Approximation and Progressive Display of Multiverse Analyses
CoRR(2023)
摘要
A multiverse analysis evaluates all combinations of "reasonable" analytic decisions to promote robustness and transparency, but can lead to a combinatorial explosion of analyses to compute. Long delays before assessing results prevent users from diagnosing errors and iterating early. We contribute (1) approximation algorithms for estimating multiverse sensitivity and (2) monitoring visualizations for assessing progress and controlling execution on the fly. We evaluate how quickly three sampling-based algorithms converge to accurately rank sensitive decisions in both synthetic and real multiverse analyses. Compared to uniform random sampling, round robin and sketching approaches are 2 times faster in the best case, while on average estimating sensitivity accurately using 20% of the full multiverse. To enable analysts to stop early to fix errors or decide when results are "good enough" to move forward, we visualize both effect size and decision sensitivity estimates with confidence intervals, and surface potential issues including runtime warnings and model quality metrics.
更多查看译文
关键词
multiverse analyses,approximation,progressive display
AI 理解论文
溯源树
样例
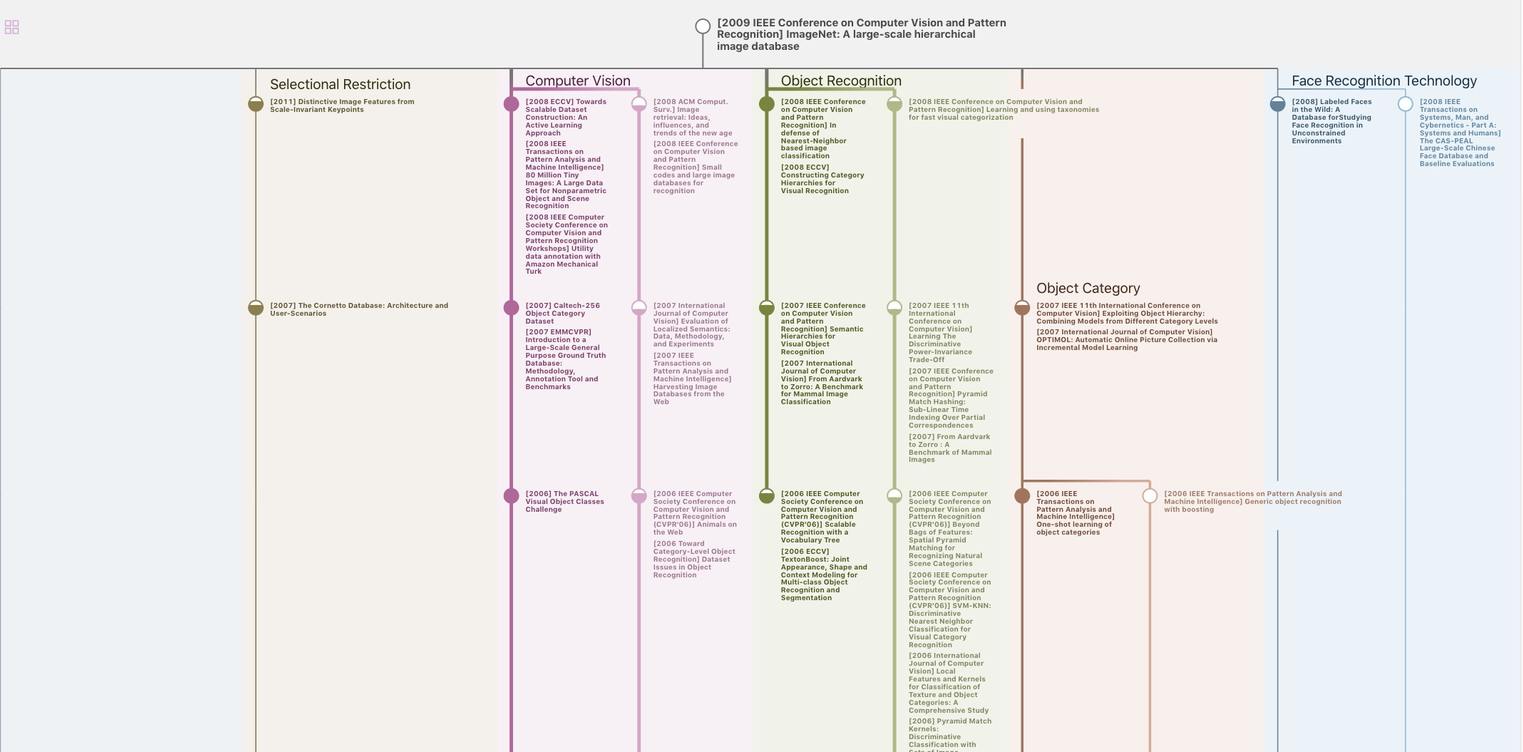
生成溯源树,研究论文发展脉络
Chat Paper
正在生成论文摘要