Gross Domestic Product Prediction in Various Countries with Classic Machine Learning Techniques
Nature of Computation and Communication(2023)
摘要
Gross Domestic Product (GDP) is an indicator used to measure the total market value of all final goods and services produced within a national territory during a given period. This is an essential indicator for formulating macroeconomic policies. This study presents a classical machine learning algorithm to forecast GDP in countries from 2013 to 2018 (with Economic Freedom Index’s Predicting GPD dataset). We use the Feature importance technique and incorporate other methods such as PCA and KBest; simultaneously, we tune the hyperparameters for the model to have more optimal results. We compare the predictive accuracy of Random Forest (RF) with other classical models such as Support Vector Machines (SVM). We find that RF KBest outperforms RF and SVM. The forecast accuracy is measured by
$$R^2$$
has reached 0.904 in predicting GDP in 186 countries. This study encourages increasing the use of machine learning models in macroeconomic forecasting. Besides, we present GDP growth rates (as a percentage) by region. We also analyze and find some critical factors that can significantly affect GDP, such as Freedom from Corruption, Property rights, and the unemployment rate.
更多查看译文
AI 理解论文
溯源树
样例
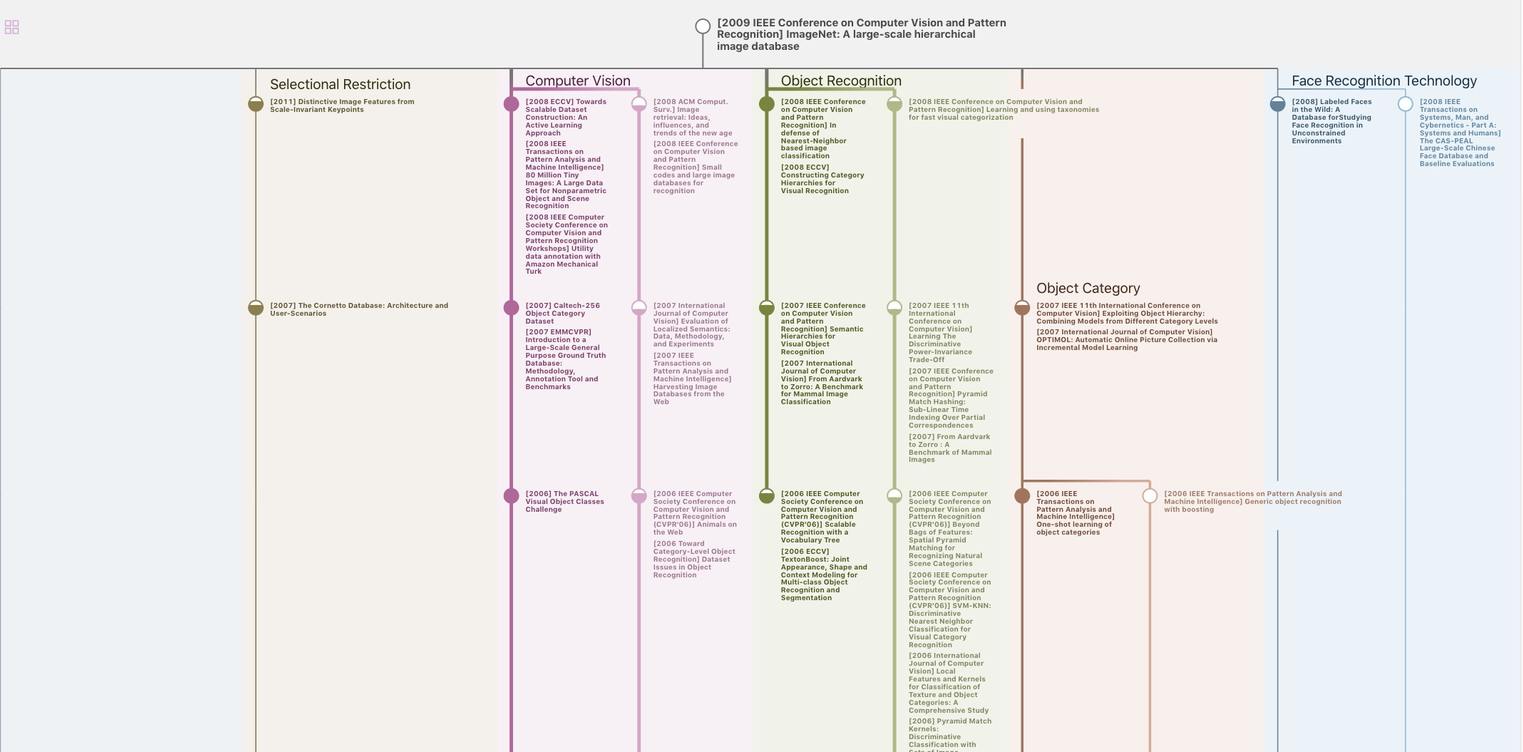
生成溯源树,研究论文发展脉络
Chat Paper
正在生成论文摘要