FedAR plus : A Federated Learning Approach to Appliance Recognition with Mislabeled Data in Residential Environments
ICCPS '23: Proceedings of the ACM/IEEE 14th International Conference on Cyber-Physical Systems (with CPS-IoT Week 2023)(2023)
摘要
With the enhancement of people's living standards and the rapid evolution of cyber-physical systems, residential environments are becoming smart and well-connected, causing a significant raise in overall energy consumption. As household appliances are major energy consumers, their accurate recognition becomes crucial to avoid unattended usage and minimize peak-time load on the smart grids, thereby conserving energy and making smart environments more sustainable. Traditionally, an appliance recognition model is trained at a central server (service provider) by collecting electricity consumption data via smart plugs from the clients (consumers), causing a privacy breach. Besides that, the data are susceptible to noisy labels that may appear when an appliance gets connected to a non-designated smart plug. While addressing these issues jointly, we propose a novel federated learning approach to appliance recognition, called FedAR+, enabling decentralized model training across clients in a privacy-preserving way even with mislabeled training data. FedAR+ introduces an adaptive noise handling method, essentially a joint loss function incorporating weights and label distribution, to empower the appliance recognition model against noisy labels. By deploying smart plugs in an apartment complex, we collect a labeled dataset that, along with two existing datasets, are utilized to evaluate the performance of FedAR+. Experimental results show that our approach can effectively handle up to 30% concentration of noisy labels while outperforming the prior solutions by a large margin on accuracy.
更多查看译文
关键词
Appliance recognition,federated learning,noisy labels,smart plug
AI 理解论文
溯源树
样例
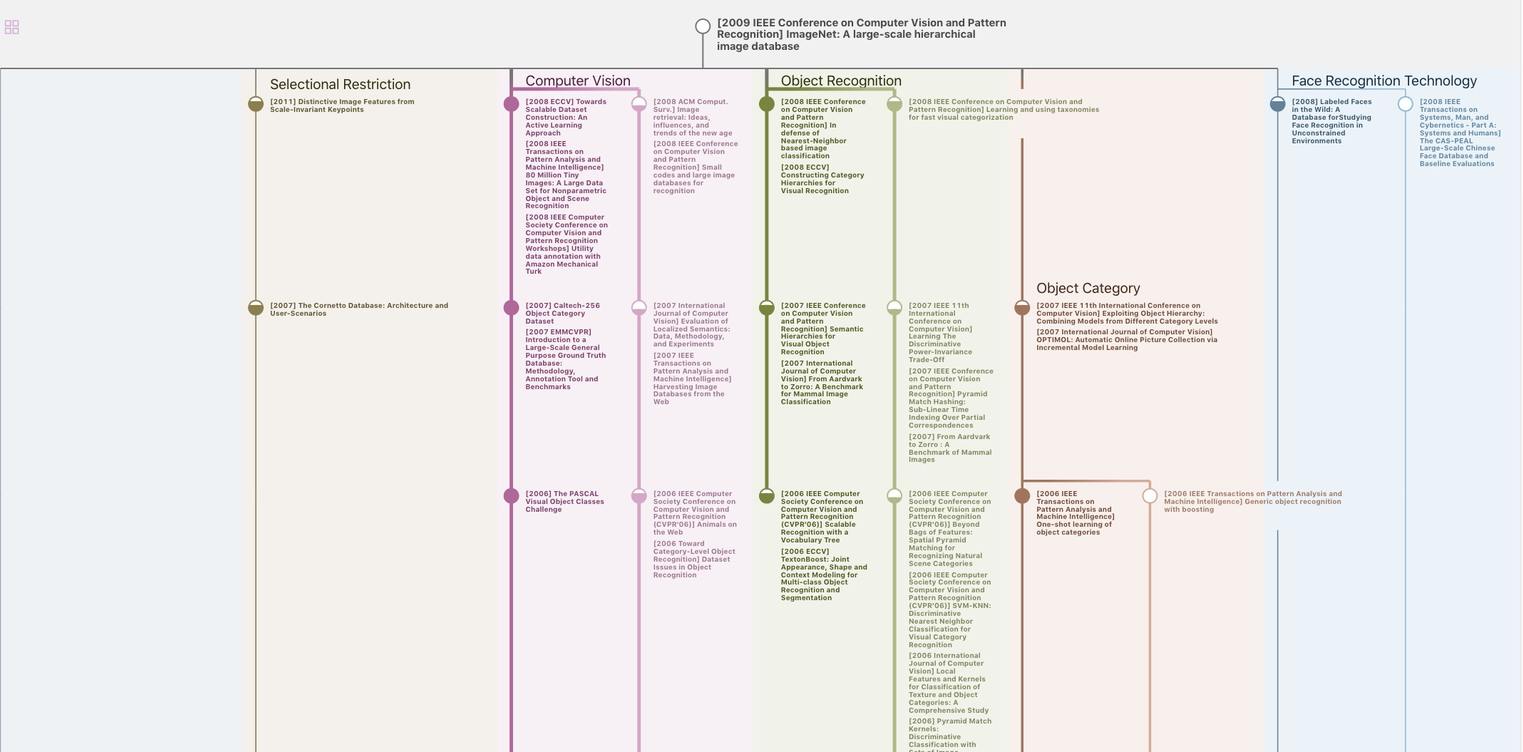
生成溯源树,研究论文发展脉络
Chat Paper
正在生成论文摘要