A Causal Inference Framework for Leveraging External Controls in Hybrid Trials
arXiv (Cornell University)(2023)
摘要
We consider the challenges associated with causal inference in settings where data from a randomized trial is augmented with control data from an external source to improve efficiency in estimating the average treatment effect (ATE). Through the development of a formal causal inference framework, we outline sufficient causal assumptions about the exchangeability between the internal and external controls to identify the ATE and establish the connection to a novel graphical criteria. We propose estimators, review efficiency bounds, develop an approach for efficient doubly-robust estimation even when unknown nuisance models are estimated with flexible machine learning methods, and demonstrate finite-sample performance through a simulation study. To illustrate the ideas and methods, we apply the framework to a trial investigating the effect of risdisplam on motor function in patients with spinal muscular atrophy for which there exists an external set of control patients from a previous trial.
更多查看译文
关键词
causal inference framework,trials,external controls
AI 理解论文
溯源树
样例
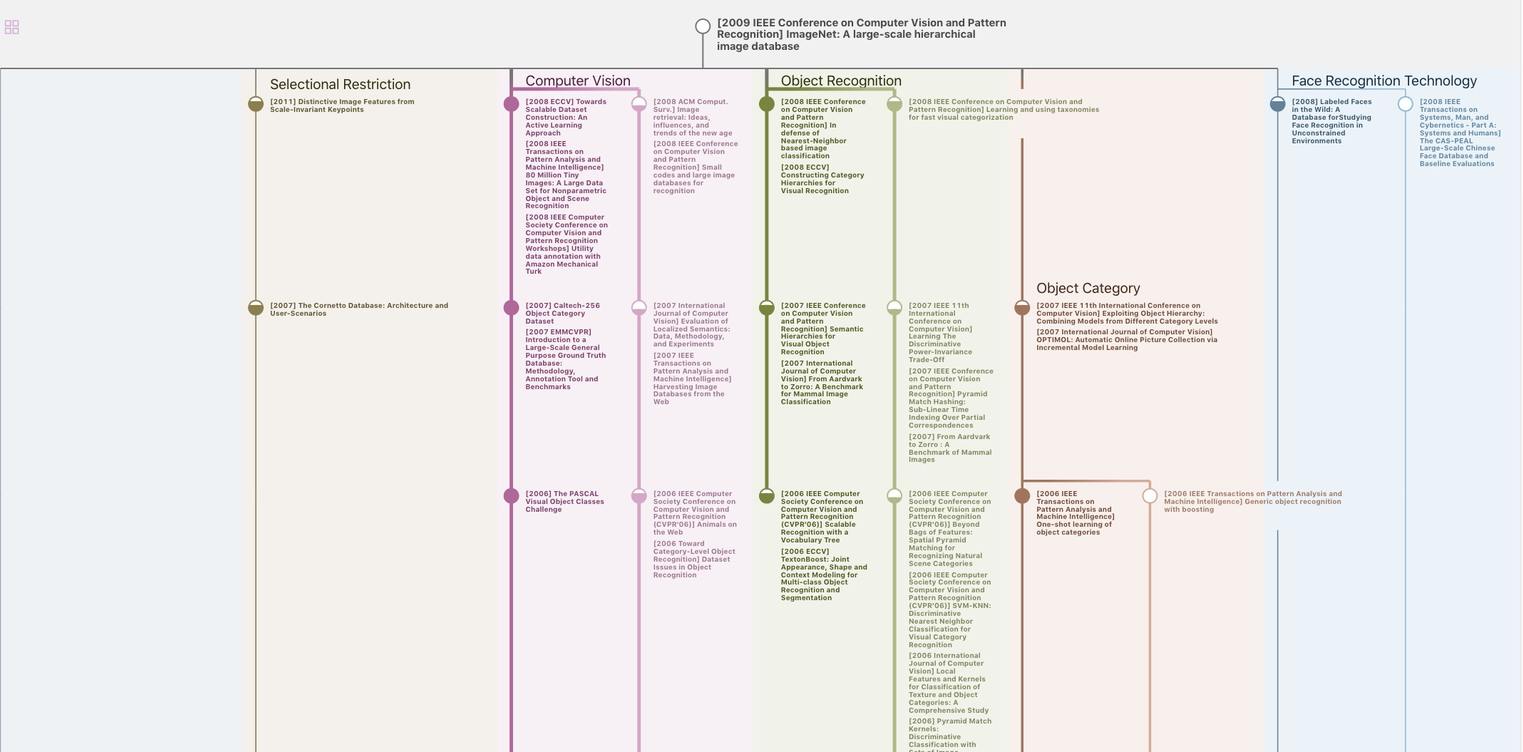
生成溯源树,研究论文发展脉络
Chat Paper
正在生成论文摘要