One True Pairing: Evaluating Effective Language Pairings for Fake News Detection Employing Zero-Shot Cross-Lingual Transfer
Soft Computing and Its Engineering Applications(2023)
摘要
Fake news poses a great threat to democracy, human rights, health and more. Its viral proliferation, especially in low-resource languages, necessitates automated means for fake news detection be employed to combat the scourge. The improvements demonstrated by cross-lingual language models such as XLM-RoBERTa (XLM-R) and Multilingual BERT (mBERT) in cross-lingual understanding provide an opportunity to develop language-independent models, utilizing zero-shot cross-lingual transfer (i.e., training a model on a dataset in one language and applying it to a dataset in another language with no additional training), to address limitations posed by the lack of training data in low-resource languages. This paper demonstrates that, for particular language pairings, it is possible to employ zero-shot cross-lingual transfer for fake news detection in full-text articles. Utilizing Support Vector Machine and Neural Network classifiers for fake news detection and XLM-R and mBERT embeddings achieves average F1 scores of 0.90 for an Urdu monolingual model evaluated on Bengali fake news dataset; 0.68 for a Bengali monolingual model evaluated on an English fake news dataset; 0.67 for Spanish and Urdu monolingual models on English fake news datasets; 0.67 for Bengali monolingual model evaluating a Spanish fake news dataset. This paper also demonstrates that low-resource language pairings outperform pairings with high-resource language models for fake news detection in low-resource languages.
更多查看译文
关键词
NLP,Cross-lingual language models,Fake news,Low-resource language,Zero-shot cross-lingual transfer,XLM-RoBERTa,Multilingual BERT
AI 理解论文
溯源树
样例
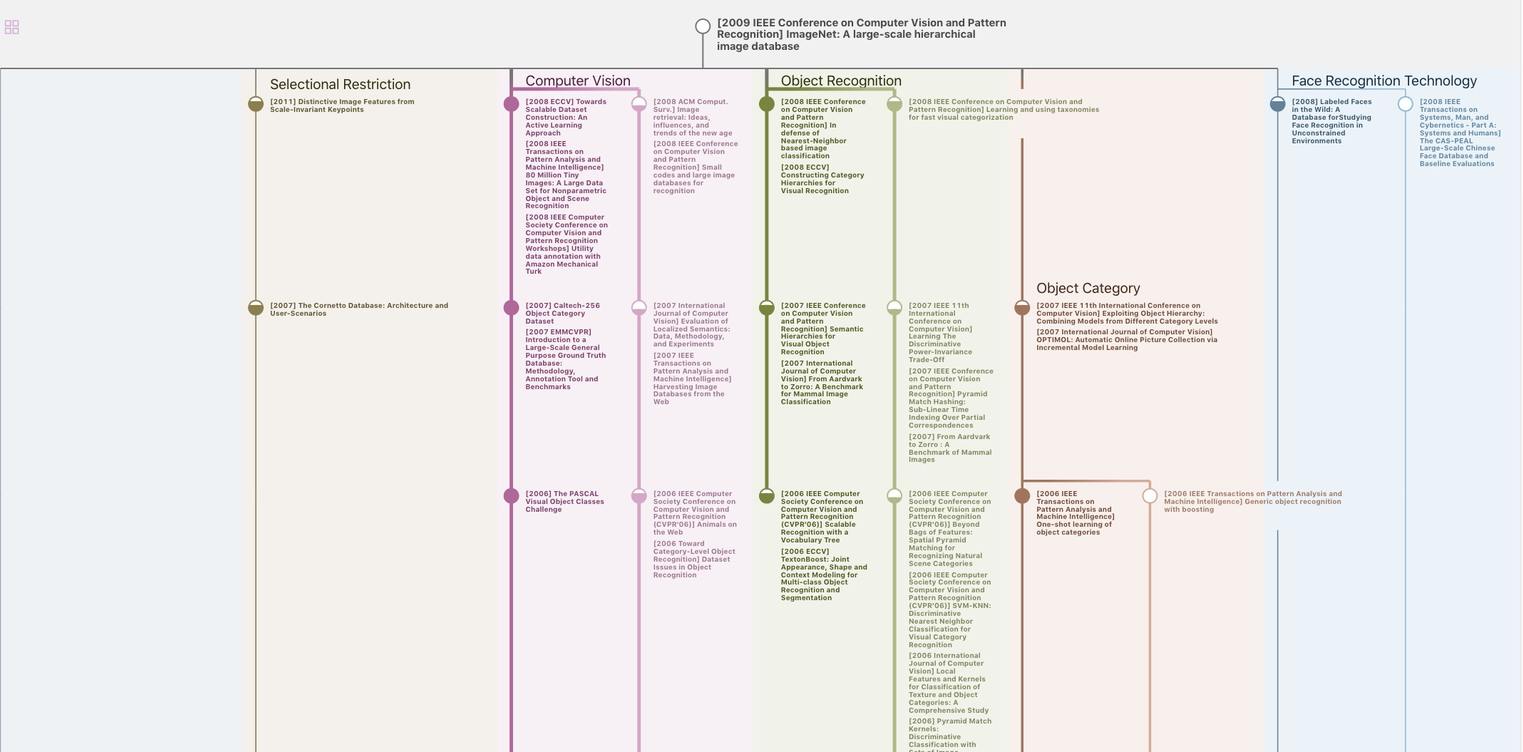
生成溯源树,研究论文发展脉络
Chat Paper
正在生成论文摘要