ALT: Breaking the Wall between Data Layout and Loop Optimizations for Deep Learning Compilation
EuroSys '23: Proceedings of the Eighteenth European Conference on Computer Systems(2023)
摘要
Deep learning models rely on highly optimized tensor libraries for efficient inference on heterogeneous hardware. Current deep compilers typically predetermine layouts of tensors and then optimize loops of operators. However, such unidirectional and one-off workflow strictly separates graph-level optimization and operator-level optimization into different system layers, missing opportunities for unified tuning. This paper proposes ALT, a deep compiler that performs joint graph-level layout optimization and operator-level loop optimization. ALT provides a generic transformation module to manipulate layouts and loops with easy-to-use primitive functions. ALT further integrates an auto-tuning module that jointly optimizes graph-level data layouts and operator-level loops while guaranteeing efficiency. Experimental results show that ALT significantly outperforms state-of-the-art compilers (e.g., Ansor) in terms of both single operator performance (e.g., 1.5x speedup on average) and end-to-end inference performance (e.g., 1.4x speedup on average).
更多查看译文
关键词
compiler techniques and optimizations,code generation and synthesis,deep learning systems
AI 理解论文
溯源树
样例
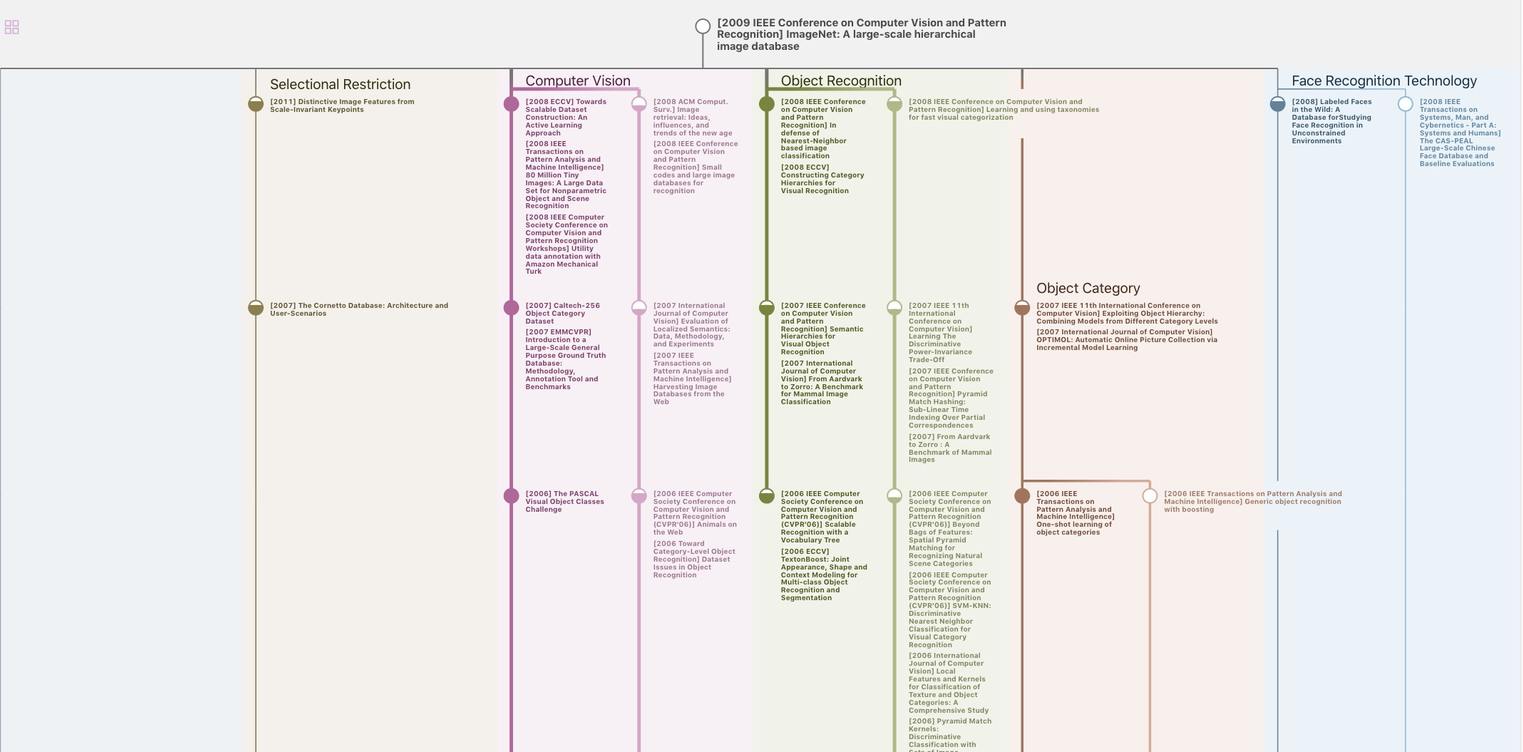
生成溯源树,研究论文发展脉络
Chat Paper
正在生成论文摘要