Monitoring and Adapting ML Models on Mobile Devices
CoRR(2023)
摘要
ML models are increasingly being pushed to mobile devices, for low-latency inference and offline operation. However, once the models are deployed, it is hard for ML operators to track their accuracy, which can degrade unpredictably (e.g., due to data drift). We design Nazar, the first end-to-end system for continuously monitoring and adapting models on mobile devices without requiring feedback from users. Our key observation is that often model degradation is due to a specific root cause, which may affect a large group of devices. Therefore, once Nazar detects a consistent degradation across a large number of devices, it employs a root cause analysis to determine the origin of the problem and applies a cause-specific adaptation. We evaluate Nazar on two computer vision datasets, and show it consistently boosts accuracy compared to existing approaches. On a dataset containing photos collected from driving cars, Nazar improves the accuracy on average by 15%.
更多查看译文
AI 理解论文
溯源树
样例
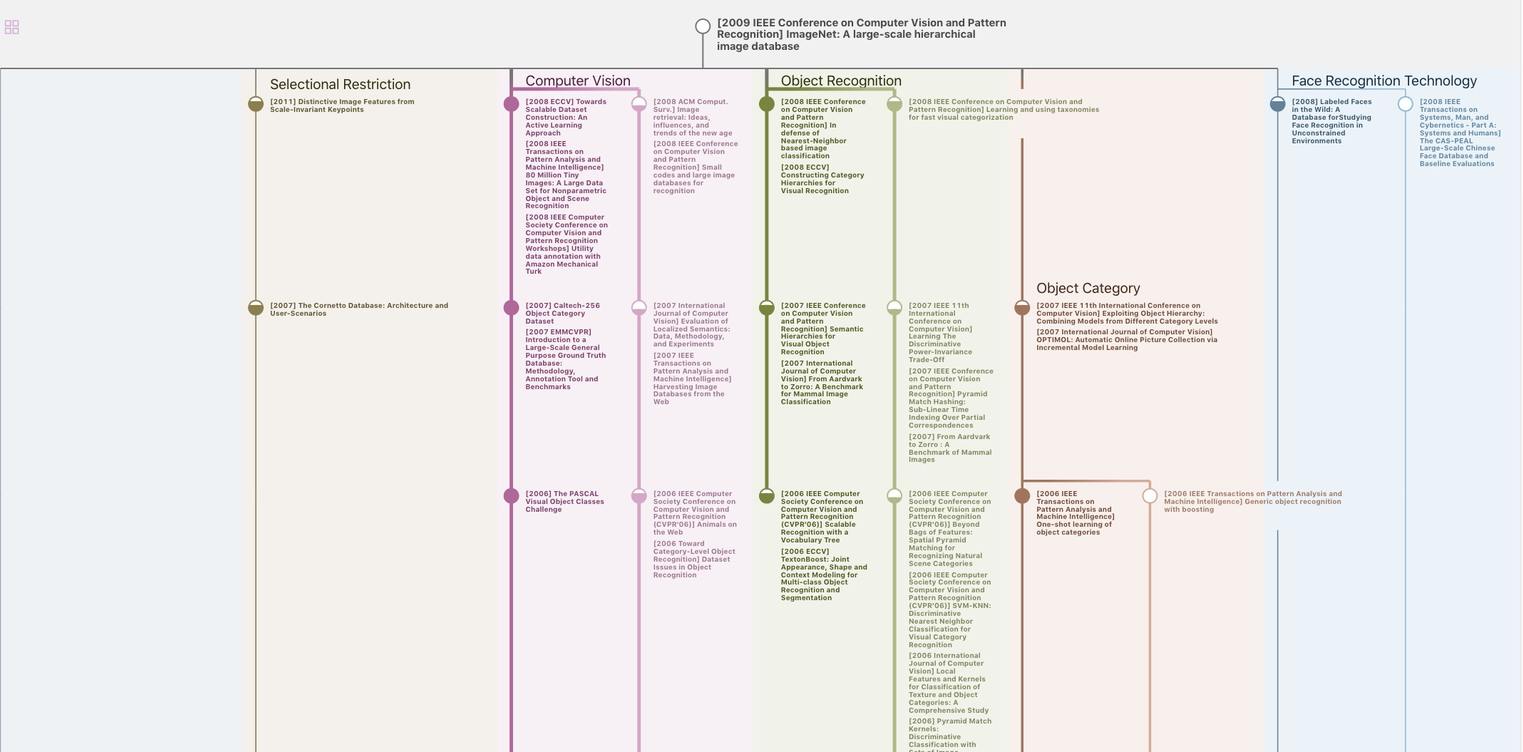
生成溯源树,研究论文发展脉络
Chat Paper
正在生成论文摘要