How to Pipeline Frame Transfer and Server Inference in Edge-assisted AR to Optimize AR Task Accuracy?
PROCEEDINGS OF THE 6TH INTERNATIONAL WORKSHOP ON EDGE SYSTEMS, ANALYTICS AND NETWORKING, EDGESYS 2023(2023)
摘要
Edge-assisted AR supports high-quality AR on resource-constrained mobile devices by offloading high-rate camera-captured frames to powerful GPU edge servers to perform heavy vision tasks. Since the result of an offloaded frame may not come back in the same frame interval, edge-assisted AR designs resort to local tracking on the last server returned result to generate more accurate result for the current frame. In such an offloading+local tracking paradigm, reducing the staleness of the last server returned result is critical to improving AR task accuracy. In this paper, we present MPCP, an online offloading scheduling framework that minimizes the staleness of server-returned result in edge-assisted AR by optimally pipelining network transfer of frames to the edge server and the Deep Neural Network inference on the edge server. MPCP is based on model predictive control (MPC). Our evaluation results show that MPCP reduces the depth estimation error by up to 10.0% compared to several baseline schemes.
更多查看译文
关键词
Edge-assisted Augmented Reality,Deep Neural Network,Edge Computing
AI 理解论文
溯源树
样例
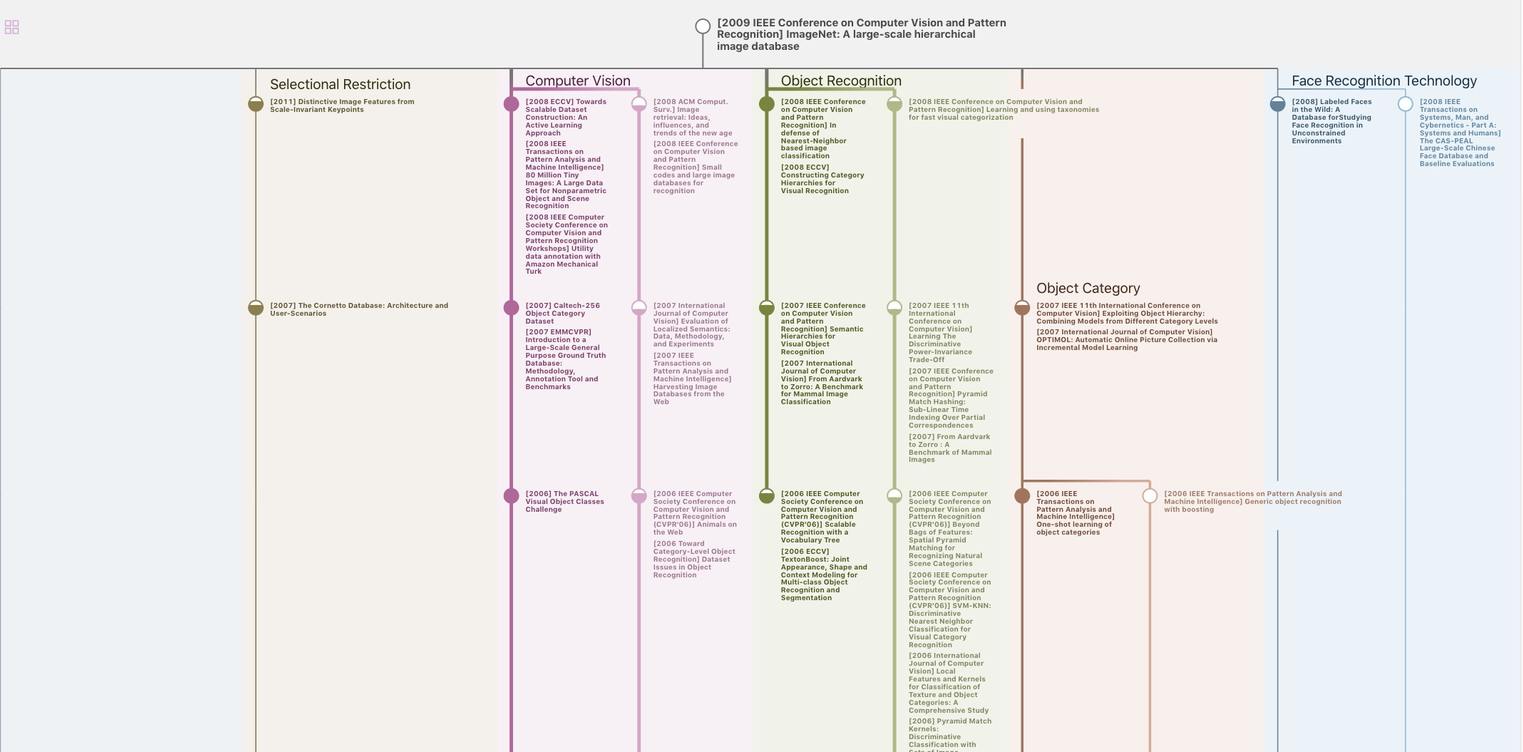
生成溯源树,研究论文发展脉络
Chat Paper
正在生成论文摘要