Link Prediction for Ex Ante Influence Maximization on Temporal Networks
Applied Network Science(2023)
摘要
Influence maximization (IM) is the task of finding the most important nodes in order to maximize the spread of influence or information on a network. This task is typically studied on static or temporal networks where the complete topology of the graph is known. In practice, however, the seed nodes must be selected before observing the future evolution of the network. In this work, we consider this realistic ex ante setting where p time steps of the network have been observed before selecting the seed nodes. Then the influence is calculated after the network continues to evolve for a total of T>p time steps. We address this problem by using statistical, non-negative matrix factorization and graph neural networks link prediction algorithms to predict the future evolution of the network, and then apply existing influence maximization algorithms on the predicted networks. Additionally, the output of the link prediction methods can be used to construct novel IM algorithms. We apply the proposed methods to eight real-world and synthetic networks to compare their performance using the susceptible-infected (SI) diffusion model. We demonstrate that it is possible to construct quality seed sets in the ex ante setting as we achieve influence spread within 87
更多查看译文
关键词
Diffusion,Dynamic networks,Graph neural networks,Influence maximization,Link prediction
AI 理解论文
溯源树
样例
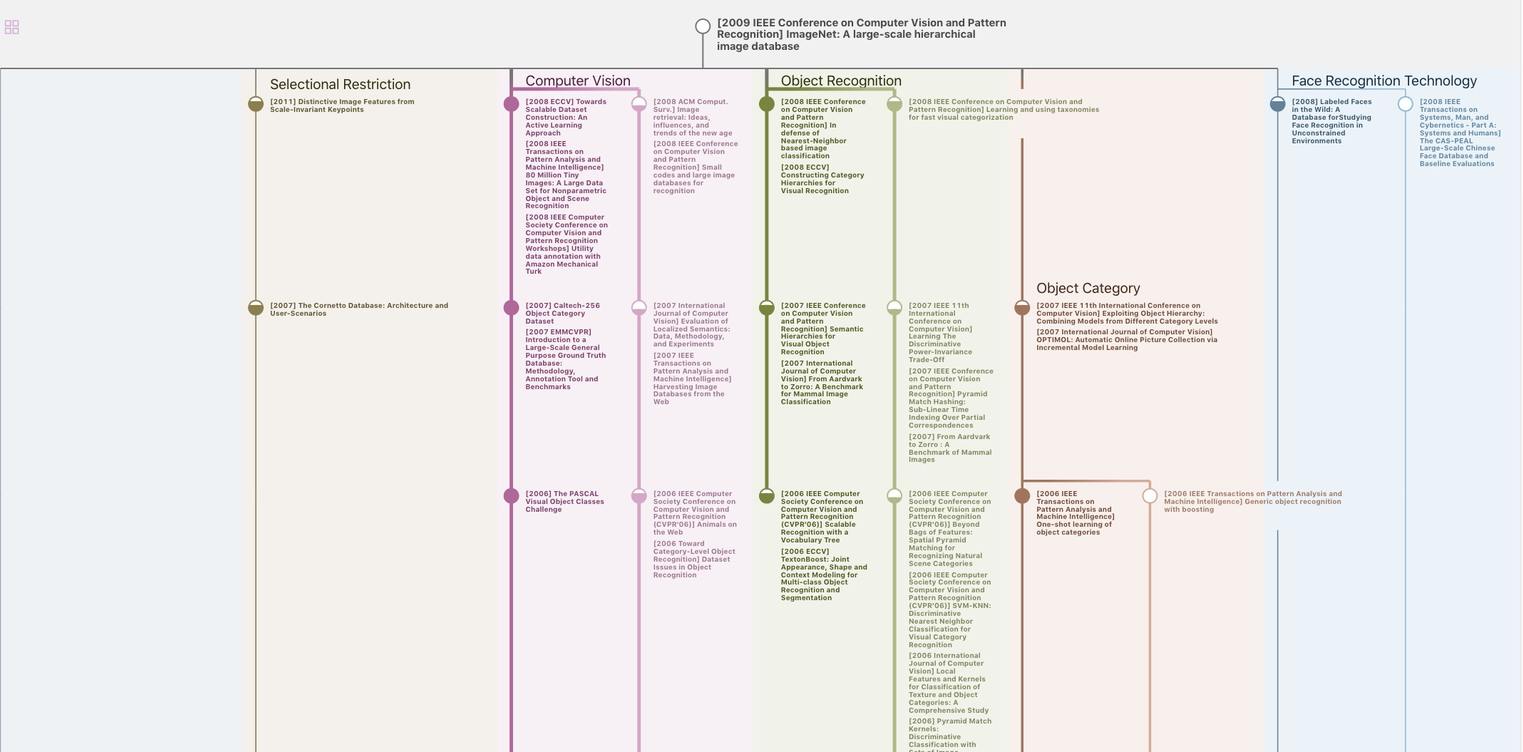
生成溯源树,研究论文发展脉络
Chat Paper
正在生成论文摘要