Evaluating Object Hallucination in Large Vision-Language Models.
CoRR(2023)
摘要
Inspired by the superior language abilities of large language models (LLM), large vision-language models (LVLM) have been recently explored by integrating powerful LLMs for improving the performance on complex multimodal tasks. Despite the promising progress on LVLMs, we find that LVLMs suffer from the hallucination problem, i.e. they tend to generate objects that are inconsistent with the target images in the descriptions. To investigate it, this work presents the first systematic study on object hallucination of LVLMs. We conduct the evaluation experiments on several representative LVLMs, and show that they mostly suffer from severe object hallucination issue. We further discuss that the visual instructions may influence the hallucination, and find that: objects that frequently occur in the visual instructions or co-occur with the image objects, are obviously prone to be hallucinated by LVLMs. Besides, we find that existing evaluation methods might be affected by the input instructions and generation styles of LVLMs. Thus, we further design an improved evaluation method for object hallucination by proposing a polling-based query method called \emph{POPE}. Experiment results demonstrate that our POPE can evaluate the object hallucination in a more stable and flexible way. Our codes and data are publicly available at https://github.com/RUCAIBox/POPE.
更多查看译文
关键词
object hallucination,vision-language
AI 理解论文
溯源树
样例
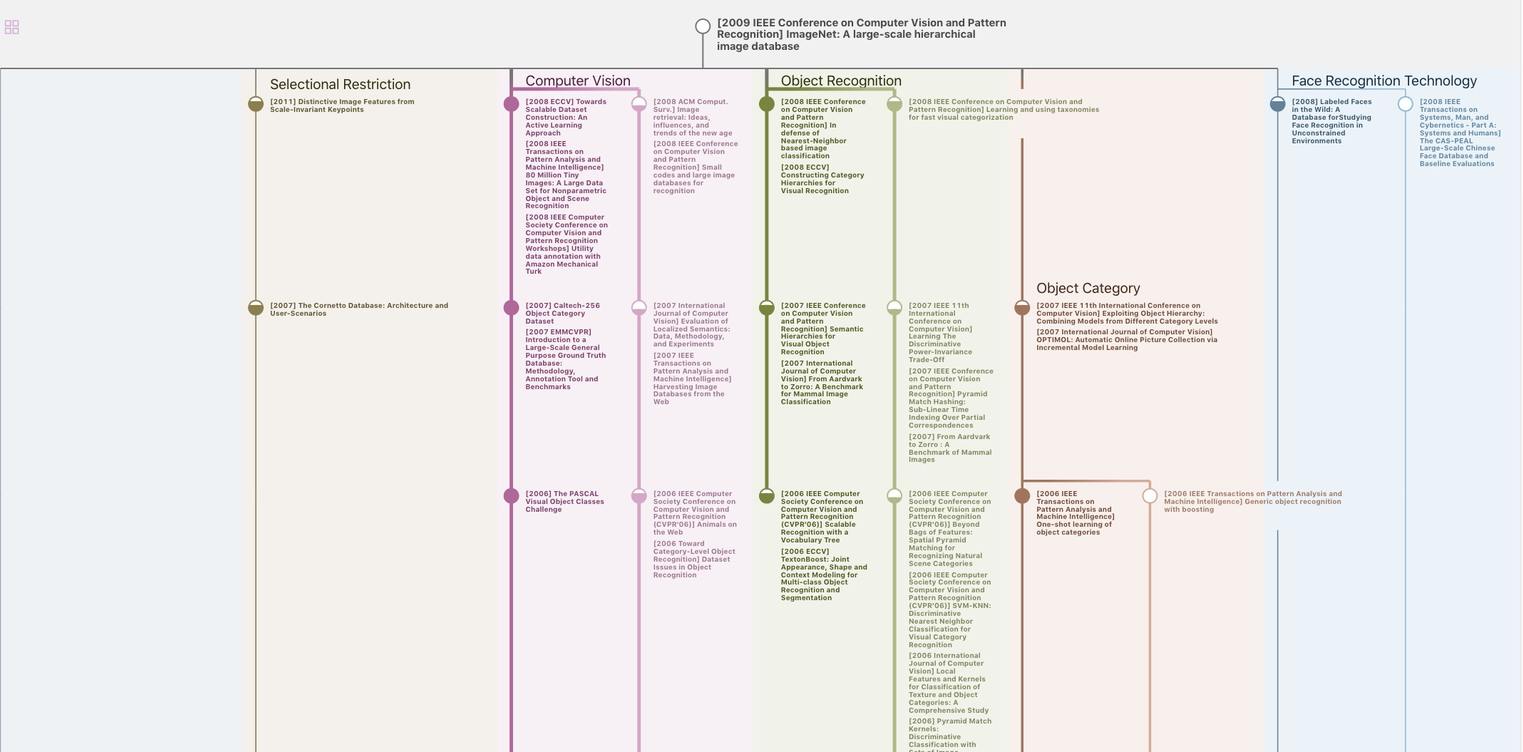
生成溯源树,研究论文发展脉络
Chat Paper
正在生成论文摘要