A Self-adaptive Single-Objective Multitasking Optimization Algorithm
Bio-Inspired Computing: Theories and Applications(2023)
摘要
Evolutionary multitasking optimization algorithms have been presented for dealing with multiple tasks simultaneously. Many studies have proved that EMTOs often perform better than conventional single-task evolutionary. Transferring knowledge plays a very important role in multitask optimization algorithms. Many existing methods transfer elite solutions between tasks to improve algorithm performance, however, these methods may or produce negative transfer if inter-task similarity is low or irrelevant. This paper presents a self-adaptive multitasking optimization algorithm, SAMTOA, to find more valuable transferred solutions between tasks. In SAMTOA, the solutions for the next generation transfer are adaptively determined based on the successful transfer solutions of the previous generation. The method can effectively reduce the probability of transferring useless solutions between tasks and effectively utilize valuable solutions in tasks to improve the efficiency of knowledge transfer between tasks. Experimental results on single-objective multitasking optimization benchmark problems indicate that SAMTOA outperforms the other the state-of-the-art EMTO algorithms.
更多查看译文
关键词
optimization,self-adaptive,single-objective
AI 理解论文
溯源树
样例
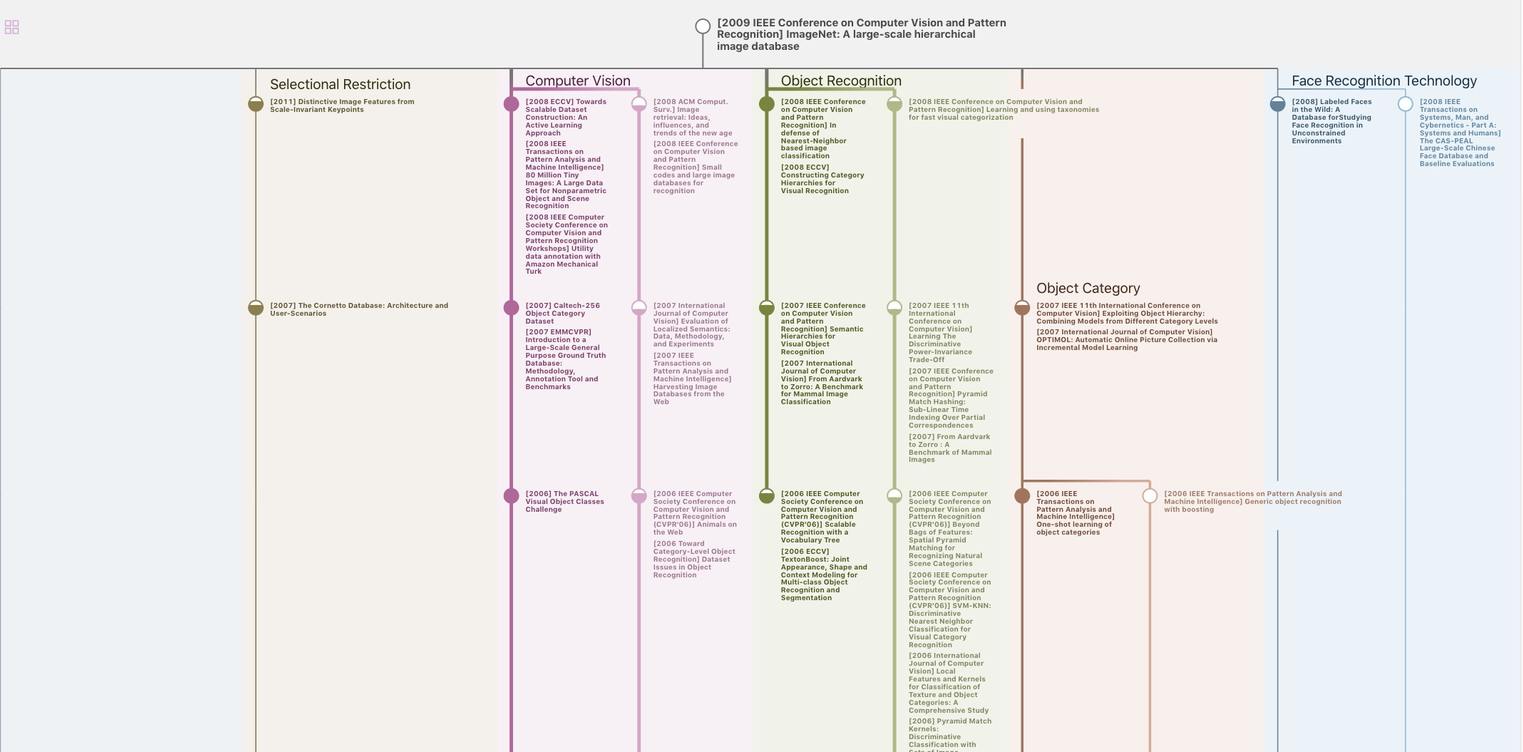
生成溯源树,研究论文发展脉络
Chat Paper
正在生成论文摘要