An extended TLD tracking algorithm using co-training learning for low frame rate videos
Multimedia Tools and Applications(2022)
摘要
The conventional tracking-learning-detection (TLD) algorithm is sensitive to illumination changes, clutter, significant changes of target shape between consecutive frames. In addition, low frame rate scenarios result in drift in object position or even missing the object. To solve these problems and enhance the tracking robustness, in this paper, TLD algorithm was extended in two folds. First, Kalman filter and Mean-shift algorithms were combined for the tracking part and second, co-training semi-supervised learning algorithm was used for the learning part of the conventional TLD structure. The Kalman filter estimates the position of the target in the next frame based on the previous positions of the target. This reduces tracking failure. On the other hands, the Mean-shift tracking algorithm is robust to rotation, partial occlusion and scale changing. In the learning part of TLD structure, two training tracking algorithms with two independent classifiers were run on the current frame simultaneously. Its structure makes data of both pools (color features and target templates) update by the results of other algorithm, in addition to the results of the corresponding algorithm in each of the tracking and detection algorithms. Therefore, classifiers can learn faster changing features of the target during the consecutive frames in online tracking process. Finally, the extended structure can solve the problem of lost object in LFR videos tracking and other similar challenges simultaneously. In terms of overlap ratio metric, comparing with conventional TLD and extended kernelized correlation filters (EKCF) algorithms, the success rate of our algorithm under various scenarios has increased by 161.03% and 255.82% respectively and under other scenarios, in terms of precision metric, it has increased by 18.46% and 1479.47%, respectively. Accordingly, comparative evaluations of the proposed method to other top state-of-the-art tracking algorithms under various scenarios present in TB-100 dataset, the superior performance of the proposed algorithm was demonstrated compared to other tracking algorithms in terms of tracking robustness, stability and real-time performance.
更多查看译文
关键词
Object tracking,Kalman filter,Mean-shift algorithm,Learning algorithm,Tracking learning detection
AI 理解论文
溯源树
样例
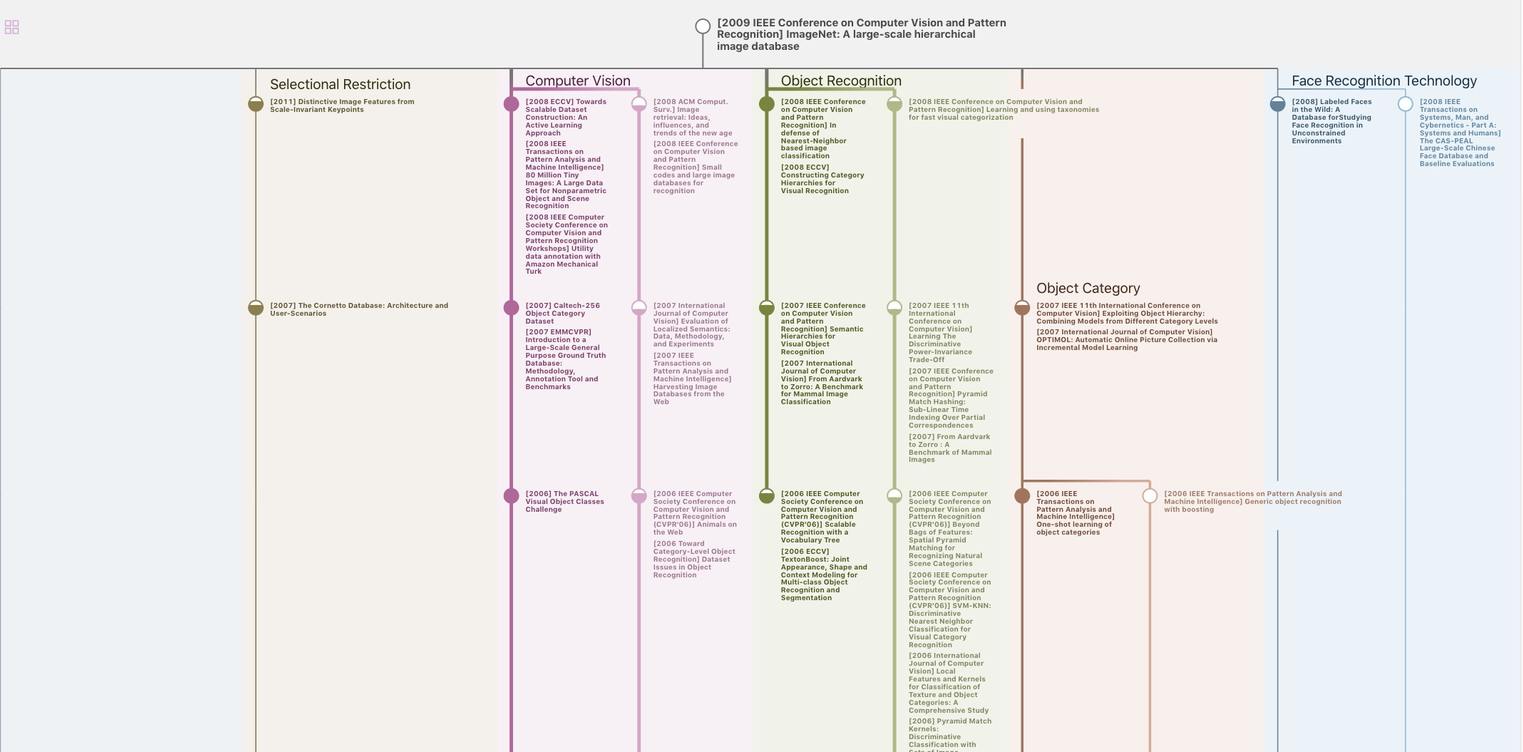
生成溯源树,研究论文发展脉络
Chat Paper
正在生成论文摘要