Selective Amnesia: A Continual Learning Approach to Forgetting in Deep Generative Models
NeurIPS(2023)
摘要
The recent proliferation of large-scale text-to-image models has led to growing concerns that such models may be misused to generate harmful, misleading, and inappropriate content. Motivated by this issue, we derive a technique inspired by continual learning to selectively forget concepts in pretrained deep generative models. Our method, dubbed Selective Amnesia, enables controllable forgetting where a user can specify how a concept should be forgotten. Selective Amnesia can be applied to conditional variational likelihood models, which encompass a variety of popular deep generative frameworks, including variational autoencoders and large-scale text-to-image diffusion models. Experiments across different models demonstrate that our approach induces forgetting on a variety of concepts, from entire classes in standard datasets to celebrity and nudity prompts in text-to-image models. Our code is publicly available at https://github.com/clear-nus/selective-amnesia.
更多查看译文
关键词
continual learning approach,forgetting,deep
AI 理解论文
溯源树
样例
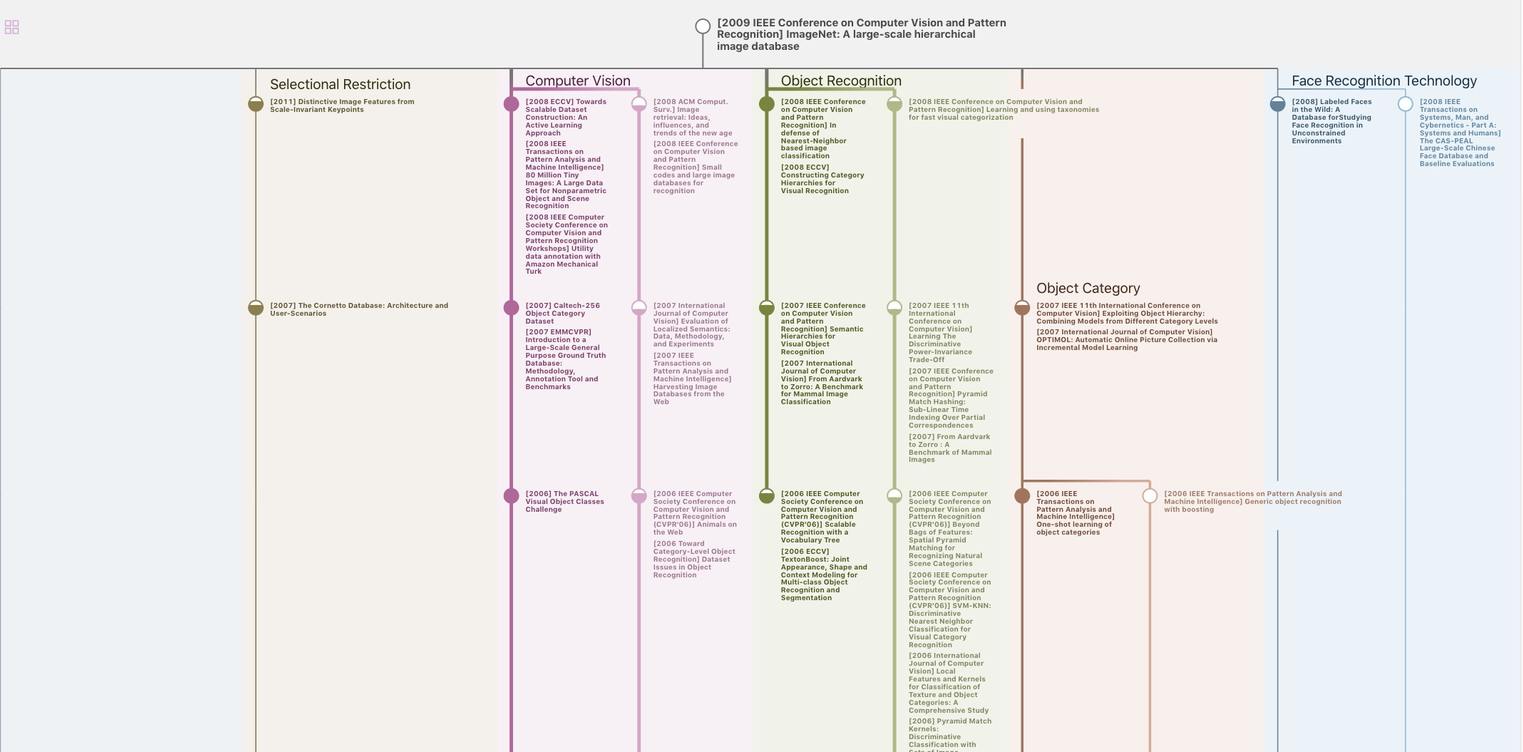
生成溯源树,研究论文发展脉络
Chat Paper
正在生成论文摘要