Easy-to-Hard Learning for Information Extraction
conf_acl(2023)
摘要
Information extraction (IE) systems aim to automatically extract structured information, such as named entities, relations between entities, and events, from unstructured texts. While most existing work addresses a particular IE task, universally modeling various IE tasks with one model has achieved great success recently. Despite their success, they employ a one-stage learning strategy, i.e., directly learning to extract the target structure given the input text, which contradicts the human learning process. In this paper, we propose a unified easy-to-hard learning framework consisting of three stages, i.e., the easy stage, the hard stage, and the main stage, for IE by mimicking the human learning process. By breaking down the learning process into multiple stages, our framework facilitates the model to acquire general IE task knowledge and improve its generalization ability. Extensive experiments across four IE tasks demonstrate the effectiveness of our framework. We achieve new state-of-the-art results on 13 out of 17 datasets. Our code is available at \url{https://github.com/DAMO-NLP-SG/IE-E2H}.
更多查看译文
关键词
extraction,information,learning,easy-to-hard
AI 理解论文
溯源树
样例
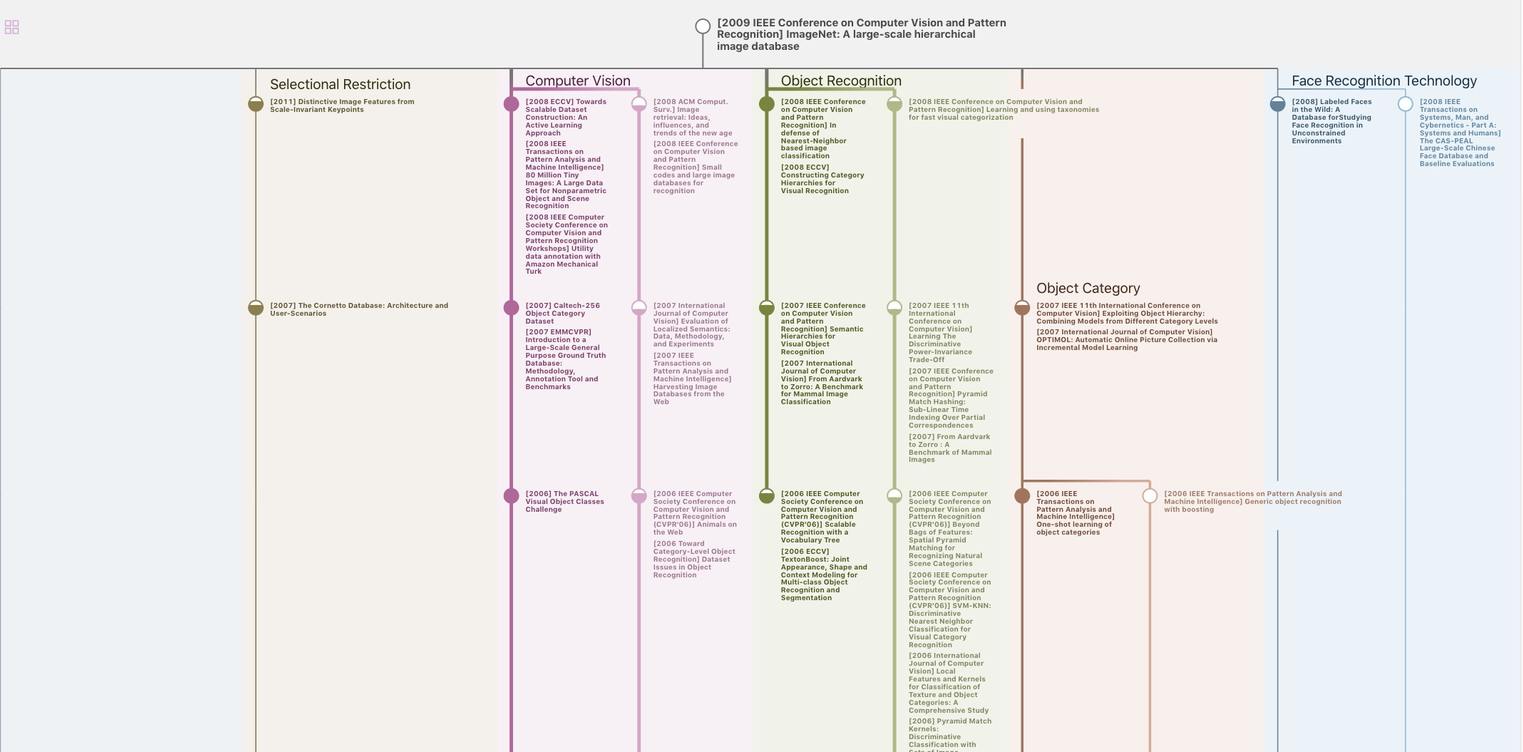
生成溯源树,研究论文发展脉络
Chat Paper
正在生成论文摘要