Best of both, Structured and Unstructured Sparsity in Neural Networks
EuroMLSys '23: Proceedings of the 3rd Workshop on Machine Learning and Systems(2023)
摘要
Besides quantization, pruning has shown to be one of the most effective methods to reduce the inference time and required energy of Deep Neural Networks (DNNs). In this work, we propose a sparsity definition that reflects the number of saved operations by pruned parameters to guide the pruning process in order to save as many operations as possible. Based on this, we show the importance of the baseline model's size and quantify the overhead of unstructured sparsity for a commercial-of-the-shelf AI Hardware Accelerator (HWA) in terms of latency reductions. Furthermore, we show that a combination of both structured and unstructured sparsity can mitigate this effect.
更多查看译文
AI 理解论文
溯源树
样例
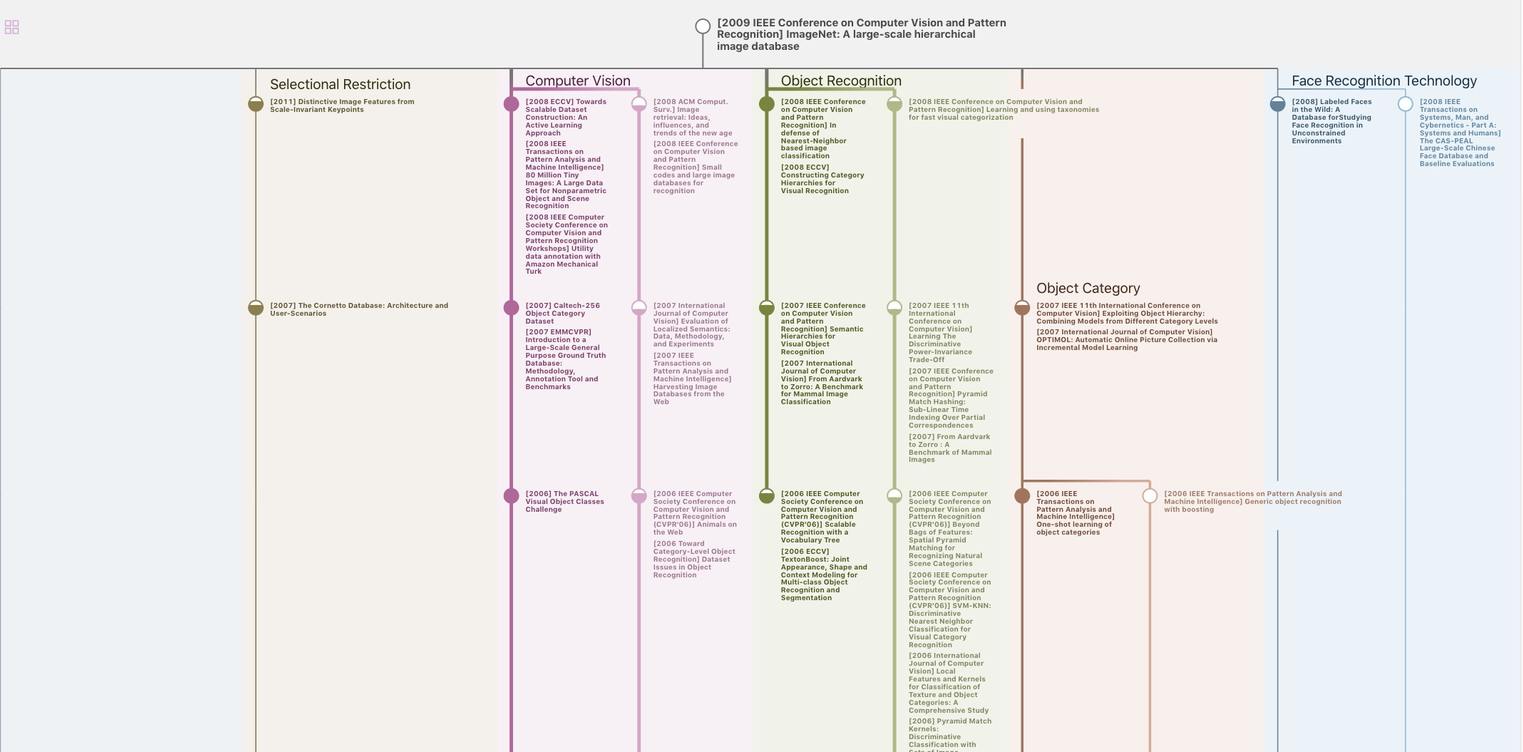
生成溯源树,研究论文发展脉络
Chat Paper
正在生成论文摘要