Data-driven spectral turbulence modelling for Rayleigh-Benard convection
JOURNAL OF FLUID MECHANICS(2023)
摘要
A data-driven turbulence model for coarse-grained numerical simulations of two-dimensional Rayleigh-Benard convection is proposed. The model starts from high-fidelity data and is based on adjusting the Fourier coefficients of the numerical solution, with the aim of accurately reproducing the kinetic energy spectra as seen in the high-fidelity reference findings. No assumptions about the underlying partial differential equation or numerical discretization are used in the formulation of the model. We also develop a constraint on the heat flux to guarantee accurate Nusselt number estimates on coarse computational grids and high Rayleigh numbers. Model performance is assessed in coarse numerical simulations at Ra = 10(10). We focus on key features including kinetic energy spectra, wall-normal flow statistics and global flow statistics. The method of data-driven modelling of flow dynamics is found to reproduce the reference kinetic energy spectra well across all scales and yields good results for flow statistics and average heat transfer, leading to computationally cheap surrogate models. Large-scale forcing extracted from the high-fidelity simulation leads to accurate Nusselt number predictions across two decades of Rayleigh numbers, centred around the targeted reference at Ra = 10(10).
更多查看译文
关键词
Benard convection,turbulence modelling
AI 理解论文
溯源树
样例
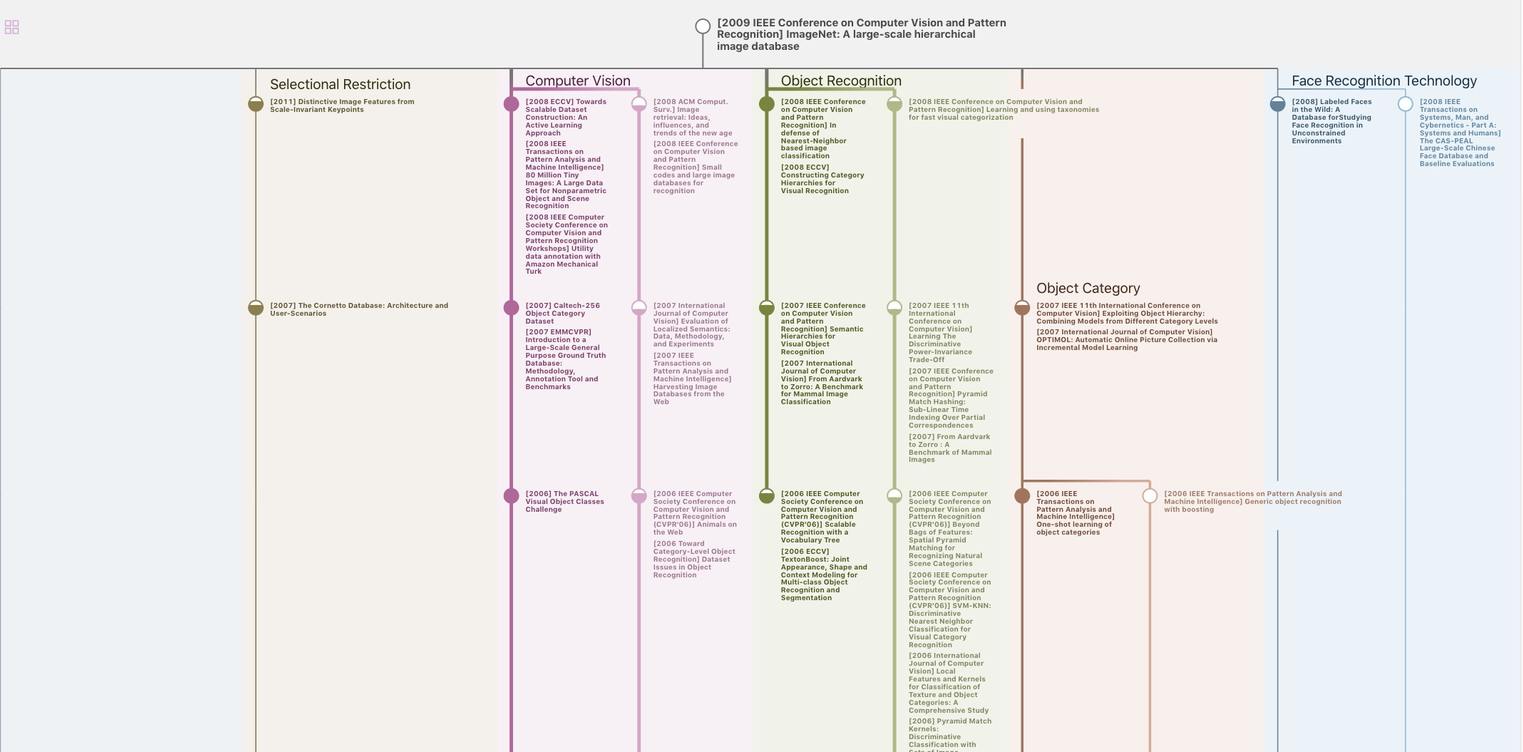
生成溯源树,研究论文发展脉络
Chat Paper
正在生成论文摘要