TieComm: Learning a Hierarchical Communication Topology Based on Tie Theory
Database Systems for Advanced Applications(2023)
摘要
Communication plays an important role in Internet of Things that assists cooperation between devices for better resource management. This work considers the problem of learning cooperative policies using communications in Multi-Agent Reinforcement Learning (MARL), which plays an important role to stabilize agent training and improve the policy learned by enabling the agent to capture more information in partially observable environments. Existing studies either adopt a prior topology by experts or learn a communication topology through a costly process. In this work, we optimize the communication mechanism by exploiting both local agent communications and distant agent communications. Our solution is motivated by tie theory in social networks, where strong ties (close friends) communicate differently with weak ties (distant friends). The proposed novel multi-agent reinforcement learning framework named TieComm, learns a dynamic communication topology consisting of inter- and intra-group communication for efficient policy learning. We factorize the joint multi-agent policy into a centralized tie reasoning policy and decentralized conditional action policies of agents, based on which we propose an alternative updating schema to achieve efficient optimization. Experimental results on Level-Based Foraging and Blind-particle Spread demonstrate the effectiveness of our tie theory based RL framework.
更多查看译文
关键词
hierarchical communication topology,learning,theory
AI 理解论文
溯源树
样例
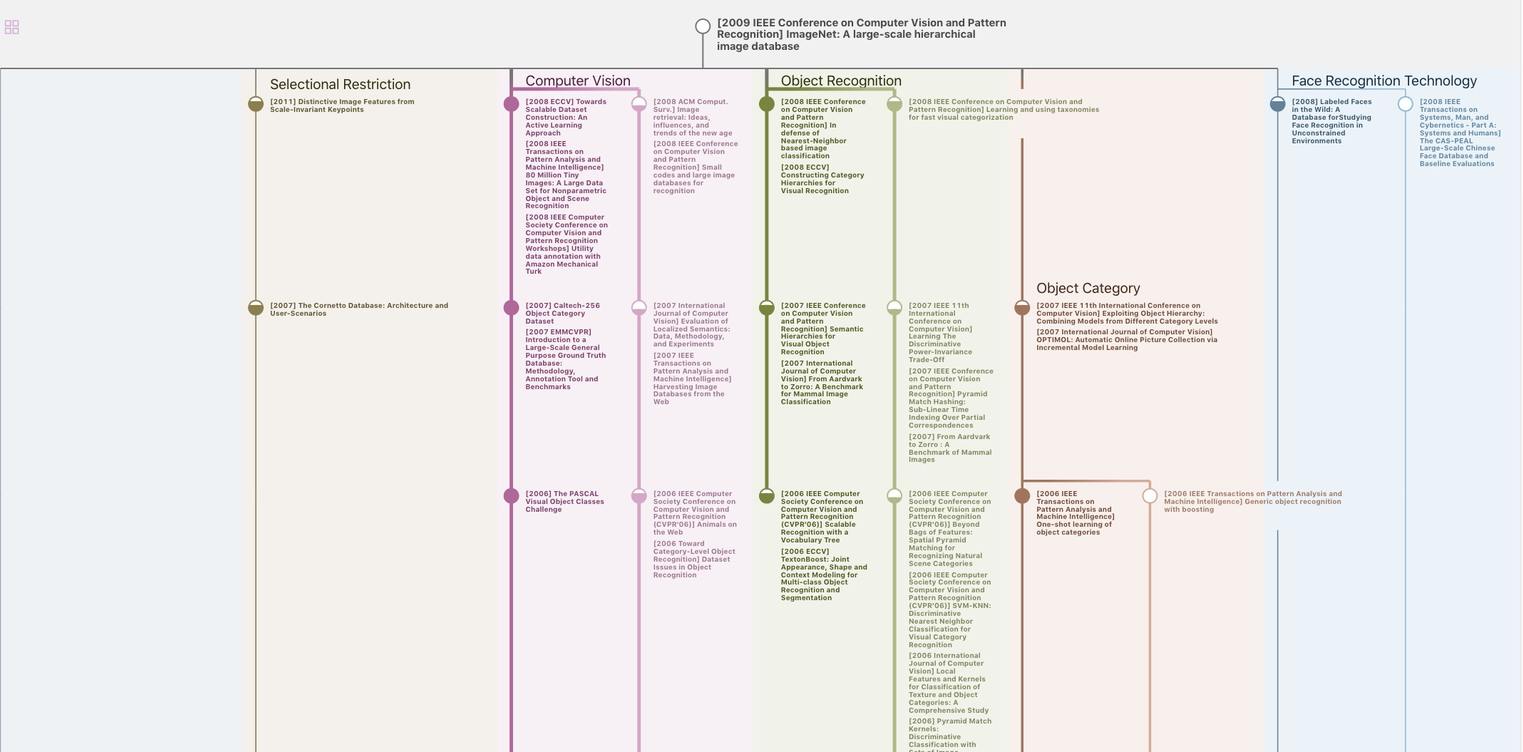
生成溯源树,研究论文发展脉络
Chat Paper
正在生成论文摘要