FICNN: A Framework for the Interpretation of Deep Convolutional Neural Networks
CoRR(2023)
摘要
With the continue development of Convolutional Neural Networks (CNNs), there is a growing concern regarding representations that they encode internally. Analyzing these internal representations is referred to as model interpretation. While the task of model explanation, justifying the predictions of such models, has been studied extensively; the task of model interpretation has received less attention. The aim of this paper is to propose a framework for the study of interpretation methods designed for CNN models trained from visual data. More specifically, we first specify the difference between the interpretation and explanation tasks which are often considered the same in the literature. Then, we define a set of six specific factors that can be used to characterize interpretation methods. Third, based on the previous factors, we propose a framework for the positioning of interpretation methods. Our framework highlights that just a very small amount of the suggested factors, and combinations thereof, have been actually studied. Consequently, leaving significant areas unexplored. Following the proposed framework, we discuss existing interpretation methods and give some attention to the evaluation protocols followed to validate them. Finally, the paper highlights capabilities of the methods in producing feedback for enabling interpretation and proposes possible research problems arising from the framework.
更多查看译文
关键词
deep convolutional neural,ficnn,neural
networks,interpretation
AI 理解论文
溯源树
样例
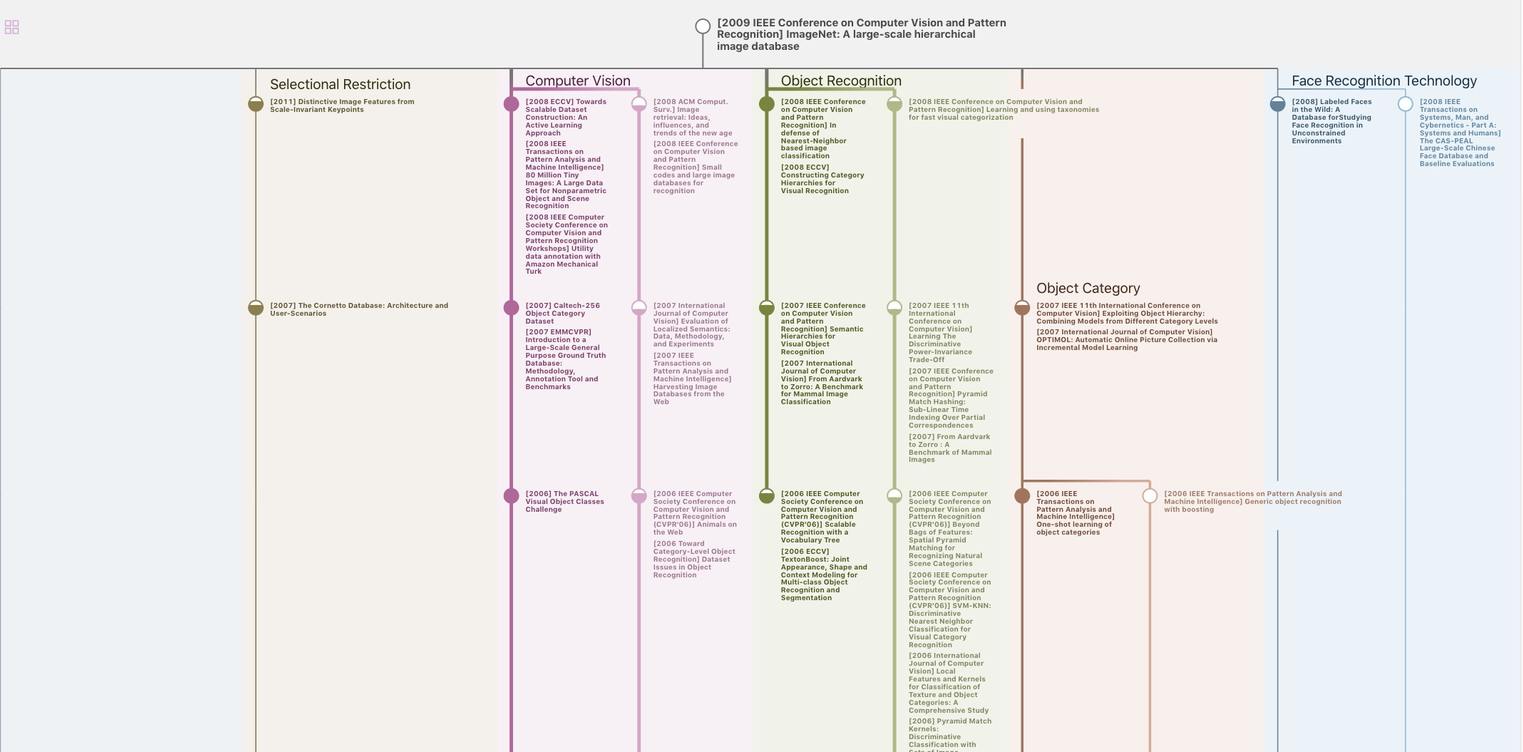
生成溯源树,研究论文发展脉络
Chat Paper
正在生成论文摘要