Hierarchical Encoder-Decoder with Addressable Memory Network for Diagnosis Prediction
Database Systems for Advanced Applications(2023)
摘要
Deep learning methods have demonstrated success in diagnosis prediction on Electronic Health Records (EHRs). Early attempts utilize sequential models to encode patient historical records, but they lack the ability to identify critical diseases for patient health conditions. Besides, some works focus on the hierarchical structure of EHR data during learning patient representations, but neglect it in diagnosis prediction process, which leads to insufficient utilization of hierarchical information. To tackle these challenges, we propose a new Hierarchical Encoder-Decoder with Addressable Memory Network for Diagnosis Prediction named HAMNet. Specifically, we employ a hierarchical encoder-decoder framework to utilize the hierarchical structure of historical visits both during representation learning and diagnosis prediction. Furthermore, we propose the addressable memory network to distinguish core diseases towards patient health status at next visit through the well-designed addressing mechanism. It employs feed-forward layers to build the memory network, which can automatically update the memory module through gradient backpropagation without explicit read/write operations. Finally, we evaluate the performance of HAMNet on a large real-world EHR dataset MIMIC-III, and it achieves the state-of-the-art performance compared with baselines.
更多查看译文
关键词
addressable memory network,diagnosis,prediction,encoder-decoder
AI 理解论文
溯源树
样例
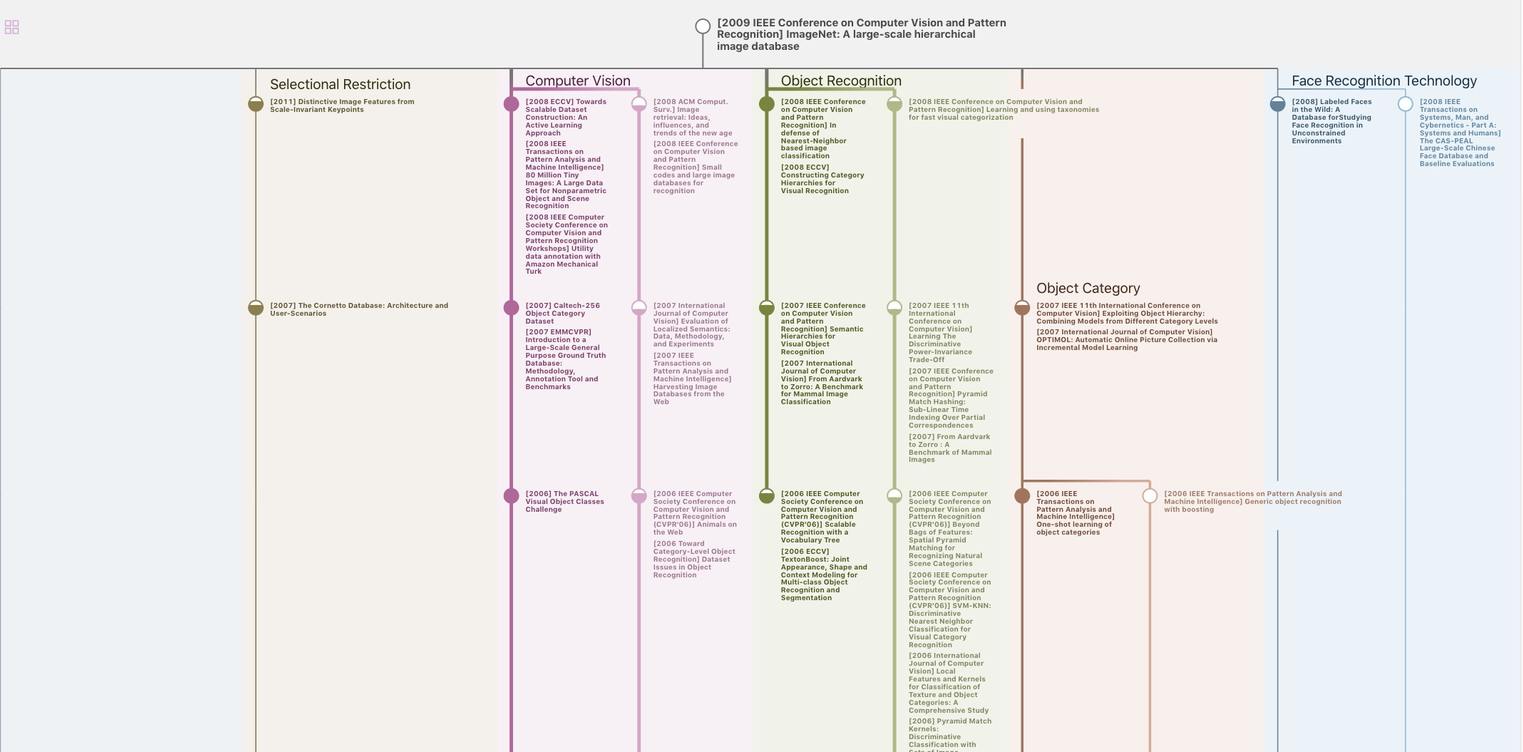
生成溯源树,研究论文发展脉络
Chat Paper
正在生成论文摘要