From Gram to Attention Matrices: a Monotonicity Constrained Method for Eeg-Based Emotion Classification
Applied Intelligence(2023)
关键词
Monotonicity constrained attention,EEG,Emotion classification,Deep learning,Parameter efficient model
AI 理解论文
溯源树
样例
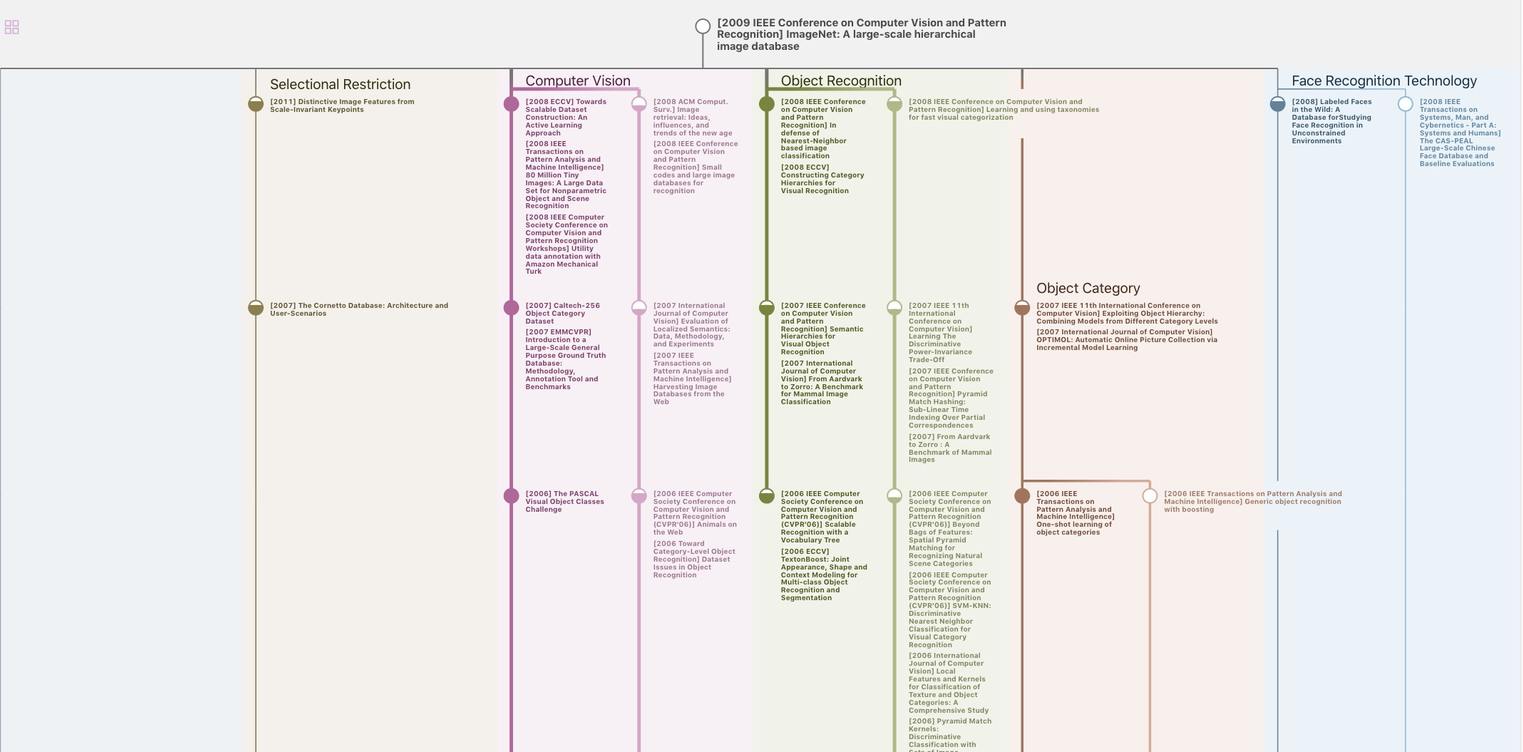
生成溯源树,研究论文发展脉络
Chat Paper
正在生成论文摘要