Self-Preserving Genetic Algorithms vs. Safe Reinforcement Learning in Discrete Action Spaces
PROCEEDINGS OF THE 2023 ACM/IEEE 14TH INTERNATIONAL CONFERENCE ON CYBER-PHYSICAL SYSTEMS, WITH CPS-IOTWEEK 2023(2023)
摘要
Safe learning techniques are learning frameworks that take safety into consideration during the training process. Safe reinforcement learning (SRL) combines reinforcement learning (RL) with safety mechanisms such as action masking and run time assurance to protect an agent during the exploration of its environment. This protection, though, can severely hinder an agent's ability to learn optimal policies as the safety systems exacerbate an already difficult exploration challenge for RL agents. An alternative to RL is an optimization approach known as genetic algorithms (GA), which utilize operators that mimic biological evolution to evolve better policies. By combining safety mechanisms with genetic algorithms, this work demonstrates a novel approach to safe learning called Self-Preserving Genetic Algorithms. To highlight the training benefits of SPGA compared to SRL in discrete action spaces, this demonstration trains and deploys an SPGA agent with action masking (SPGA-AM) and an SRL agent with action masking (SRL-AM) in real-time in the CartPole-v0 environment with a safety boundary condition b = 0.75. After training, each of the learned policies are tested in a CartPole-v0 environment with an extended max timesteps value (T = 200 -> T = 1000). After the demo, users will have a better understanding of SPGA and SRL training, as well as the benefits of using SPGA to train in discrete action spaces.
更多查看译文
关键词
genetic algorithms,safe learning,safe reinforcement learning,run time assurance,action masking
AI 理解论文
溯源树
样例
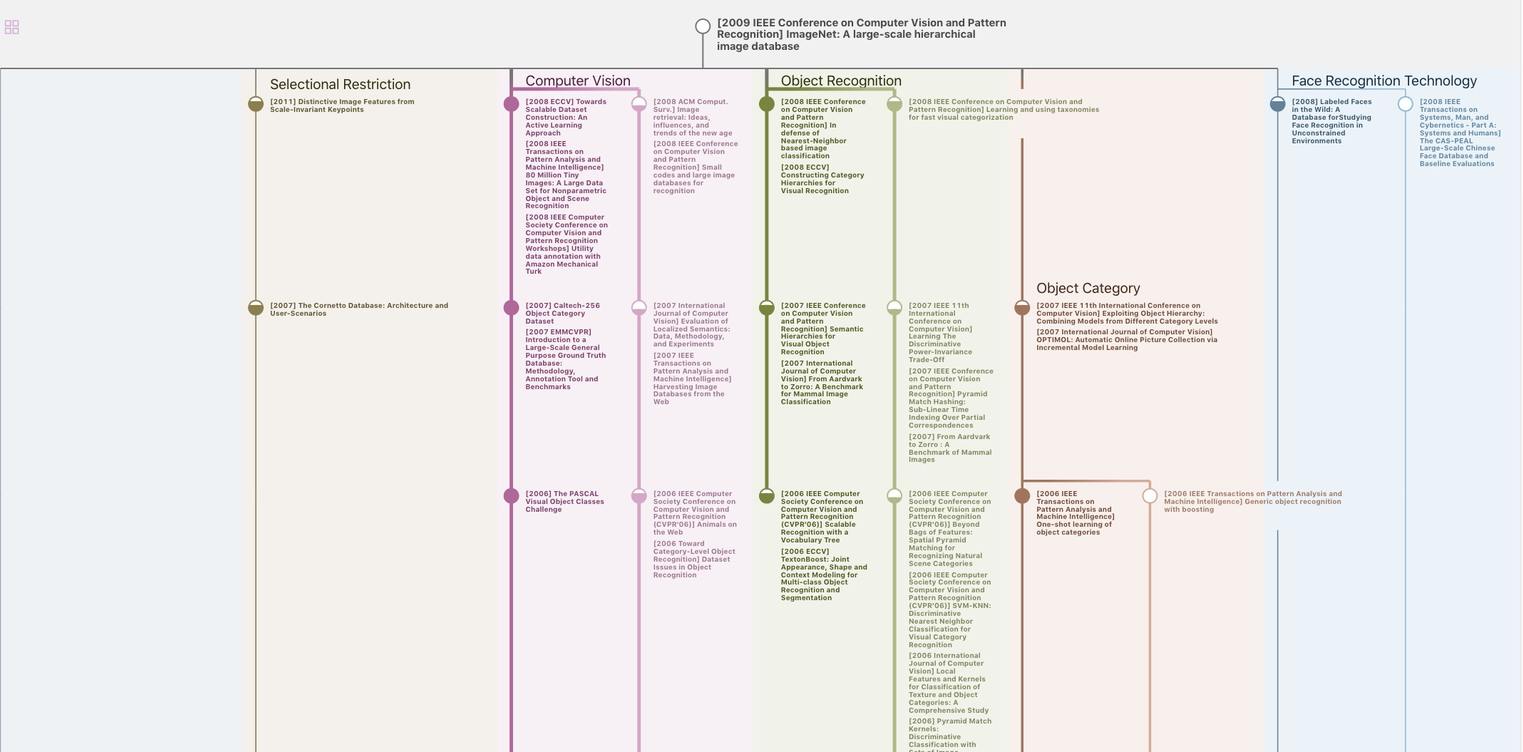
生成溯源树,研究论文发展脉络
Chat Paper
正在生成论文摘要