Search-based Recommendation: the Case for Difficult Predictions
COMPANION OF THE WORLD WIDE WEB CONFERENCE, WWW 2023(2023)
摘要
Recommender systems have achieved impressive results on benchmark datasets. However, the numbers are often influenced by assumptions made on the data and evaluation mode. This work questions and revises these assumptions, to study and improve the quality, particularly for the difficult case of search-based recommendations. Users start with a personally liked item as a query and look for similar items that match their tastes. User satisfaction requires discovering truly unknown items: new authors of books rather than merely more books of known writers. We propose a unified system architecture that combines interaction-based and content-based signals and leverages language models for Transformer-powered predictions. We present new techniques for selecting negative training samples, and investigate their performance in the underexplored search-based evaluation mode.
更多查看译文
关键词
recommender system,language model,transformer,search-based prediction,negative sampling
AI 理解论文
溯源树
样例
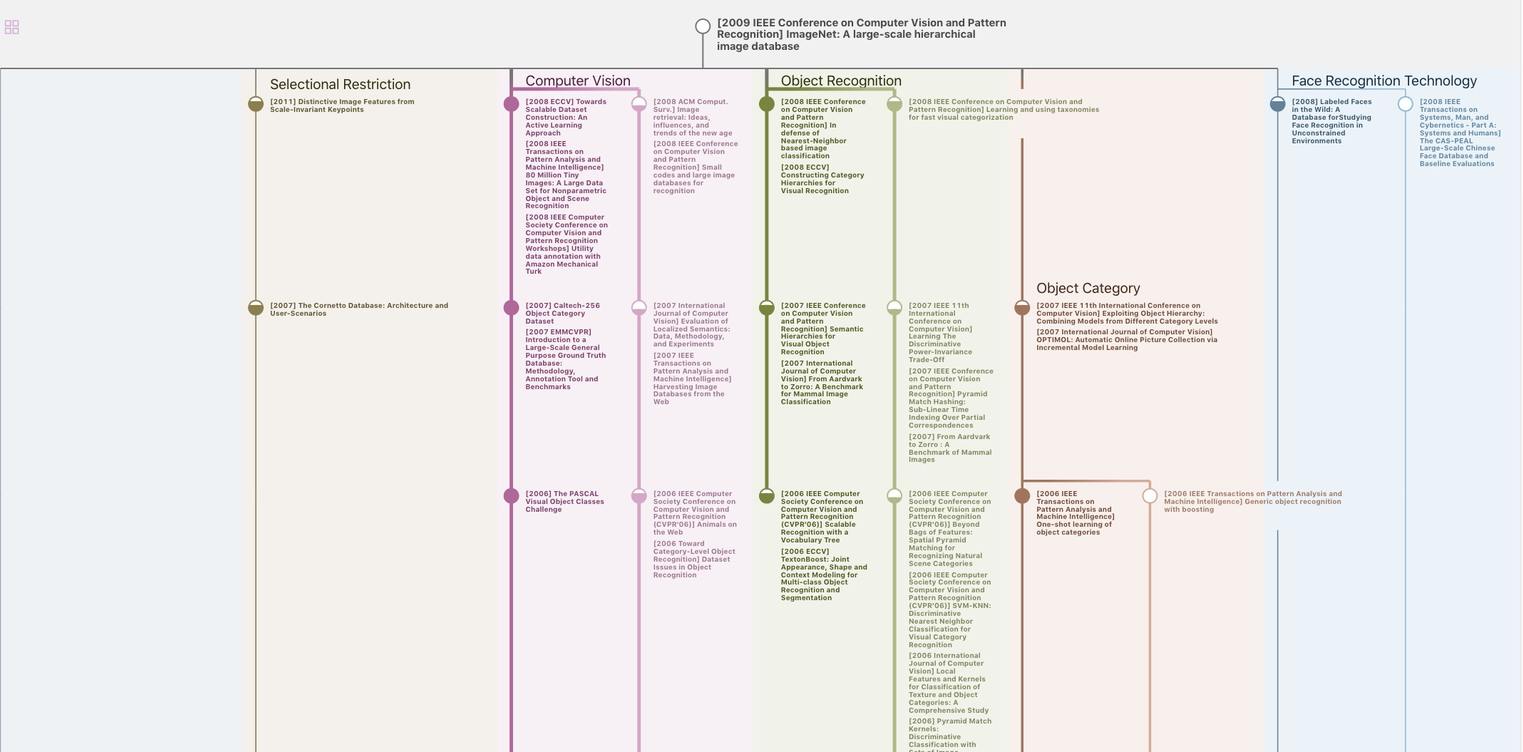
生成溯源树,研究论文发展脉络
Chat Paper
正在生成论文摘要