Microfluidic Dilution by Recycling Arbitrary Stock Solutions Using Various Mixing Models
Emerging Electronic Devices, Circuits and Systems(2023)
摘要
Microfluidic biochips are being widely used for automating biochemical laboratory protocols, and several algorithms for automated sample preparation (dilution and mixing of reagent fluids) were reported in the literature. Almost all the sample preparation algorithms assumed the availability of pure sample fluid (i.e., with 100% concentration) ignoring the fact that pure samples may not always be readily available in stock. In fact, in many practical situations, a number of arbitrary concentrations of the sample fluid are discarded as wastes, which can be recycled to reduce the sample preparation cost (usage of pure sample, etc.) and generate the desired target concentration of the fluid required elsewhere. The traditional microfluidic biochips support (1:1) mixing model, for which there exists only a few old algorithms in literature, (namely generalized dilution algorithm, i.e., GDA and dilution/mixing with reduced wastage, i.e., DMRW) which were solely proposed for solving dilution problem by recycling arbitrary stock solutions (RASS) with traditional biochips. Although, a variety of microfluidic biochips have been developed over the years, no sample preparation algorithm is proposed for solving RASS problem for such modern biochips—which may provide a cost-effective solution for RASS. In order to fill this gap, in this paper, we propose a “cost-effective” heuristic solution (called hRASS) for dilution of a sample fluid from its arbitrary stock solutions catering various mixing models supported by modern microfluidic biochips. Simulation results confirm the superiority of the proposed method and show that hRASS can improve the solution quality by 36.8% and 21% on average for a large number of random testcases over state-of-the-art methods (e.g., DMRW and GDA, respectively).
更多查看译文
关键词
Biochips, Microfluidics, Sample preparation, Dilution, Mixing models, Stock solutions
AI 理解论文
溯源树
样例
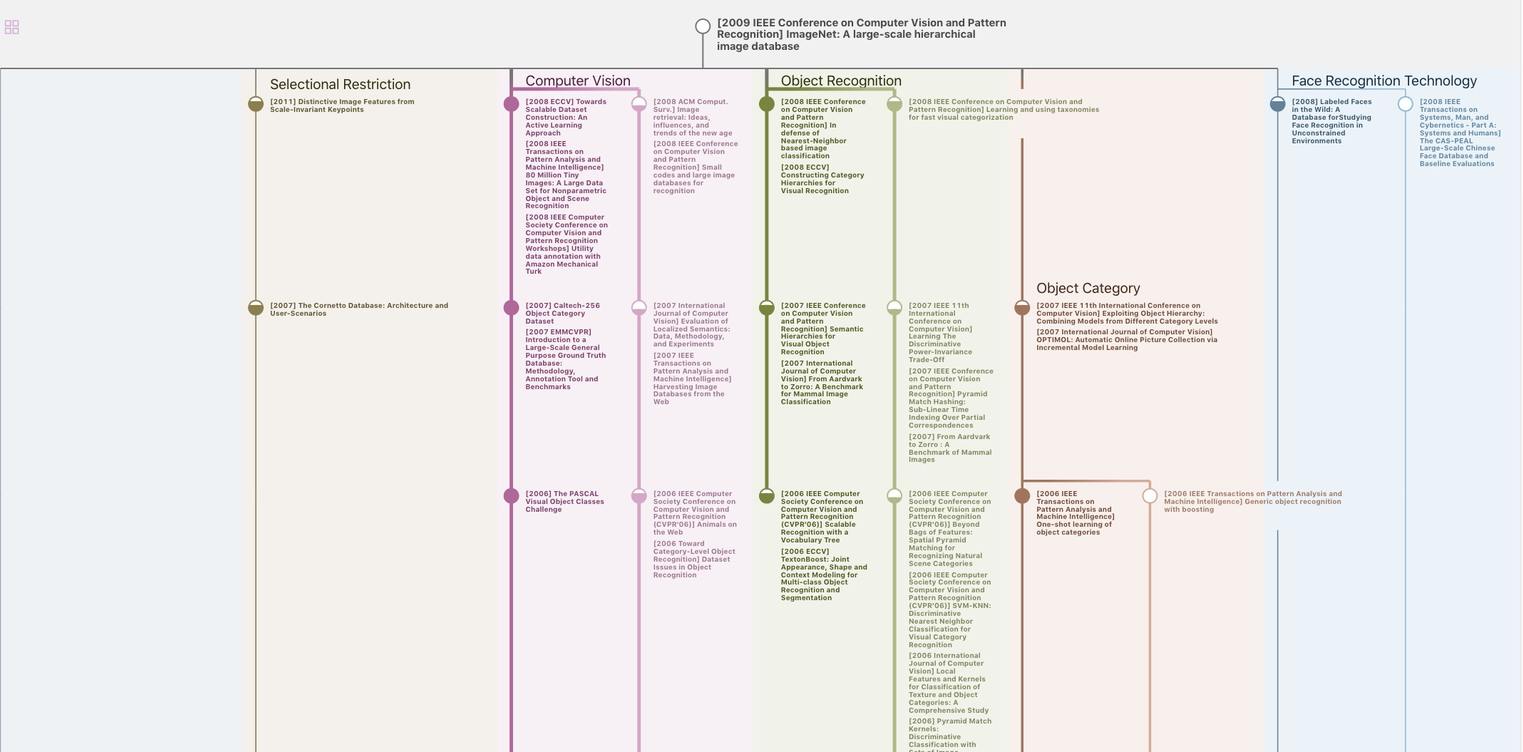
生成溯源树,研究论文发展脉络
Chat Paper
正在生成论文摘要