Mitigating Group Bias in Federated Learning: Beyond Local Fairness
CoRR(2023)
摘要
The issue of group fairness in machine learning models, where certain sub-populations or groups are favored over others, has been recognized for some time. While many mitigation strategies have been proposed in centralized learning, many of these methods are not directly applicable in federated learning, where data is privately stored on multiple clients. To address this, many proposals try to mitigate bias at the level of clients before aggregation, which we call locally fair training. However, the effectiveness of these approaches is not well understood. In this work, we investigate the theoretical foundation of locally fair training by studying the relationship between global model fairness and local model fairness. Additionally, we prove that for a broad class of fairness metrics, the global model's fairness can be obtained using only summary statistics from local clients. Based on that, we propose a globally fair training algorithm that directly minimizes the penalized empirical loss. Real-data experiments demonstrate the promising performance of our proposed approach for enhancing fairness while retaining high accuracy compared to locally fair training methods.
更多查看译文
关键词
federated learning,local fairness,bias,group
AI 理解论文
溯源树
样例
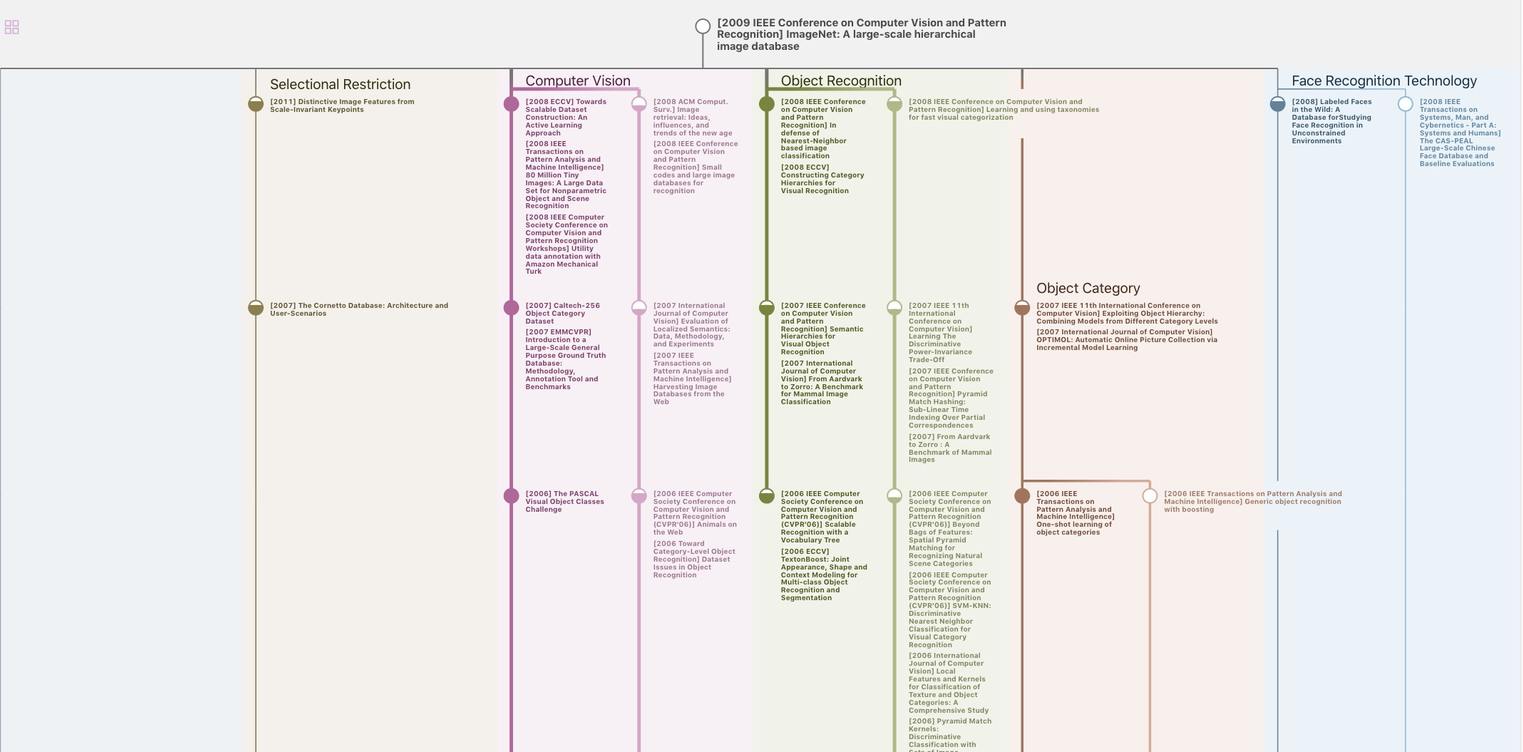
生成溯源树,研究论文发展脉络
Chat Paper
正在生成论文摘要