Driver Fatigue Detection via Eye State Analyses Based on Deep Learning Approach
Innovations in Smart Cities Applications Volume 6(2023)
Abstract
Eye state analysis systems are generally developed in different fields such as driver fatigue detection system, human-computer interaction systems and security systems, etc. This paper proposes a detection system based on Deep Learning (DL) model to detect eye states on dynamic images. This system models in DL method for the classification on eye state information by using the SGD method with different adaptive parameters in daylight conditions. To determine the optimum parameter values in this proposed Convolutional Neural Network (CNN) model, we generated 2 different models as M-1 and M-2 changing the hyperparameters of convolution steps. The generated M-1 model has been designed into 3 different submodels by M-1(a), M-1(b) and M-1(c) as updating the filter number in the convolution layers. In the experiments on these parameters, the average accuracy obtained using the ZJU database is 97.3%, the average accuracy obtained using the YawDD database is 97.9%, the average accuracy obtained using the NTHU-DDD database is 98.4%, and the average accuracy obtained using the KOU-DFD (our own dataset) database is 98.7%. These results prove that our approach is comparable to other state-of-the-art approaches. Moreover, the proposed DL method is more stable and more robust for detecting driver fatigue.
MoreTranslated text
AI Read Science
Must-Reading Tree
Example
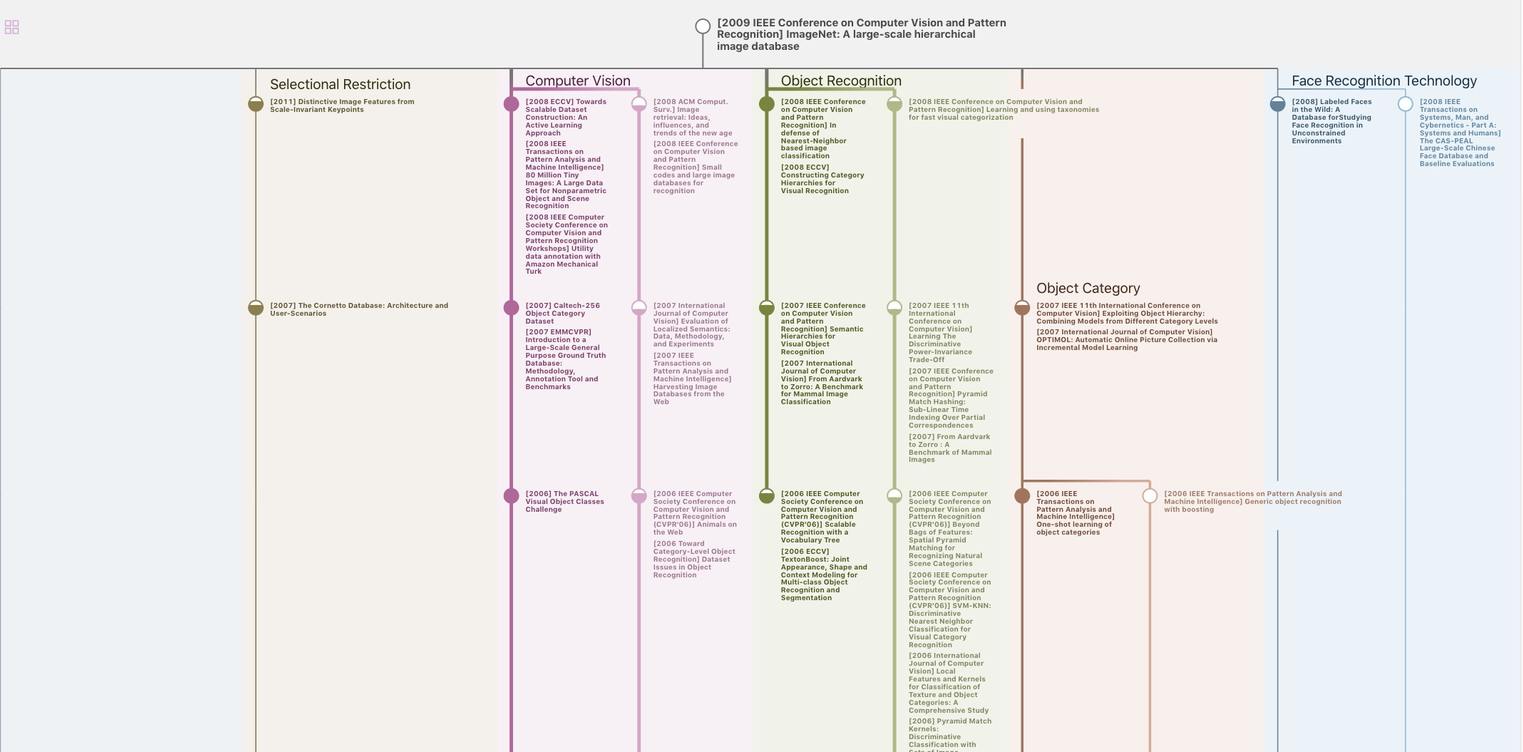
Generate MRT to find the research sequence of this paper
Chat Paper
Summary is being generated by the instructions you defined