Black-box statistical prediction of lossy compression ratios for scientific data
CoRR(2023)
摘要
Lossy compressors are increasingly adopted in scientific research, tackling volumes of data from experiments or parallel numerical simulations and facilitating data storage and movement. In contrast with the notion of entropy in lossless compression, no theoretical or data-based quantification of lossy compressibility exists for scientific data. Users rely on trial and error to assess lossy compression performance. As a strong data-driven effort toward quantifying lossy compressibility of scientific datasets, we provide a statistical framework to predict compression ratios of lossy compressors. Our method is a two-step framework where (i) compressor-agnostic predictors are computed and (ii) statistical prediction models relying on these predictors are trained on observed compression ratios. Proposed predictors exploit spatial correlations and notions of entropy and lossyness via the quantized entropy. We study 8+ compressors on 6 scientific datasets and achieve a median percentage prediction error less than 12%, which is substantially smaller than that of other methods while achieving at least a 8.8x speedup for searching for a specific compression ratio and 7.8x speedup for determining the best compressor out of a collection.
更多查看译文
关键词
Scientific data,data reduction,lossy compression,high-performance applications,data storage and movements
AI 理解论文
溯源树
样例
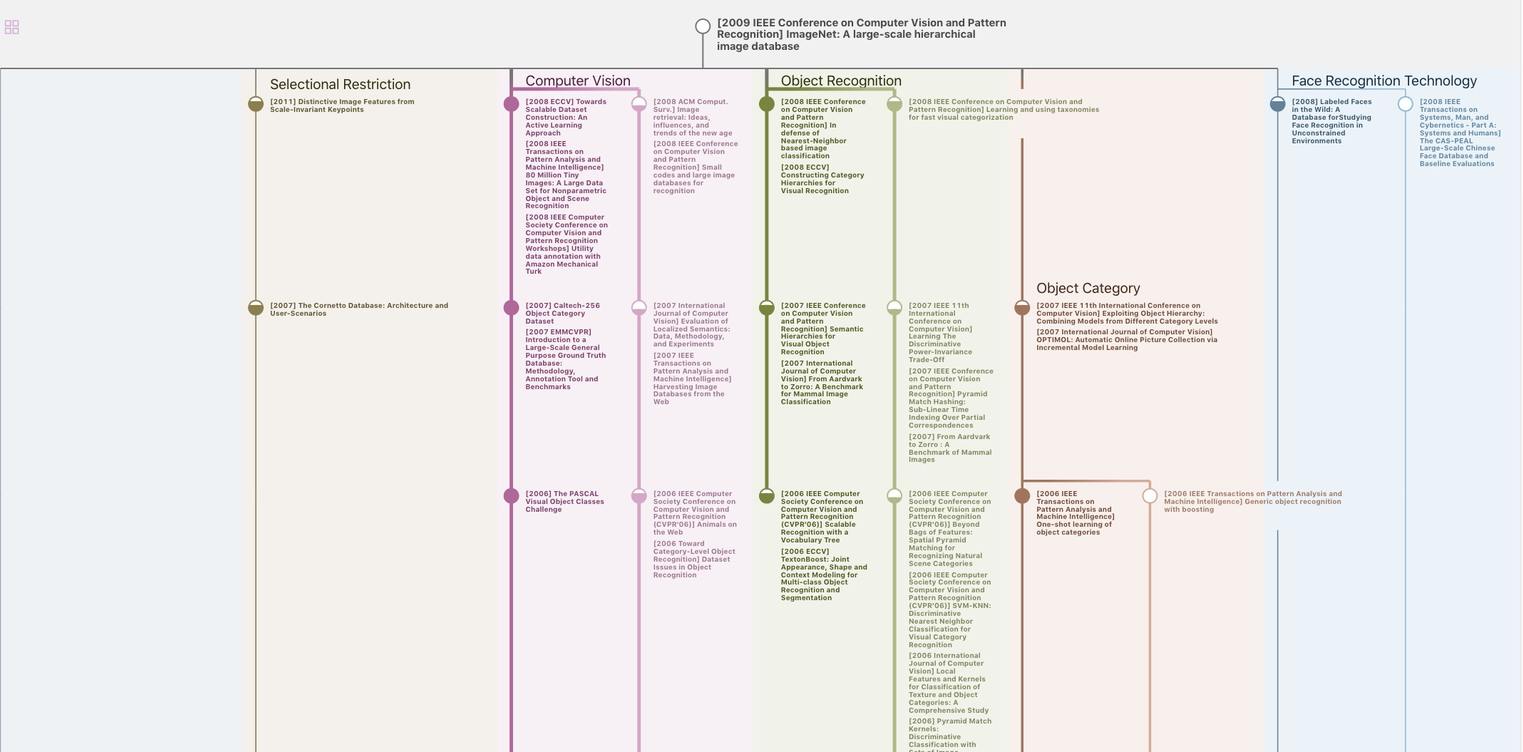
生成溯源树,研究论文发展脉络
Chat Paper
正在生成论文摘要