Location-Aware Heterogeneous Graph Neural Network for Region Recommendation
Communications, Signal Processing, and Systems(2023)
摘要
With the diversification of human activity and travel demand in urban space, recommending ROIs (region-of-interest) to users is important for both satisfying commercial demands and better understanding user urban lifestyles. Current researches mainly resort to the traditional POI-level (point-of-interest) or neural network-based recommendation methods for ROI recommendation, in disregard of the rich heterogeneous graph information, such as user-region-user, region-category-region, just to name a few. In this work, we employ the heterogeneous graph to address this issue, considering heterogeneous graph contains more comprehensive information and rich semantics. We propose a novel meta-path based graph attention network for ROI recommendation, called MRec. MRec is a newly devised heterogeneous graph neural network, which is equipped with both node-level and semantic-level attentions. Specially, the node-level attention aims to learn the importance between a node and its meta-path based neighbors, while the semantic-level attention is to learn the importance of different meta-paths. This mechanism contributes to effectively embedding users and ROIs in a hierarchical manner of fully considering both node and semantic-level component information. An extensive experiment on two real-world datasets demonstrates the effectiveness of the proposed framework.
更多查看译文
关键词
ROI recommendation, Graph neural network, Heterogeneous information network, Attention network
AI 理解论文
溯源树
样例
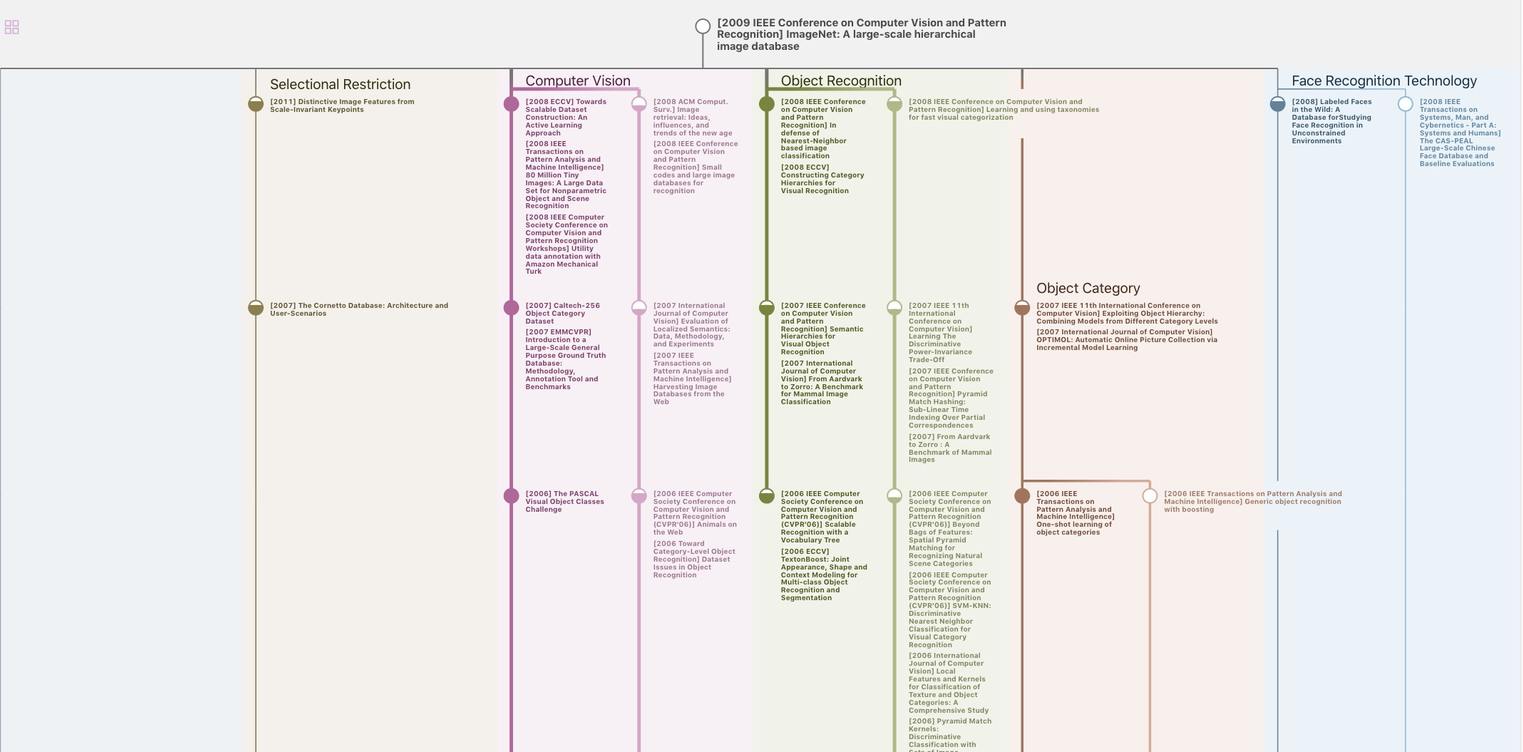
生成溯源树,研究论文发展脉络
Chat Paper
正在生成论文摘要