Unsupervised Fraud Transaction Detection on Dynamic Attributed Networks
Database Systems for Advanced Applications(2023)
摘要
Fraud transaction detection is a pressing need in industrial applications, aiming to detect the fraud for a transaction involving the buyer and the seller. Due to the prohibitive cost of accessing appropriate labels for the task in a supervised fashion, unsupervised anomaly detection has become an alternative solution. However, previous methods mainly handcraft some features to detect the fraud on a single entity, which neglects the dynamic and topological nature between the buyer and the seller within the transaction. In this paper, we propose a novel Temporal Structure Augmented Gaussian Mixture Model (TSAGMM) for unsupervised fraud transaction detection on dynamic attributed networks. Specifically, we propose a time-encoded graph autoencoder to utilize both the topological structure and temporal information within the dynamic transaction graph to reconstruct the node attributes and graph topology. The learned latent representations as well as reconstruction errors are combined and fed into a density-based model for unsupervised fraud detection. Experimental results on the real-world transaction dataset from Alipay show the superiority of our proposed method among the state-of-the-art methods.
更多查看译文
关键词
dynamic attributed networks,detection
AI 理解论文
溯源树
样例
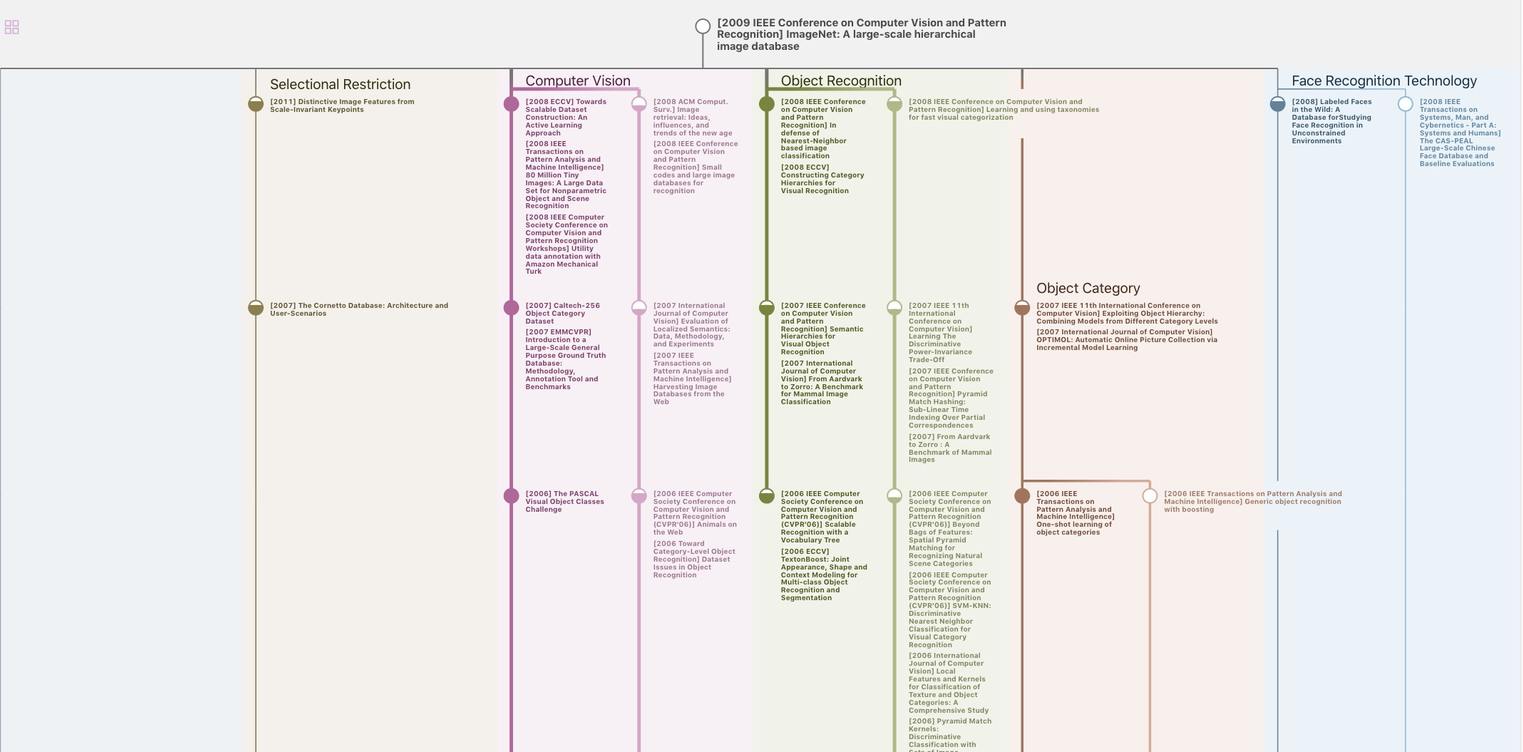
生成溯源树,研究论文发展脉络
Chat Paper
正在生成论文摘要