Learning Non-linguistic Skills without Sacrificing Linguistic Proficiency
conf_acl(2023)
摘要
The field of Math-NLP has witnessed significant growth in recent years, motivated by the desire to expand LLM performance to the learning of non-linguistic notions (numerals, and subsequently, arithmetic reasoning). However, non-linguistic skill injection typically comes at a cost for LLMs: it leads to catastrophic forgetting of core linguistic skills, a consequence that often remains unaddressed in the literature. As Math-NLP has been able to create LLMs that can closely approximate the mathematical skills of a grade-schooler or the arithmetic reasoning skills of a calculator, the practicality of these models fail if they concomitantly shed their linguistic capabilities. In this work, we take a closer look into the phenomena of catastrophic forgetting as it pertains to LLMs and subsequently offer a novel framework for non-linguistic skill injection for LLMs based on information theoretic interventions and skill-specific losses that enable the learning of strict arithmetic reasoning. Our model outperforms the state-of-the-art both on injected non-linguistic skills and on linguistic knowledge retention, and does so with a fraction of the non-linguistic training data (1/4) and zero additional synthetic linguistic training data.
更多查看译文
关键词
learning,proficiency,skills,non-linguistic
AI 理解论文
溯源树
样例
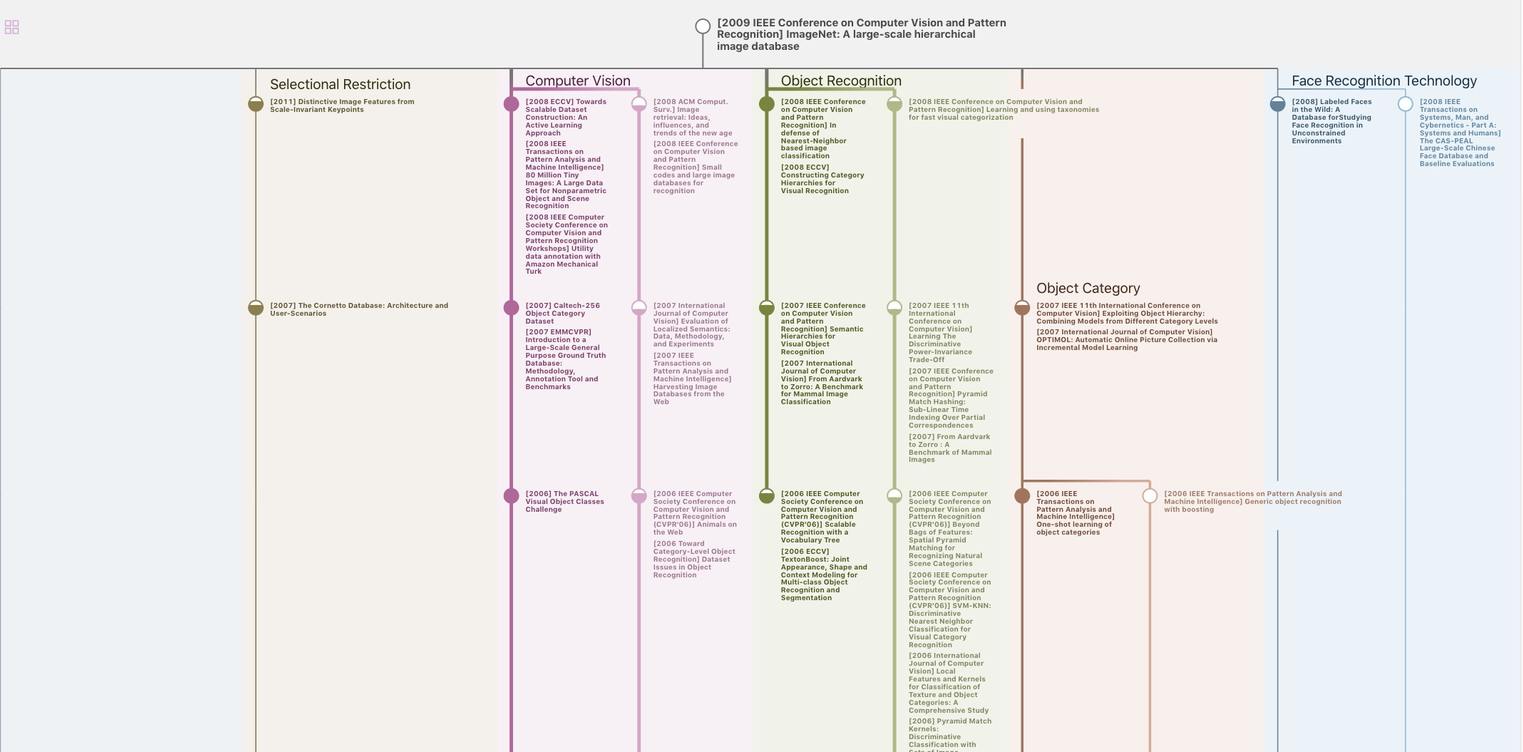
生成溯源树,研究论文发展脉络
Chat Paper
正在生成论文摘要