Concurrent Misclassification and Out-of-Distribution Detection for Semantic Segmentation via Energy-Based Normalizing Flow
CoRR(2023)
摘要
Recent semantic segmentation models accurately classify test-time examples that are similar to a training dataset distribution. However, their discriminative closed-set approach is not robust in practical data setups with distributional shifts and out-of-distribution (OOD) classes. As a result, the predicted probabilities can be very imprecise when used as confidence scores at test time. To address this, we propose a generative model for concurrent in-distribution misclassification (IDM) and OOD detection that relies on a normalizing flow framework. The proposed flow-based detector with an energy-based inputs (FlowEneDet) can extend previously deployed segmentation models without their time-consuming retraining. Our FlowEneDet results in a low-complexity architecture with marginal increase in the memory footprint. FlowEneDet achieves promising results on Cityscapes, Cityscapes-C, FishyScapes and SegmentMeIfYouCan benchmarks in IDM/OOD detection when applied to pretrained DeepLabV3+ and SegFormer semantic segmentation models.
更多查看译文
关键词
semantic segmentation,concurrent misclassification,out-of-distribution,energy-based
AI 理解论文
溯源树
样例
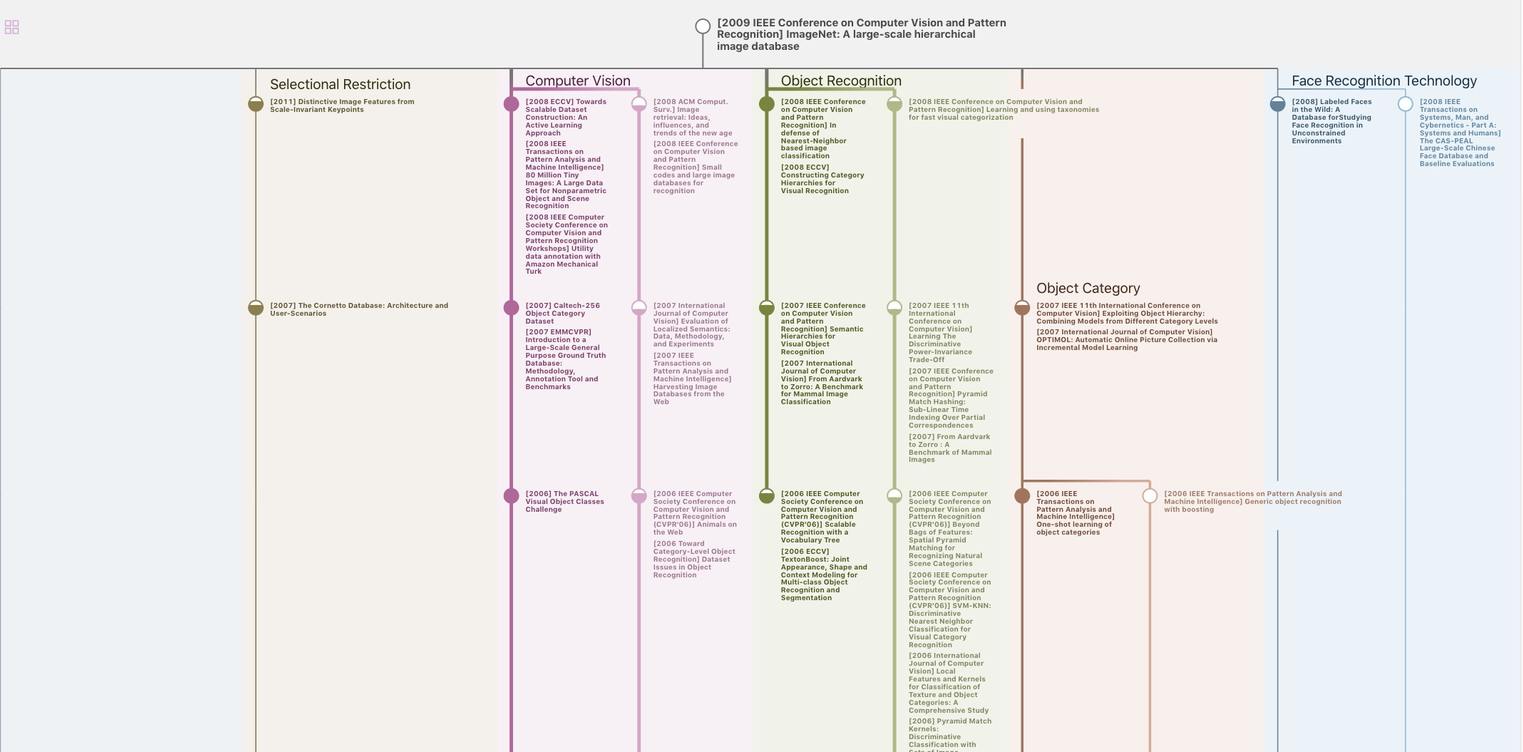
生成溯源树,研究论文发展脉络
Chat Paper
正在生成论文摘要