Enhancing Keyphrase Extraction from Long Scientific Documents using Graph Embeddings
CoRR(2023)
摘要
In this study, we investigate using graph neural network (GNN) representations to enhance contextualized representations of pre-trained language models (PLMs) for keyphrase extraction from lengthy documents. We show that augmenting a PLM with graph embeddings provides a more comprehensive semantic understanding of words in a document, particularly for long documents. We construct a co-occurrence graph of the text and embed it using a graph convolutional network (GCN) trained on the task of edge prediction. We propose a graph-enhanced sequence tagging architecture that augments contextualized PLM embeddings with graph representations. Evaluating on benchmark datasets, we demonstrate that enhancing PLMs with graph embeddings outperforms state-of-the-art models on long documents, showing significant improvements in F1 scores across all the datasets. Our study highlights the potential of GNN representations as a complementary approach to improve PLM performance for keyphrase extraction from long documents.
更多查看译文
关键词
keyphrase extraction,long scientific documents,graph embeddings
AI 理解论文
溯源树
样例
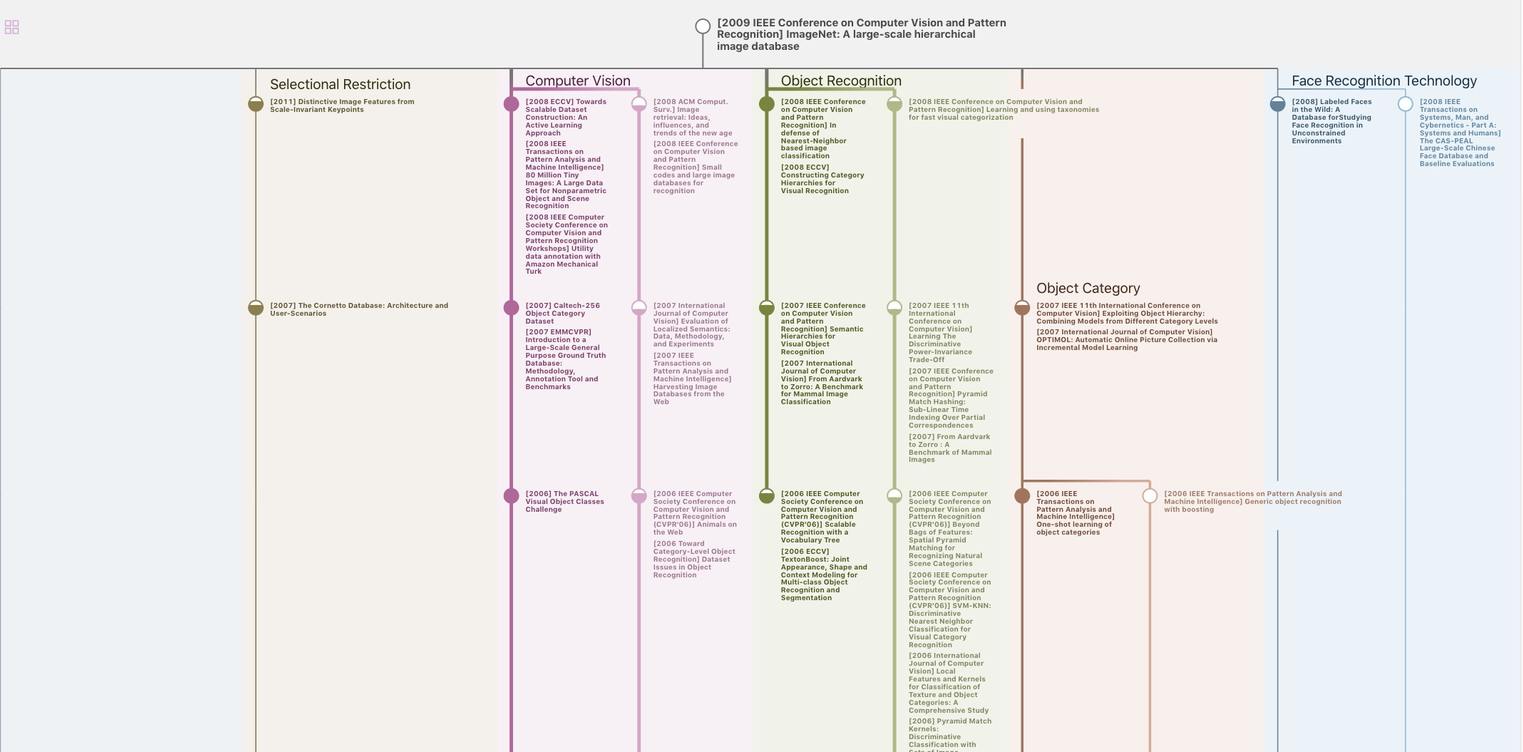
生成溯源树,研究论文发展脉络
Chat Paper
正在生成论文摘要