A Machine Learning Approach for Locating Businesses Along Main Arteries in Inner Cities
Resilient and Responsible Smart Cities(2023)
摘要
Throughout history, central squares and main pedestrian arteries of cities provided goods and services to meet the needs of their catchment areas. This study examines which types of businesses tend to agglomerate together and which ones are spread out. In addition, we analyzed whether there is a correlation between the location of the businesses and their clientele. Our research question is whether a Machine Learning (ML) system be trained to predict the best-performing locations for new commercial uses in the city—using city data showing openings/closures of various types of business during the last ten years. This study is based on four main phases: (1) Geoeconomic analysis by calculating ordered good index, (2) Clustering business categories by geolocation features, (3) Clustering business categories by the Wasserstein metric, and (4) Developing an interface for a ML predictor. The performance of the ML setup improves significantly when including additional features like demographic and socio-economic criteria in a supervised deep learning environment. This study suggests that our approach can help cities identify apt locations for a particular business type. We believe our approach can be applied to the disposition of public and government building programs in the future, which can dramatically help cities optimize their urban program layouts.
更多查看译文
关键词
Location allocation, Machine learning, Ordered good ındex, Wasserstein metric
AI 理解论文
溯源树
样例
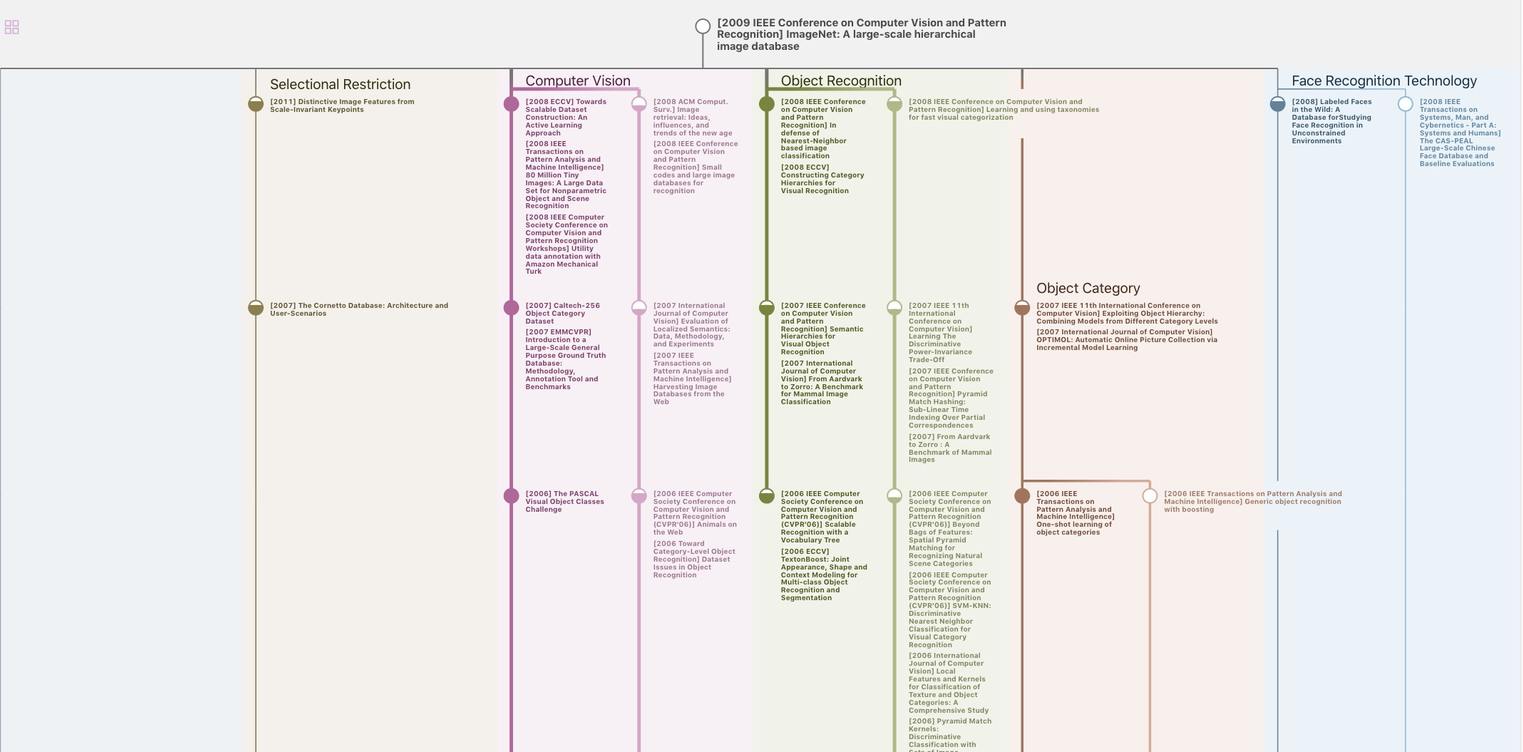
生成溯源树,研究论文发展脉络
Chat Paper
正在生成论文摘要