Stacked Bin Convolutional Neural Networks based Sparse Low-Rank Regressor: Robust, Scalable and Novel Model for Memorability Prediction of Videos
Multimedia Tools and Applications(2023)
摘要
Driven by Internet technology, the explosion of video content is growing exponentially. Hence, the need for video analysis and interpretation by human cognition is taken into account. Memorability is one of the cognitive measures that define the recall ability of watched visual content. Prior research works are done on image memorability, and video memorability modeling is least focused. Moreover, the computational features need to be robust to build a strong video memorability predictor model that is also not focused earlier. This paper proposes a robust, scalable and novel Stacked Bin- Convolutional Neural Network (SB_CNN) based Sparse Low-Rank Regressor (SLRR) model that classifies and predicts the interesting events of videos by performing a robust feature scaling process and intelligent memorability tasks. Inspired by the Low-Rank Representation (LRR) property in noise data handling, the proposed feature extraction process covered the low-rank issue on attributes such as objects, scenes, and annotated to the original feature space. The estimated sparse coefficient vector is fed into the Stacked Bin- Convolutional Neural Networks (SB-CNN) model that establishes the connections between video frames and their memorability scores by means of electing the best frames by following the objectives of Multi-Attribute Decision Making (MADM) technique. The selected frames are fed into the CNN classifier. With the estimated decision score, the proposed classifier has effectively predicted the events from relevant video with the better recall time 49. 9247. Experiments conducted on public datasets, SumMe and SUN database demonstrates the efficacy of the proposed technique.
更多查看译文
关键词
Video memorability,Human cognition,Recall,Low-rank representation,Stacked bin,Convolutional Neural Networks &Feature extraction
AI 理解论文
溯源树
样例
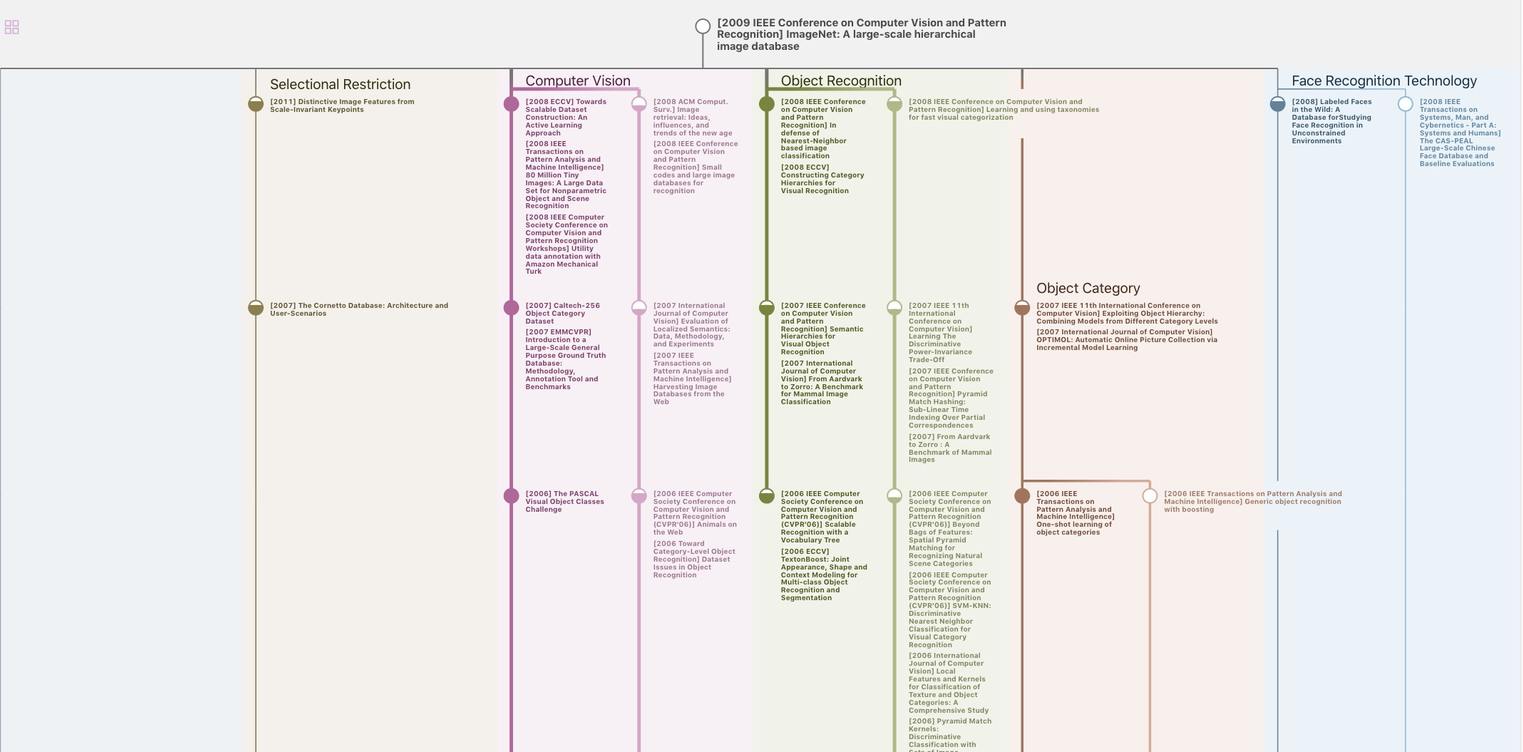
生成溯源树,研究论文发展脉络
Chat Paper
正在生成论文摘要