Towards Practical Few-shot Federated NLP
EuroMLSys '23: Proceedings of the 3rd Workshop on Machine Learning and Systems(2023)
摘要
Transformer-based pre-trained models have emerged as the predominant solution for natural language processing (NLP). Fine-tuning such pre-trained models for downstream tasks often requires a considerable amount of labeled private data. In practice, private data is often distributed across heterogeneous mobile devices and may be prohibited from being uploaded. Moreover, well-curated labeled data is often scarce, presenting an additional challenge. To address these challenges, we first introduce a data generator for federated few-shot learning tasks, which encompasses the quantity and skewness of scarce labeled data in a realistic setting. Subsequently, we propose AUG-FedPrompt, a prompt -based fed erated learning system that exploits abundant unlabeled data for data aug mentation. Our experiments indicate that AUG-FedPrompt can perform on par with full-set fine-tuning with a limited amount of labeled data. However, such competitive performance comes at a significant system cost.
更多查看译文
关键词
few-shot
AI 理解论文
溯源树
样例
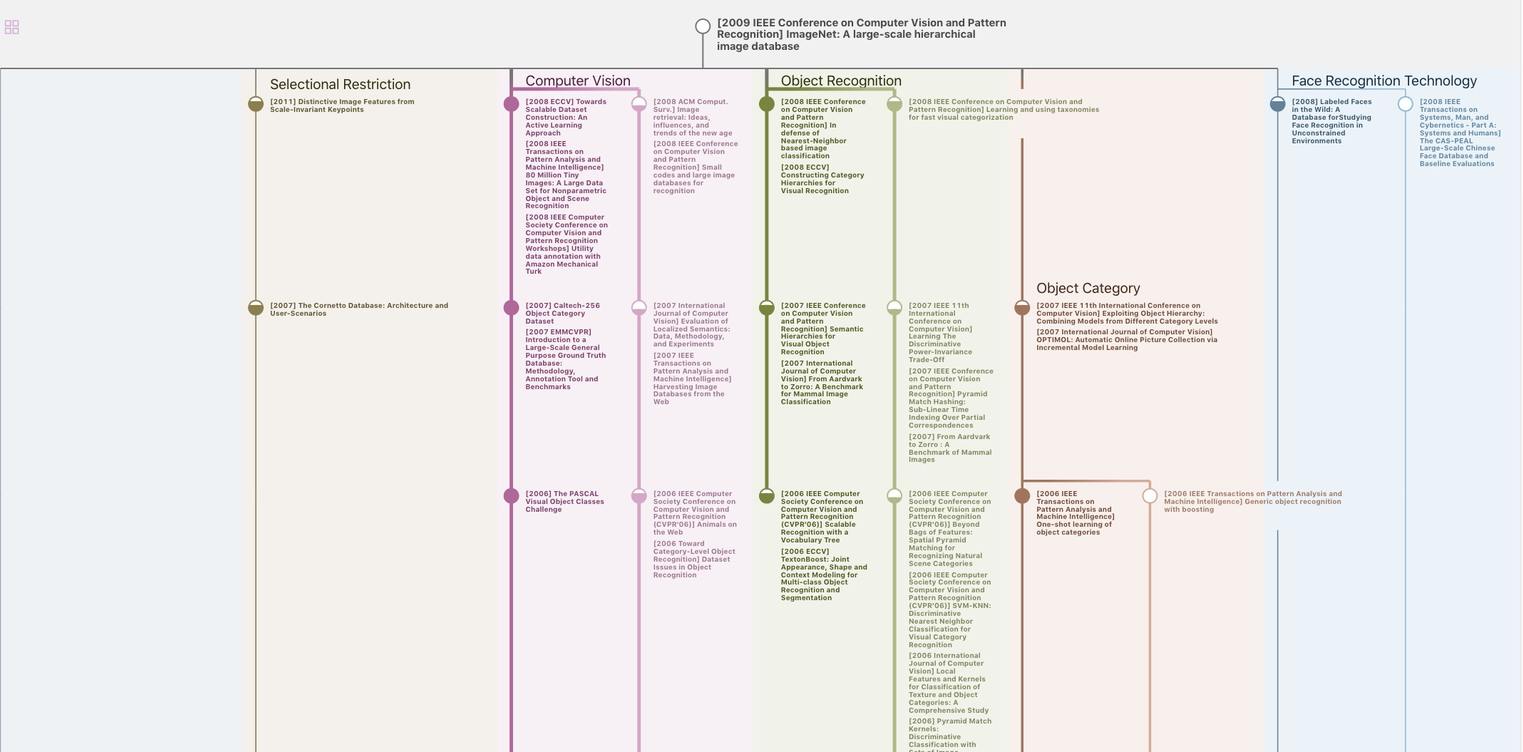
生成溯源树,研究论文发展脉络
Chat Paper
正在生成论文摘要