Classification and Segmentation on Multi-regional Brain Tumors Using Volumetric Images of MRI with Customized 3D U-Net Framework
Proceedings of International Conference on Information and Communication Technology for Development(2023)
摘要
Automated segmentation is a computerized technique that helps to find tumor location, size, and shape. Human segmentation is error prone, time consuming, and needs an expert radiologist. In our study, we developed a customized 3D U-Net model that processes 3D volumetric images for multiclass tumor segmentation. This framework is modified in such a way that the gradient flow is better for finding accurate output. The BraTS 2020 dataset is used to train this network with end-to-end learning strategy followed by defining the proper skip connection from encoder to decoder. In model evaluation, binary cross-entropy with Dice loss functions is utilized. Testing samples are predicted and classified into three regions: whole tumor (WT), tumor core (TC), and enhancing tumor (ET). Model performance is evaluated through Dice and Jaccard coefficient metrics for each class. Furthermore, in the testing dataset, the Dice scores are found at 0.753, 0.799, and 0.887 for the ET, TC, and WT classes.
更多查看译文
关键词
Deep learning, Medical imaging, MRI, Semantic segmentation, 3D U-Net, BraTS 2020
AI 理解论文
溯源树
样例
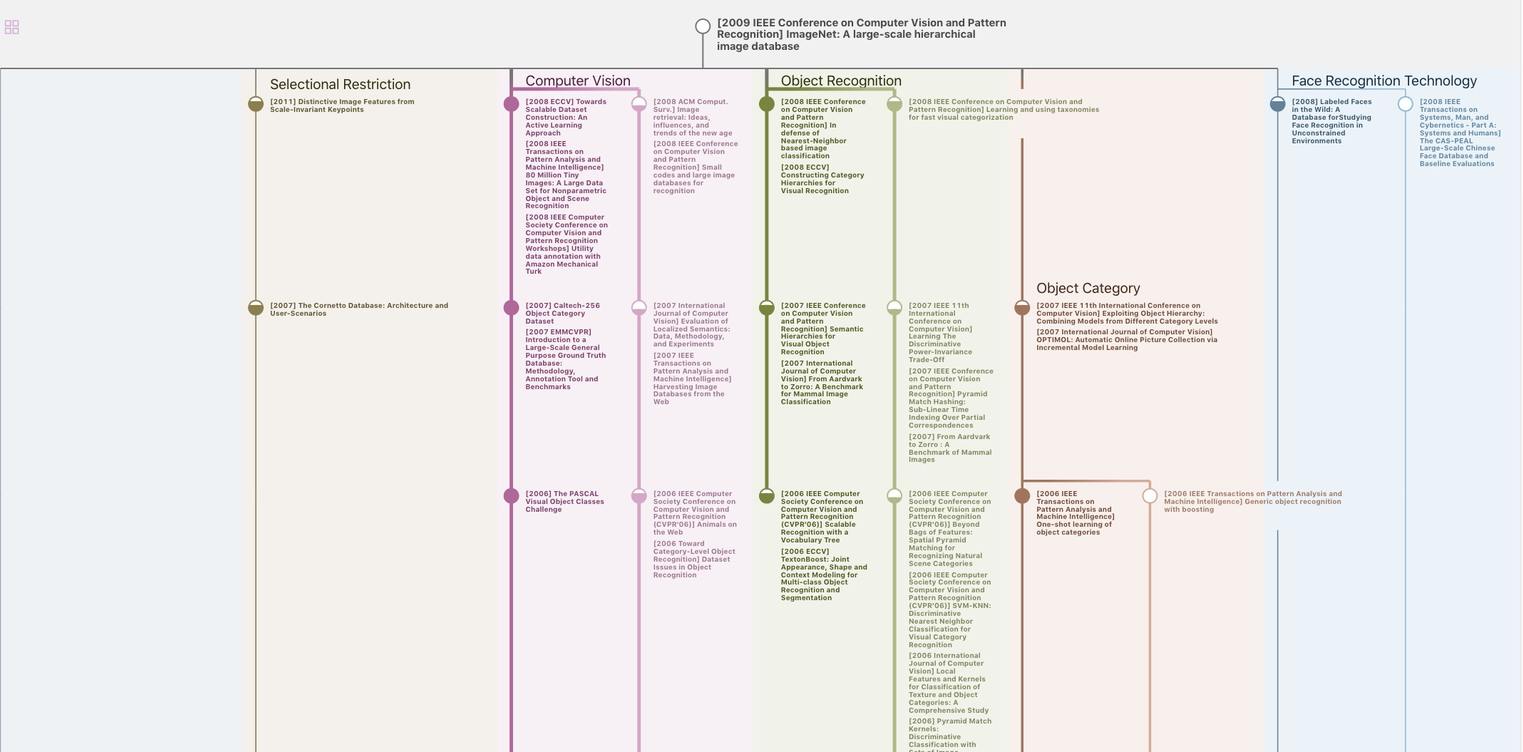
生成溯源树,研究论文发展脉络
Chat Paper
正在生成论文摘要