HAPENS: Hardness-Personalized Negative Sampling for Implicit Collaborative Filtering
COMPANION OF THE WORLD WIDE WEB CONFERENCE, WWW 2023(2023)
摘要
For training implicit collaborative filtering (ICF) models, hard negative sampling (HNS) has become a state-of-the-art solution for obtaining negative signals from massive uninteracted items. However, selecting appropriate hardness levels for personalized recommendations remains a fundamental, yet underexplored, problem. Previous HNS works have primarily adjusted the hardness level by tuning a single hyperparameter. However, applying the same hardness level to each user is unsuitable due to varying user behavioral characteristics, the quantity and quality of user records, and different consistencies of models' inductive biases. Moreover, increasing the number of hyperparameters is not practical due to the massive number of users. To address this important and challenging problem, we propose a model-agnostic and practical approach called hardness-personalized negative sampling (HAPENS). HAPENS uses a two-stage approach: in stage one, it trains the ICF model with a customized objective function that optimizes its worst performance on each user's interacted item set. In stage two, it utilizes these worst performances as personalized hardness levels with a well-designed sampling distribution, and trains the final model with the same architecture. We evaluated HAPENS on the collected Bing advertising dataset and one public dataset, and the comprehensive experimental results demonstrate its robustness and superiority. Moreover, HAPENS has delivered significant benefits to the Bing advertising system. To the best of our knowledge, we are the first to study this important and challenging problem.
更多查看译文
关键词
Personalization,Collaborative Filtering,Negative Sampling
AI 理解论文
溯源树
样例
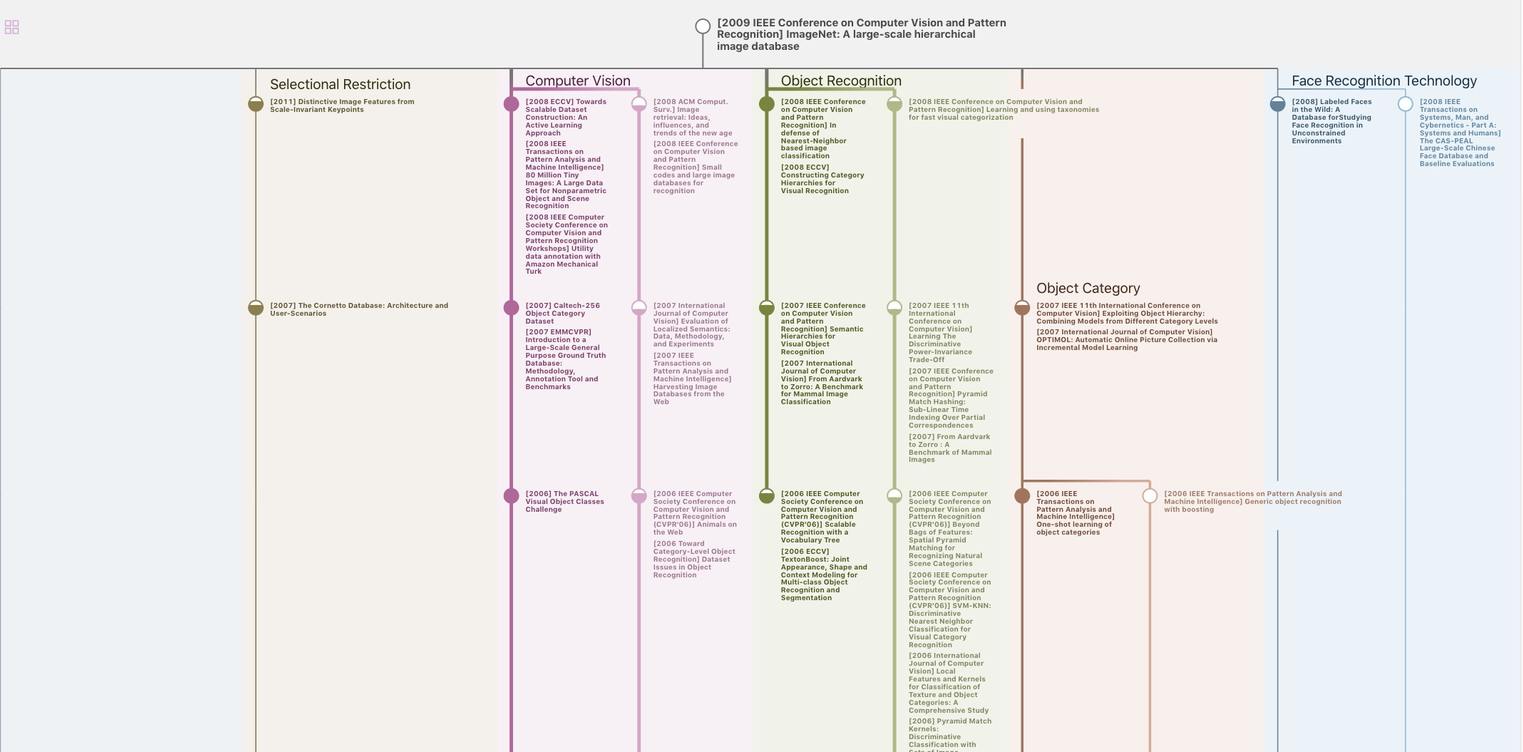
生成溯源树,研究论文发展脉络
Chat Paper
正在生成论文摘要