A Novel Deep Learning Framework for Interpretable Drug-Target Interaction Prediction with Attention and Multi-task Mechanism
Database Systems for Advanced Applications(2023)
摘要
The measurement of drug-target interaction(DTI) is a major task in the field of drug discovery, where drugs are typically small molecules and targets are typically proteins. Traditional DTI measurements in the lab are time consuming and expensive. DTI can be predicted through the use of computational methods like ligand similarity comparison and molecular docking simulation. However, these methods strongly rely on domain expertise. Deep learning has recently advanced, and some deep learning techniques are being used to predict DTI. These deep learning ways can extract drug and target features automatically without domain knowledge and produce good results. In this work, we propose an end-to-end deep learning framework to predict DTI. The unsupervised method Mol2Vec with self-attention is used to extract the drug features. To extract the target features, we pre-train a BERT model, which is the state-of-the-art model for many text comprehension tasks in NLP. In order to improve the generalization ability of the model, we introduce a multi-task learning mechanism by using two transformer encoder-decoders. As far as we know, we are the first to apply Mol2Vec, BERT, attention mechanism and multi-task mechanism to one model. The experiment results show that our model outperforms other latest deep learning methods. Finally, we interpret our model through a case study by visualizing the predicted binding sites.
更多查看译文
关键词
attention,deep learning,drug-target,multi-task
AI 理解论文
溯源树
样例
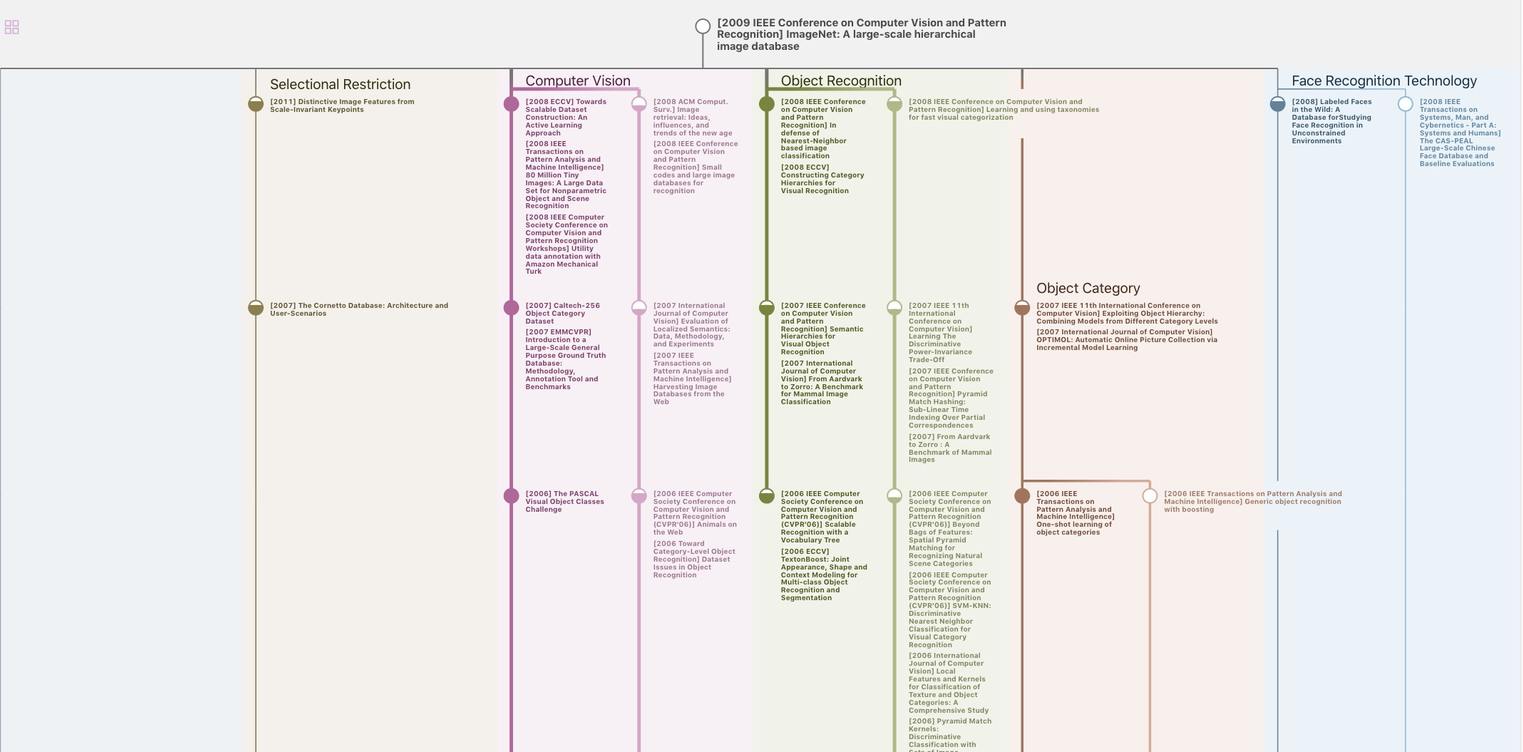
生成溯源树,研究论文发展脉络
Chat Paper
正在生成论文摘要