Can Learning Deteriorate Control? Analyzing Computational Delays in Gaussian Process-Based Event-Triggered Online Learning
CoRR(2023)
摘要
When the dynamics of systems are unknown, supervised machine learning techniques are commonly employed to infer models from data. Gaussian process (GP) regression is a particularly popular learning method for this purpose due to the existence of prediction error bounds. Moreover, GP models can be efficiently updated online, such that event-triggered online learning strategies can be pursued to ensure specified tracking accuracies. However, existing trigger conditions must be able to be evaluated at arbitrary times, which cannot be achieved in practice due to non-negligible computation times. Therefore, we first derive a delay-aware tracking error bound, which reveals an accuracy-delay trade-off. Based on this result, we propose a novel event trigger for GP-based online learning with computational delays, which we show to offer advantages over offline trained GP models for sufficiently small computation times. Finally, we demonstrate the effectiveness of the proposed event trigger for online learning in simulations.
更多查看译文
关键词
online learning,computational delays,deteriorate control,process-based,event-triggered
AI 理解论文
溯源树
样例
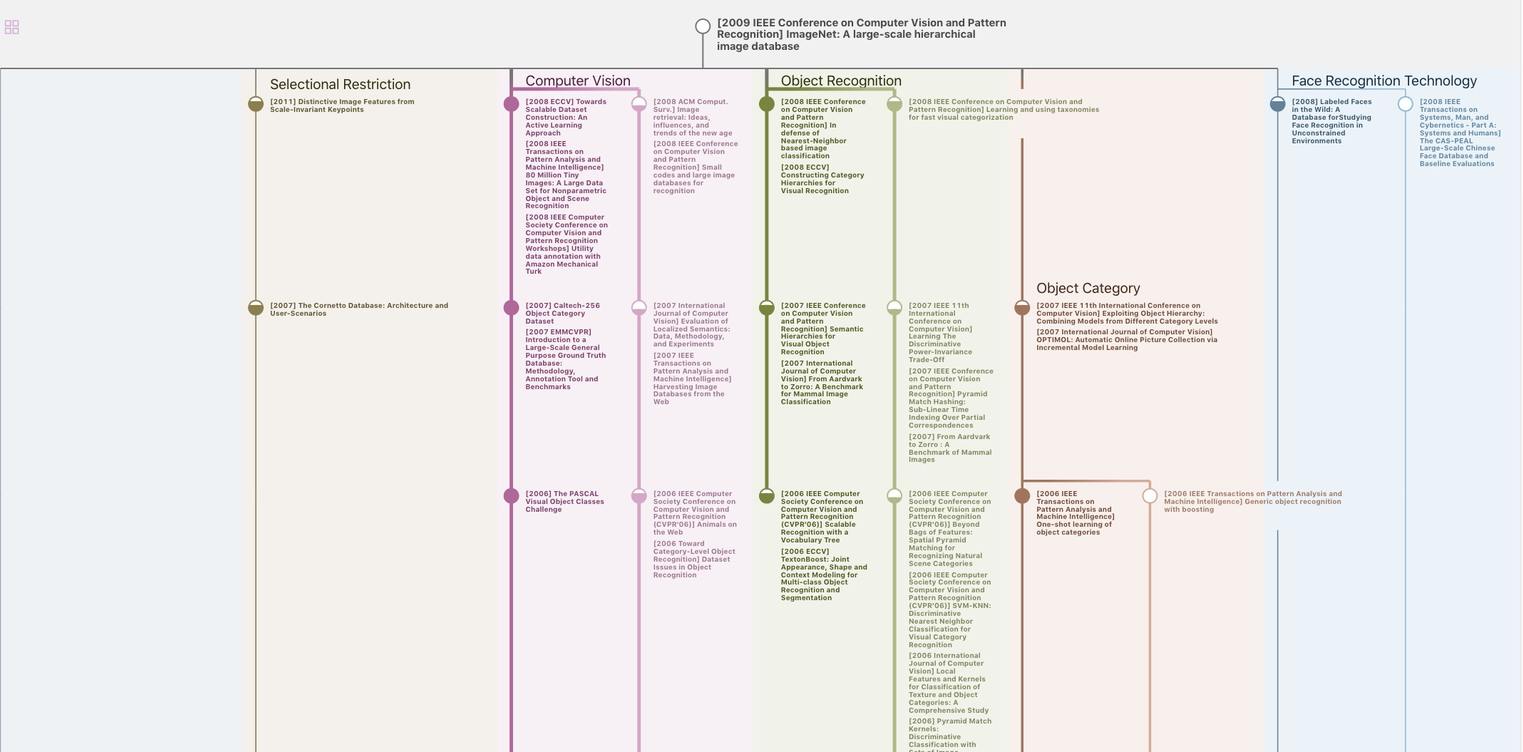
生成溯源树,研究论文发展脉络
Chat Paper
正在生成论文摘要