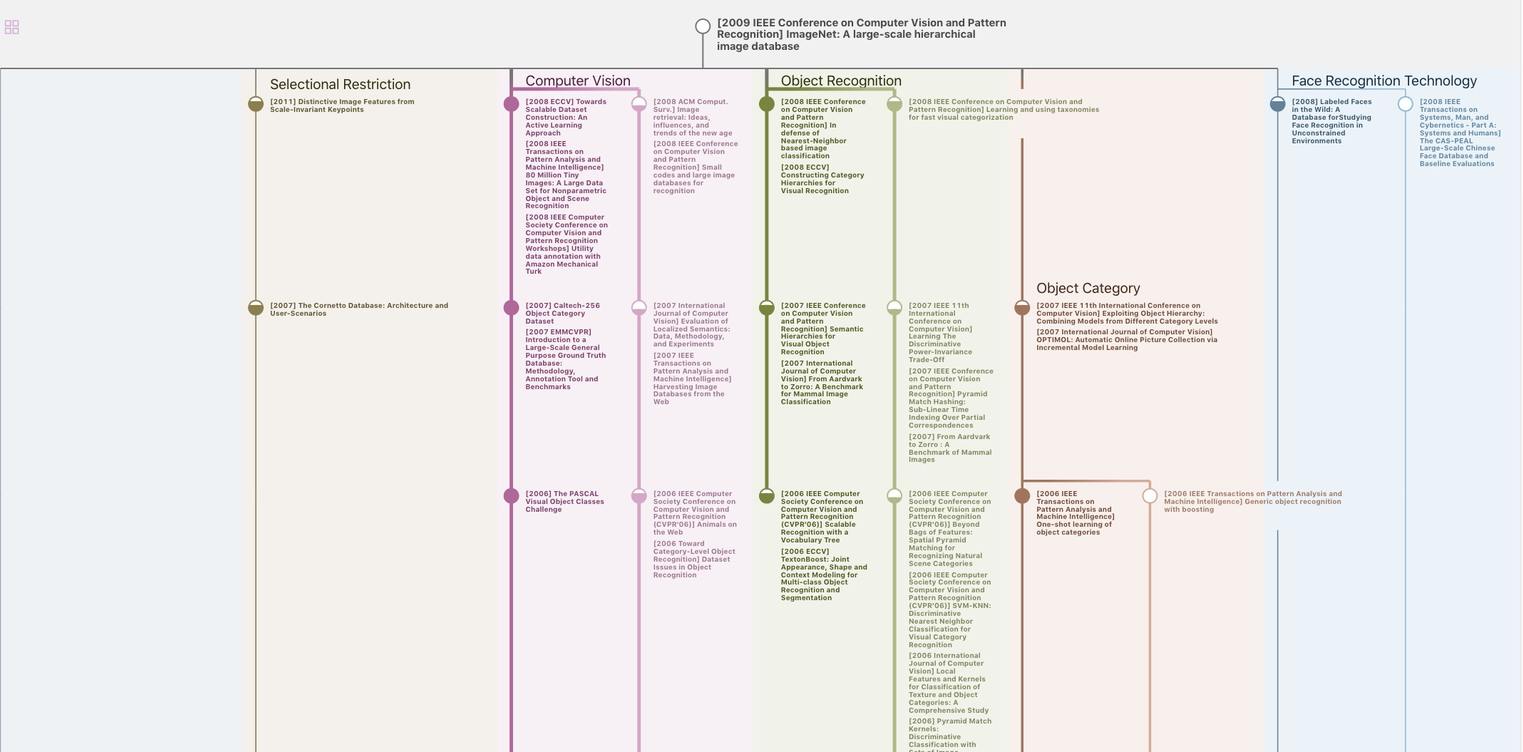
生成溯源树,研究论文发展脉络
Chat Paper
正在生成论文摘要