Causal Discovery with Missing Data in a Multicentric Clinical Study
CoRR(2023)
摘要
Causal inference for testing clinical hypotheses from observational data presents many difficulties because the underlying data-generating model and the associated causal graph are not usually available. Furthermore, observational data may contain missing values, which impact the recovery of the causal graph by causal discovery algorithms: a crucial issue often ignored in clinical studies. In this work, we use data from a multi-centric study on endometrial cancer to analyze the impact of different missingness mechanisms on the recovered causal graph. This is achieved by extending state-of-the-art causal discovery algorithms to exploit expert knowledge without sacrificing theoretical soundness. We validate the recovered graph with expert physicians, showing that our approach finds clinically-relevant solutions. Finally, we discuss the goodness of fit of our graph and its consistency from a clinical decision-making perspective using graphical separation to validate causal pathways.
更多查看译文
关键词
causal discovery,missing data,clinical
AI 理解论文
溯源树
样例
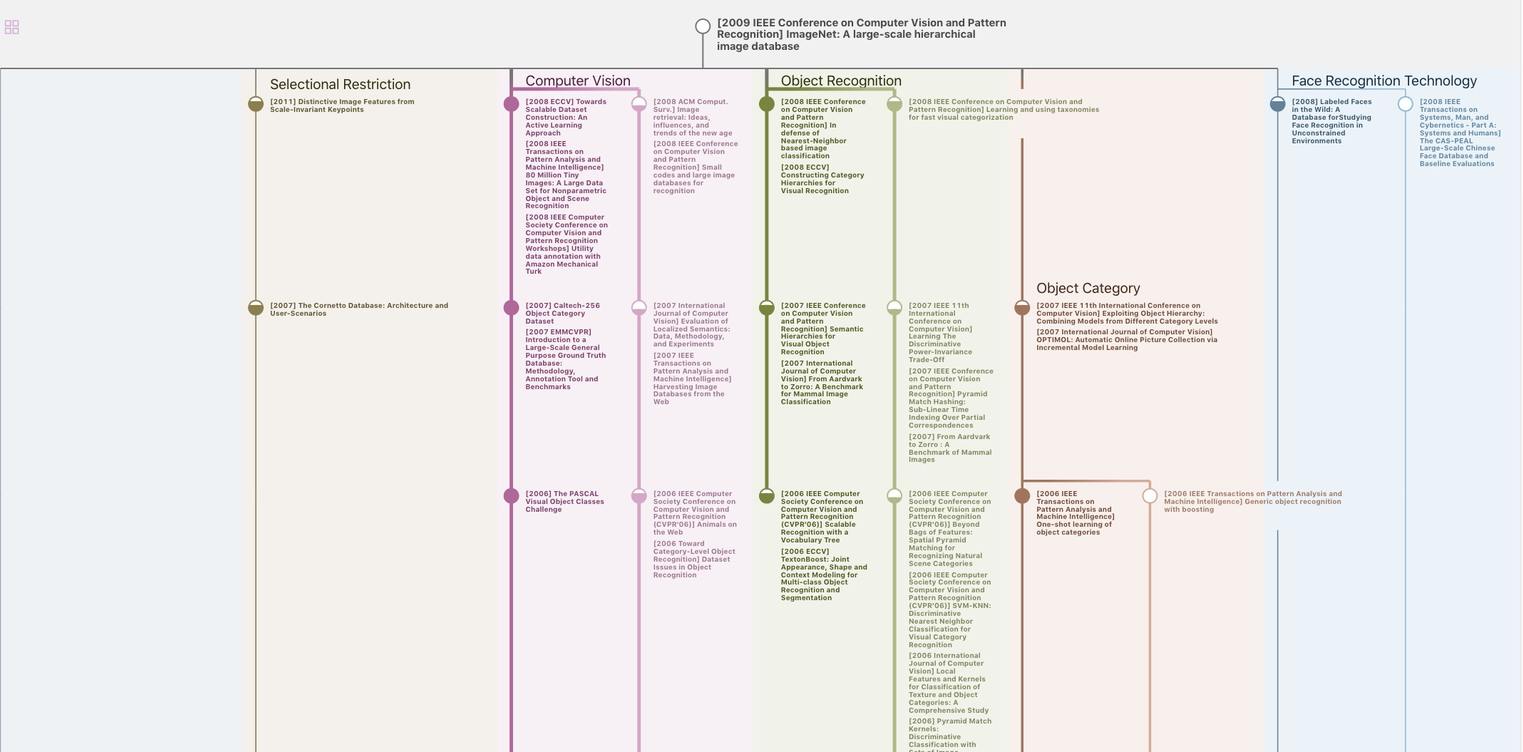
生成溯源树,研究论文发展脉络
Chat Paper
正在生成论文摘要