Dissection of medical AI reasoning processes via physician and generative-AI collaboration
medRxiv : the preprint server for health sciences(2023)
Abstract
Despite the proliferation and clinical deployment of artificial intelligence (AI)-based medical software devices, most remain black boxes that are uninterpretable to key stakeholders including patients, physicians, and even the developers of the devices. Here, we present a general model auditing framework that combines insights from medical experts with a highly expressive form of explainable AI that leverages generative models, to understand the reasoning processes of AI devices. We then apply this framework to generate the first thorough, medically interpretable picture of the reasoning processes of machine-learning–based medical image AI. In our synergistic framework, a generative model first renders "counterfactual" medical images, which in essence visually represent the reasoning process of a medical AI device, and then physicians translate these counterfactual images to medically meaningful features. As our use case, we audit five high-profile AI devices in dermatology, an area of particular interest since dermatology AI devices are beginning to achieve deployment globally. We reveal how dermatology AI devices rely both on features used by human dermatologists, such as lesional pigmentation patterns, as well as multiple, previously unreported, potentially undesirable features, such as background skin texture and image color balance. Our study also sets a precedent for the rigorous application of explainable AI to understand AI in any specialized domain and provides a means for practitioners, clinicians, and regulators to uncloak AI's powerful but previously enigmatic reasoning processes in a medically understandable way.
### Competing Interest Statement
R.D. reports fees from L'Oreal, Frazier Healthcare Partners, Pfizer, DWA, and VisualDx for consulting; stock options from MDAcne and Revea for advisory board; and research funding from UCB.
### Funding Statement
A.J.D., J.D.J., and S.-I.L. were supported by the National Science Foundation (CAREER DBI-1552309 and DBI-1759487) and the National Institutes of Health (R35 GM 128638 and R01 AG061132). R.D. was supported by the National Institutes of Health (5T32 AR007422-38) and the Stanford Catalyst Program.
### Author Declarations
I confirm all relevant ethical guidelines have been followed, and any necessary IRB and/or ethics committee approvals have been obtained.
Yes
The details of the IRB/oversight body that provided approval or exemption for the research described are given below:
The study used ONLY openly available human data that were originally located at: https://challenge.isic-archive.com/data/, https://github.com/mattgroh/fitzpatrick17k, and https://stanfordaimi.azurewebsites.net/datasets/35866158-8196-48d8-87bf-50dca81df965
I confirm that all necessary patient/participant consent has been obtained and the appropriate institutional forms have been archived, and that any patient/participant/sample identifiers included were not known to anyone (e.g., hospital staff, patients or participants themselves) outside the research group so cannot be used to identify individuals.
Yes
I understand that all clinical trials and any other prospective interventional studies must be registered with an ICMJE-approved registry, such as ClinicalTrials.gov. I confirm that any such study reported in the manuscript has been registered and the trial registration ID is provided (note: if posting a prospective study registered retrospectively, please provide a statement in the trial ID field explaining why the study was not registered in advance).
Yes
I have followed all appropriate research reporting guidelines, such as any relevant EQUATOR Network research reporting checklist(s) and other pertinent material, if applicable.
Yes
Images used in this study were obtained from publicly available repositories. ISIC images are available at https://challenge.isic-archive.com/data/. Fitzpatrick17k images are available at https://github.com/mattgroh/fitzpatrick17k. The DDI images are available at https://stanfordaimi.azurewebsites.net/datasets/35866158-8196-48d8-87bf-50dca81df965. Model weights for the DeepDerm classifier are available at https://zenodo.org/record/6784279#.ZFrDc9LMK-Z. The weights and model specification for the ModelDerm classifier are available at https://figshare.com/articles/Caffemodel\_files\_and\_Python\_Examples/5406223. Model weights for our retrained variant of the SIIM-ISIC competition classifier are available at https://drive.google.com/drive/folders/1Zn7hNRgiI2jt7vpZO1ohpr-so9YztCCb. Scanoma and Smart Skin Cancer Detection are third party software for which we cannot redistribute model weights. At the time of writing, both are apps are available for download with no fee from the Google Play store and third-party APK package download sites. Our code, including a PyTorch implementation of explanation by progressive exaggeration and classes for loading datasets and classifiers are available at https://github.com/suinleelab/derm_audit. Weights for our trained generative models and the re-trained SIIM-ISIC classifier are available at https://drive.google.com/drive/folders/1Zn7hNRgiI2jt7vpZO1ohpr-so9YztCCb.
MoreTranslated text
Key words
medical ai reasoning processes,physician
AI Read Science
Must-Reading Tree
Example
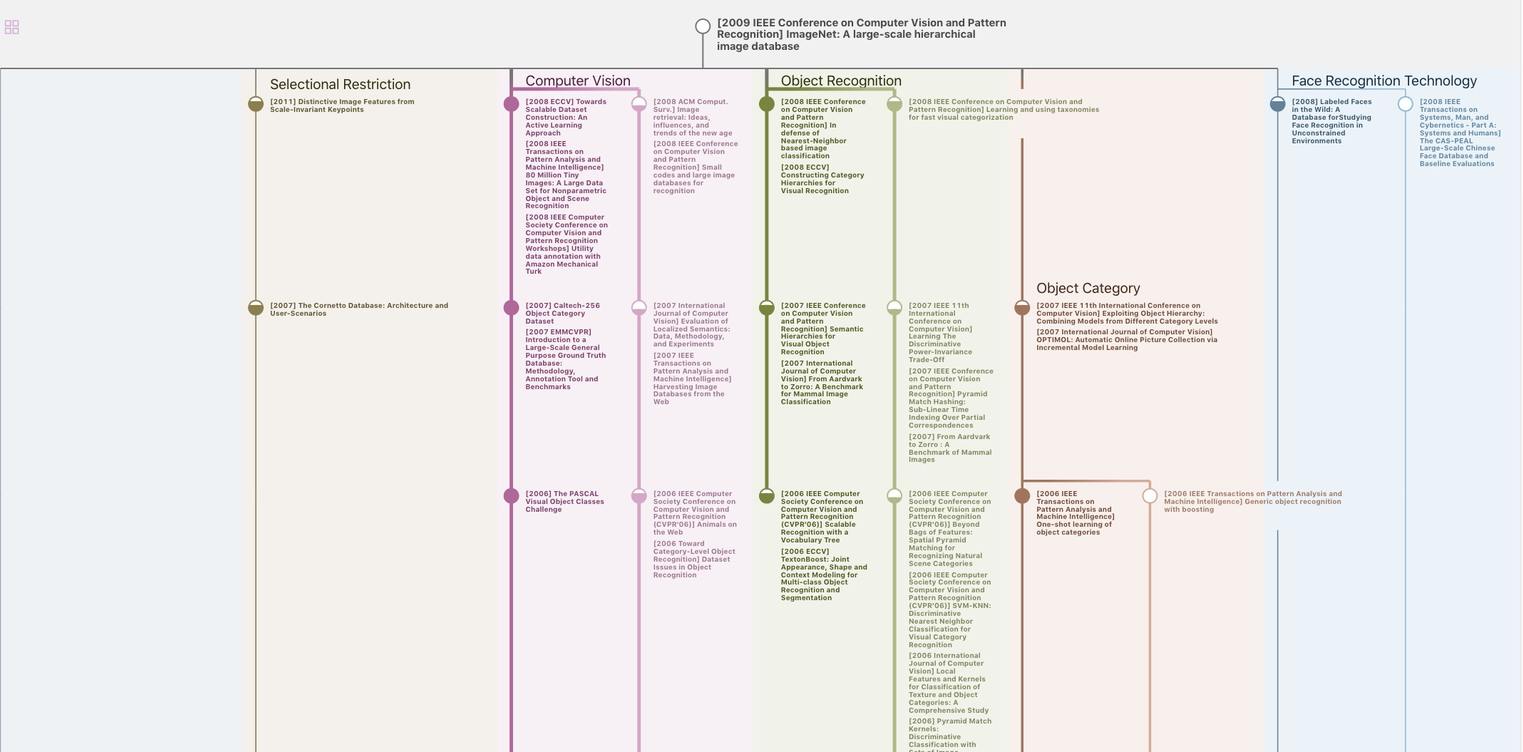
Generate MRT to find the research sequence of this paper
Chat Paper
Summary is being generated by the instructions you defined