Using Gradient Boosting Algorithms in Predicting Student Academic Performance
2023 International Conference on Business Analytics for Technology and Security (ICBATS)(2023)
摘要
Educational institutes carried out a huge research to investigate student academic performance and analyses the major factors that may affect student performance. Educational institutes care about how many students will continue their studies successfully and their appreciation. There is a crucial need to investigate and address the main challenges that may affect the student academic performance. In this paper, we propose a new approach to analyses student academic performance using Gradient Boosting algorithms. In particular, the proposed approach implements the XGBoost, CaBoost and LighGBM Gradient Boosting algorithms. The proposed approach consists of two prediction models: the student final status model and student appreciation model. The achieved results show that the CaBoost algorithm gets the best prediction results when it reaches 92.16% for student final status model and 86.89% for student appreciation model. On the other hand, the LightGBM gets the best results in terms of speed.
更多查看译文
关键词
Student Academic Performance,Gradient Boosting algorithms,XGBoost,CatBoost,LightGBM,Appreciation,Final Status
AI 理解论文
溯源树
样例
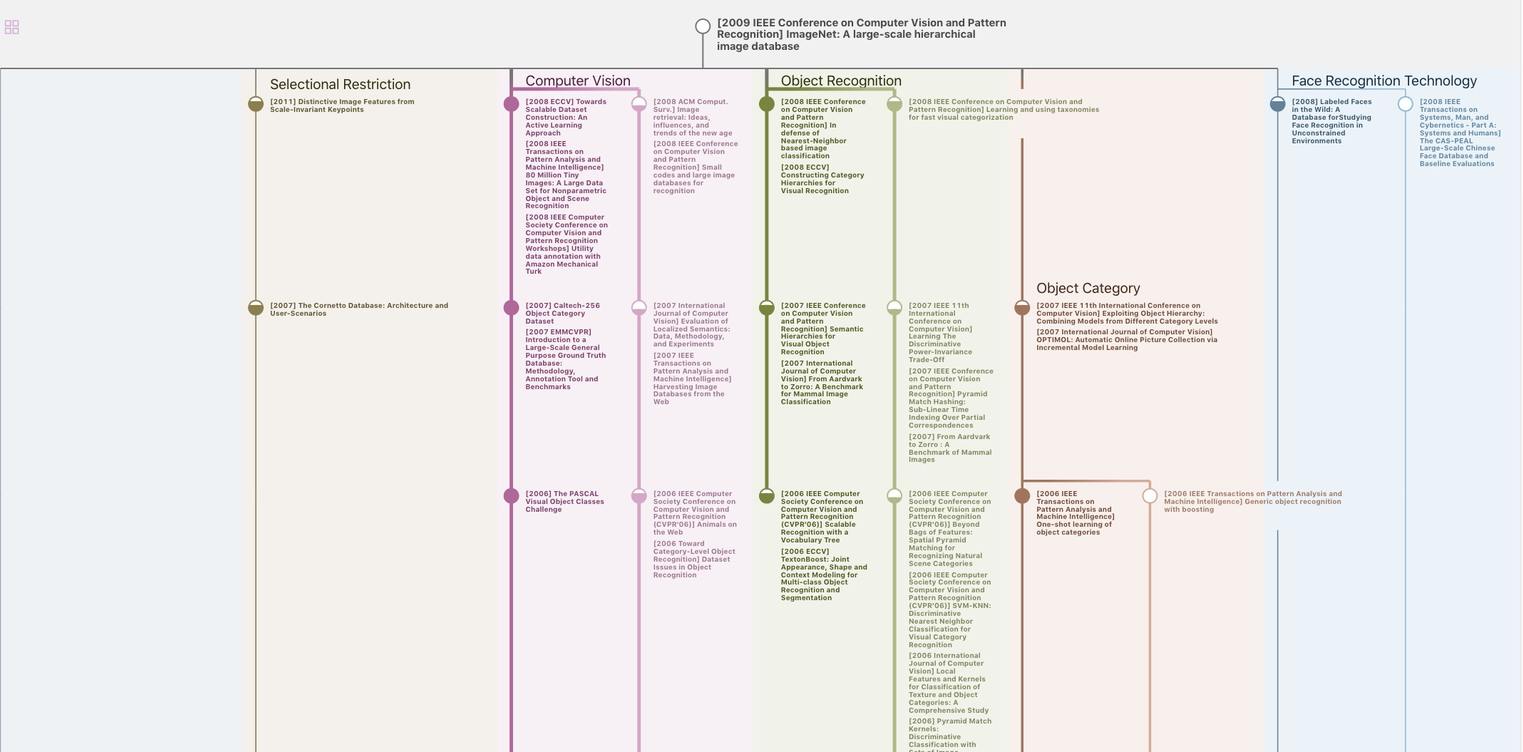
生成溯源树,研究论文发展脉络
Chat Paper
正在生成论文摘要