Automated Training Set Size Reduction for Detection of Small and Thin Objects
12th International Conference on Information Systems and Advanced Technologies “ICISAT 2022”(2023)
摘要
Convolutional neural network-based object detectors such as traffic sign detectors can achieve high accuracy, but are computationally expensive to train. This becomes an acute problem as the size of training sets increase. Thus we need methods to eliminate (1) redundant data in the training sets to reduce the training time and (2) mislabelled data to increase accuracy. Recent works have shown that it is possible to decrease training time, but struggle in improving accuracy. Therefore, we propose three methods for training set size reduction with the aim to improve the detector’s accuracy measured in intersection over union. The first method uses label information to remove images from the training set: we discard images without objects of interest. In contrast, the second method uses feature data of the input images: we discard images that are too similar. Finally, the third method combines the two above-mentioned methods.
更多查看译文
关键词
Training set optimization, Deep learning, Artificial neural network, Object detection, Traffic signs
AI 理解论文
溯源树
样例
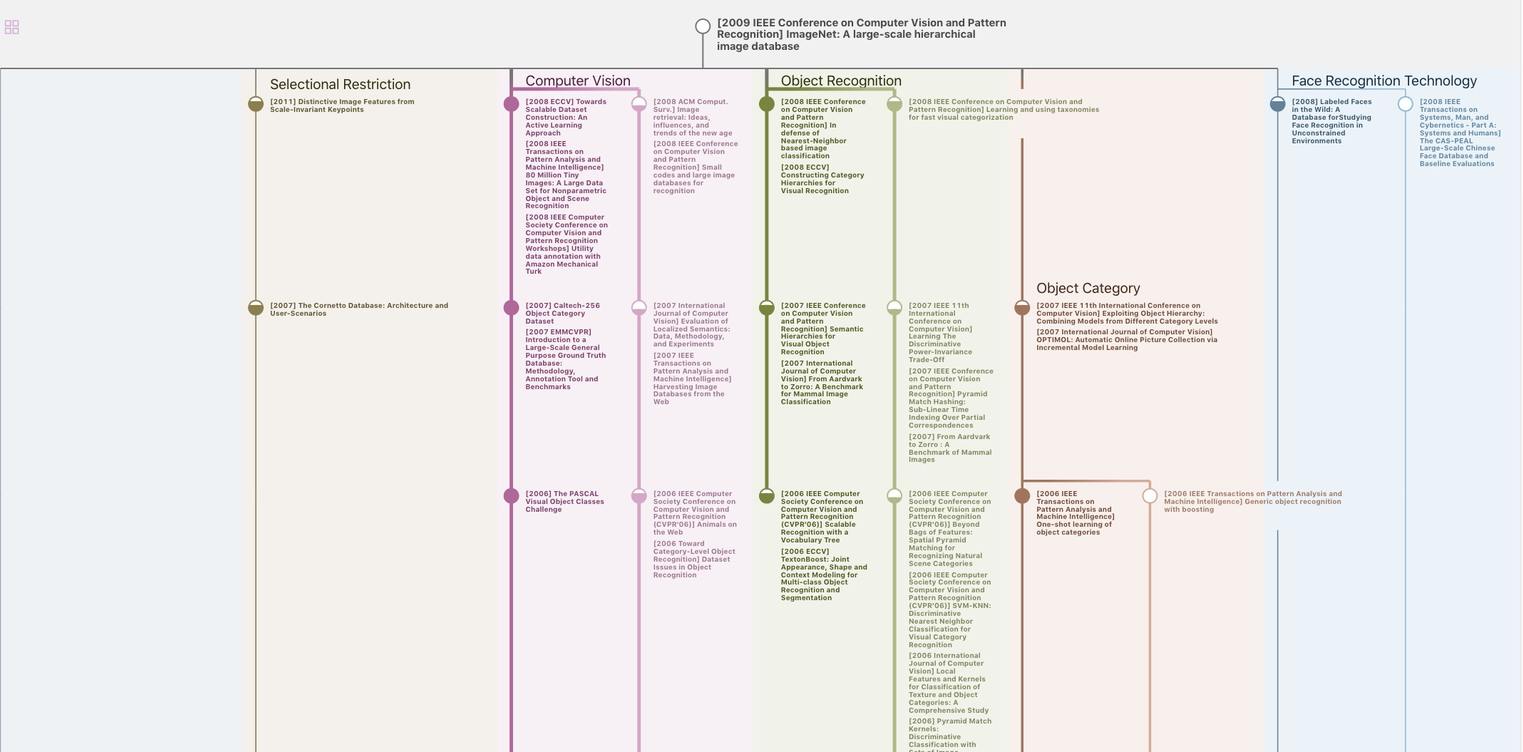
生成溯源树,研究论文发展脉络
Chat Paper
正在生成论文摘要