Deep Reinforcement Learning to Maximize Arterial Usage during Extreme Congestion
CoRR(2023)
摘要
Collisions, crashes, and other incidents on road networks, if left unmitigated, can potentially cause cascading failures that can affect large parts of the system. Timely handling such extreme congestion scenarios is imperative to reduce emissions, enhance productivity, and improve the quality of urban living. In this work, we propose a Deep Reinforcement Learning (DRL) approach to reduce traffic congestion on multi-lane freeways during extreme congestion. The agent is trained to learn adaptive detouring strategies for congested freeway traffic such that the freeway lanes along with the local arterial network in proximity are utilized optimally, with rewards being congestion reduction and traffic speed improvement. The experimental setup is a 2.6-mile-long 4-lane freeway stretch in Shoreline, Washington, USA with two exits and associated arterial roads simulated on a microscopic and continuous multi-modal traffic simulator SUMO (Simulation of Urban MObility) while using parameterized traffic profiles generated using real-world traffic data. Our analysis indicates that DRL-based controllers can improve average traffic speed by 21\% when compared to no-action during steep congestion. The study further discusses the trade-offs involved in the choice of reward functions, the impact of human compliance on agent performance, and the feasibility of knowledge transfer from one agent to other to address data sparsity and scaling issues.
更多查看译文
关键词
arterial usage,reinforcement
AI 理解论文
溯源树
样例
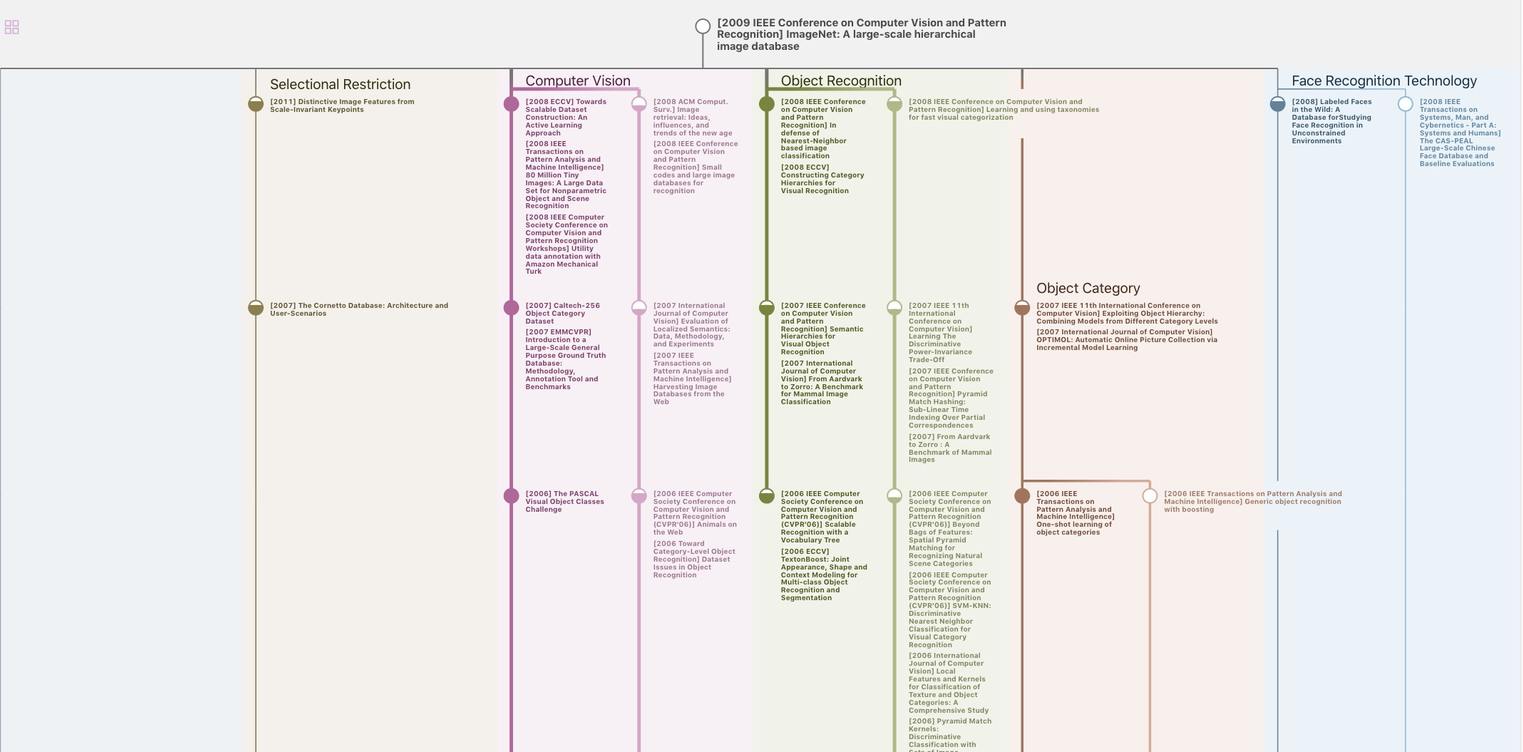
生成溯源树,研究论文发展脉络
Chat Paper
正在生成论文摘要